Choosing the Right Data Analysis Tool for Your Needs
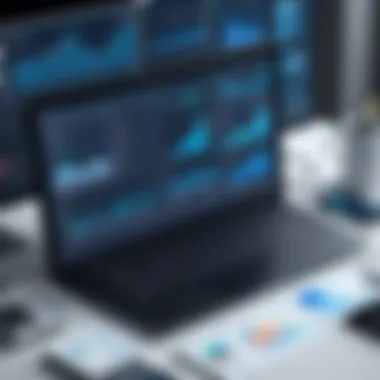
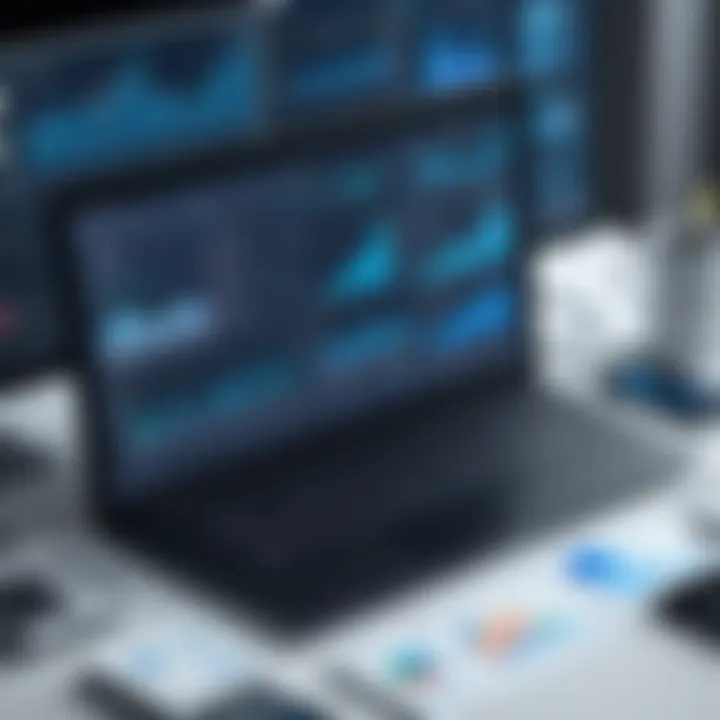
Intro
In the rapidly advancing world of data analytics, the selection of a tool has become a pivotal decision for organizations and individuals alike. As the saying goes, "a carpenter is only as good as his tools," which similarly holds true in the realm of data analysis. The right choices can not only drive efficiency but also enhance the quality of insights gleaned from data sets. With a plethora of options available, navigating this landscape can feel overwhelming. Therefore, understanding the core functionalities, user experiences, and industry applications is essential in determining the optimal tool.
This guide provides a deep dive into the features of prominent data analysis tools and how they cater to the varied needs of professionals across different fields. By outlining the key aspects that influence tool selection and shedding light on usability, we aim to empower decision-makers to make informed choices that resonate with their analytical goals.
Overview of Core Features
Description of essential functionalities
To sift through the myriad of options in the market, one must first understand the core features that define effective data analysis tools. Typically, the essential functionalities include:
- Data Integration: The ability to merge data from various sources is crucial. Good tools must handle structured, semi-structured, and unstructured data smoothly.
- Data Visualization: Visual representation assists in comprehending complex data. Tools should offer customizable dashboards and data charts.
- Statistical Analysis: The inclusion of advanced analytical capabilities helps users perform in-depth evaluations, providing clearer insights into data trends.
- Collaboration Features: In today’s work environment, collaboration is key. Tools should facilitate sharing and collective analysis among teams.
Comparison of features across top software options
When evaluating tools, comparing their features can unveil their relative strengths and weaknesses. For instance, let's explore a few prominent tools in the arena:
- Tableau: Renowned for its robust data visualization capabilities, making complex data sets digestible with striking graphics.
- Microsoft Power BI: Combines comprehensive data integration and user-friendly design, beneficial for those already in the Microsoft ecosystem.
- SAS Analytics: Offers deep statistical and predictive capabilities, ideal for industries requiring advanced analytics.
- R Programming: While free and highly flexible, R requires programming knowledge and is more suited for data scientists.
Each of these tools carries its unique offerings, and the ideal choice largely depends on the specific analytical requirements and user skill levels.
User Experience and Interface
Insights into UI/UX design aspects
The user interface plays a vital role in how effectively a tool can be utilized. A well-designed UI/UX simplifies the process of data analysis. It’s not just about aesthetics but also functionality. Tools that prioritize intuitive design often enhance user engagement, minimizing the learning curve.
- Drag-and-drop features: Many top-tier tools allow users to easily visualize data with intuitive drag-and-drop capabilities, fostering swift data manipulation.
- Responsive layouts: As more users analyze data on multiple devices, responsive designs enable seamless transitions between platforms, from desktops to mobile.
Importance of usability and accessibility
Usability and accessibility are non-negotiable in today’s diverse working environment. Products that prioritize these aspects ensure all users, irrespective of skill level, can carry out their analytical tasks efficiently. This is especially relevant in industries where non-technical stakeholders require insights to make data-driven decisions. A tool must cater to a broad audience, allowing everyone to tap into the power of data analysis.
"The best data analysis tool isn’t necessarily the most powerful one; it’s the one that the user can navigate with ease and confidence."
In summary, the landscape of data analysis tools is rich with variety. By grasping what core features are necessary and understanding the importance of usability, decision-makers can significantly enhance their data analysis capabilities.
Foreword to Data Analysis Tools
In the modern business landscape, the ability to seamlessly interpret data transforms an overwhelming mass of numbers into actionable insights. As organizations grapple with the flood of information from various sources, choosing the right data analysis tool becomes paramount. It's not just about crunching numbers; it's about untangling stories hidden within the data and powering strategic decisions. The discussion of data analysis tools is essential because it sets the foundation for what follows in this article. Here, we'll focus on the key elements of utilizing these instruments, the myriad benefits they offer, and the considerable factors one must heed when selecting the finest option for their specific needs.
The Importance of Data Analysis
Data analysis serves as the backbone of informed decision-making. It's akin to having a map in a foggy journey—you might have a destination, but without visibility, you could easily go astray. Effectively processing data allows organizations to:
- Identify Trends: By examining data over time, stakeholders can detect patterns that may inform future actions.
- Enhance Efficiency: Analyzing processes can spotlight inefficiencies, allowing for targeted improvements.
- Support Risk Management: With thorough analysis, organizations can foresee potential challenges and develop mitigation strategies.
- Drive Innovation: Insights gained from data can lead to fresh ideas and approaches, keeping enterprises ahead of the competition.
Overall, data analysis is not merely an ancillary function but a vital component interwoven into the fabric of every successful organization.
Key Objectives of Data Analysis
When diving into data analysis, it’s crucial to outline the objectives clearly. These goals guide the analysis process, ensuring alignment with business strategies. The following points illustrate the primary aims:
- Decision-Making Support: A significant objective revolves around informing decision-makers with clear data-backed insights.
- Understanding Behavior: For industries like retail, analyzing consumer behavior is indispensable. It fosters tailored marketing strategies and product development that resonate with customers.
- Increase Competitiveness: Organizations utilize data to gauge market trends, thus positioning themselves strategically in their respective sectors.
- Resource Allocation: By analyzing performance metrics, firms can allocate resources more efficiently, ensuring optimum use.
In sum, establishing concrete objectives in data analysis not only streamlines the analysis process but also maximizes the impact of the data processed on overall business strategy.
"In data, we find the keys to unlock the door of understanding.
Choosing the optimal tool for analyzing data is akin to selecting the right instrument for a musician. It’s essential not only for personal preference but also for achieving the finer results required to produce harmony in any organization.
Criteria for Selecting Data Analysis Tools
Choosing the right data analysis tool is akin to selecting the right tool from a toolkit: the wrong choice can lead to frustration and inefficiency, while the right one can enhance your ability to uncover insights and drive decisions. As the realm of data analytics continues to evolve, the landscape of available tools becomes increasingly complex. Decision-makers need to navigate through a myriad of options, each boasting unique features and capabilities. Therefore, understanding specific criteria that influence tool selection becomes paramount. The efficacy of your data analysis largely hinges on your choice of tool, making this assessment crucial for achieving optimal results.
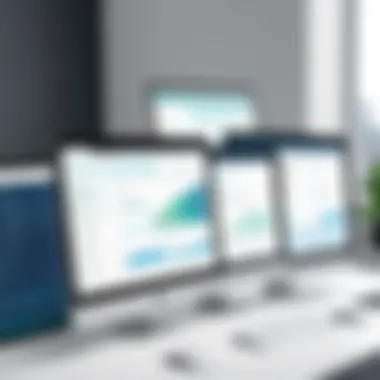
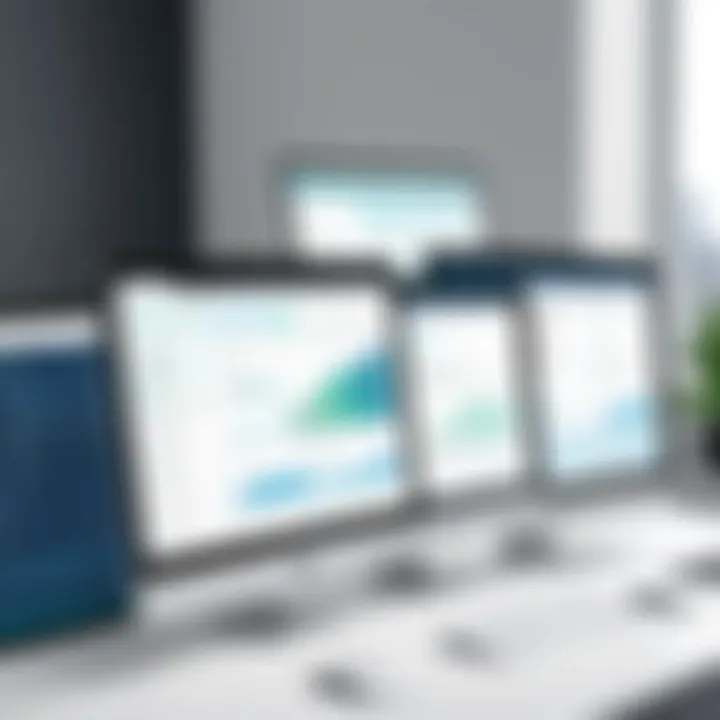
Usability and User Experience
The usability factor of a data analysis tool cannot be overstated. When the interface is intuitive, users of all skill levels can engage with the data effectively, reducing the learning curve significantly. Modern tools often offer a graphical user interface (GUI) that simplifies data manipulation. For instance, software like Microsoft Excel allows users to perform complex calculations using simple commands, while tools like Tableau offer drag-and-drop features for building visualizations. Ultimately, a positive user experience enhances productivity and facilitates more profound insights, as users can focus on analysis rather than struggling with the tool itself.
Integration Capabilities
In the age of diverse data sources, the integration capabilities of your chosen tool play a significant role. Organizations often utilize multiple systems to gather data—be it through CRM platforms, web analytics, or databases. A tool that seamlessly integrates with these systems allows for smoother data transfers and more cohesive analyses. For example, tools like R and Python offer libraries that enable easy integration with APIs, databases, and external data sources. Such capabilities minimize data silos and enhance the continuity of analysis, making it crucial for any organization aiming for holistic insights.
Cost Considerations
Cost is often a make-or-break factor when selecting a data analysis tool. Organizations need to weigh the initial purchasing price against long-term value, factoring in subscription fees, maintenance costs, and potential scaling expenses. Open-source platforms like R and Python provide powerful alternatives at little to no cost, but they may demand more in terms of employee skill set. Propriety options like SAS or Tableau come with a price tag, often promising robust support and advanced features, but may not be feasible for every budget. Finding the right balance between cost and functionality is vital to ensure sustainability.
Support and Resources
Support can make a world of difference in the performance of your data analysis tools. Assessing the availability of training materials, community forums, and direct customer support is crucial. Tools like Tableau and Power BI, for instance, offer extensive documentation and active communities that empower users to support one another. Furthermore, reliable vendor support can alleviate potential downtime and enhance workflow continuity. In such a technical domain, having a safety net can significantly ease the burden on businesses and encourage users to dive deeper into data exploration.
Overview of Popular Data Analysis Tools
In the ever-expanding realm of data analysis, understanding the vast array of tools available is crucial for professionals and organizations alike. This section sheds light on some of the most widely used software, each offering unique capabilities and strengths. Recognizing the right tool for the job can lead to enhanced insights, improved efficiency, and ultimately, better decision-making. Here, we will focus on a variety of popular tools, offering insights into their functionalities, strengths, and suitability for specific analytical needs.
Spreadsheet Software: Microsoft Excel
Microsoft Excel has long been a stalwart in data analysis. Its familiar interface and versatile features make it a go-to tool for many. Excel is particularly useful for basic data manipulation and visualization. Users can easily create tables, apply formulas, and generate charts. For many small businesses or individuals, Excel is often the first step into data analysis, facilitating budget tracking, simple statistical calculations, and presenting data in a clear, understandable way.
However, it is essential to recognize its limitations. As datasets grow larger and more complex, users may find themselves facing performance issues or struggling with advanced analytics. While Excel offers some functionalities like PivotTables and basic trend analysis, it might not be the best fit for sophisticated, large-scale data projects.
Statistical Analysis Software: R
R has earned its reputation as a powerful tool in the demographic of statisticians and data scientists. This open-source programming language excels in statistical computing and graphics. With thousands of packages developed by a vibrant community, R can handle myriad tasks, from data manipulation to complex modeling. Its capabilities in statistical analysis are unmatched, making it a favorite in academia and among researchers.
One significant advantage of R is its visualization capabilities. The ggplot2 package, for instance, allows users to create intricate and aesthetically pleasing graphics. However, R's steeper learning curve might deter some beginners. Familiarity with coding concepts is often necessary, which can pose a challenge for non-technical users. Still, for those who invest the time, R can unlock a world of analytical power.
Business Intelligence Tools: Tableau
Shifting gears, Tableau stands out as one of the leading business intelligence tools available today. Known for its user-friendly interface, Tableau simplifies the process of data visualization and reporting. Users can easily connect to various data sources, creating dashboards that communicate key insights at a glance.
Tableau's strengths lie in its interactivity and ability to handle large data sets seamlessly. Users can drill down into data as needed, fostering a more in-depth understanding. In an age where data storytelling is paramount, Tableau offers compelling visualizations that can engage stakeholders. However, its pricing model might be a consideration for smaller organizations looking to adopt robust BI solutions.
Big Data Technologies: Apache Hadoop
When it comes to handling massive amounts of data, Apache Hadoop is a name that's hard to ignore. This open-source framework allows for the distributed processing of large data sets across clusters of computers. It effectively stores and manages extensive volumes of data, enabling insights at a scale previously unattainable.
Moreover, Hadoop's flexibility in integrating with various data types—be it structured or unstructured—makes it a versatile choice for big data projects. However, it's essential to highlight that Hadoop requires a certain level of infrastructure and expertise. Organizations need to consider factors such as server setup and ongoing maintenance when adopting this technology.
Machine Learning Platforms: Python Libraries
In the realm of machine learning, Python libraries have gained immense traction. Libraries such as pandas, NumPy, and scikit-learn have empowered data practitioners to perform data analyses, model training, and predictions with unprecedented ease. Python’s syntax is often considered more intuitive compared to other programming languages, which lowers the barrier for entry for new users.
Furthermore, the community surrounding Python is expansive, providing a wealth of resources, tutorials, and forums for support. Its capability to integrate with other technologies and perform ETL (Extract, Transform, Load) tasks positions Python as a robust choice for data analysis.
However, while accessible, the powerful functionalities may require time to master fully, particularly for users aiming to leverage the more sophisticated aspects of machine learning.
"Choosing the right tool is fundamental for uncovering the hidden stories within data. Each software presents its own flavor of capabilities; hence, aligning these with your specific needs becomes paramount."
Comparing Functionality and Performance
When selecting a data analysis tool, one cannot overlook functionality and performance. In this tech-driven world, equipping oneself with a tool that can efficiently process, visualize, and report data is crucial for decision-makers, IT professionals, and entrepreneurs alike. The right selection not only boosts productivity but also influences the quality of insights derived.
Data Processing Capabilities
Data processing capabilities determine how well a tool can handle incoming data sets. This involves aspects such as speed, efficiency, and scalability of data operations. When dealing with increasingly large datasets, having a tool that can quickly clean, merge, or sort data is a top priority. For instance, tools like Microsoft Excel may facilitate quick analyses for smaller datasets but can bog down with extensive data operations. Conversely, platforms like Apache Hadoop are built for big data, enabling users to efficiently process terabytes of information without breaking a sweat.
In evaluating data processing capabilities, consider:
- Speed: How fast can the tool perform data operations?
- Scalability: Can it handle growing amounts of data?
- Flexibility: Is it adaptable for various data types and sources?
These factors play a significant role in determining not just the effectiveness of the analysis process but also the actionable insights that follow.
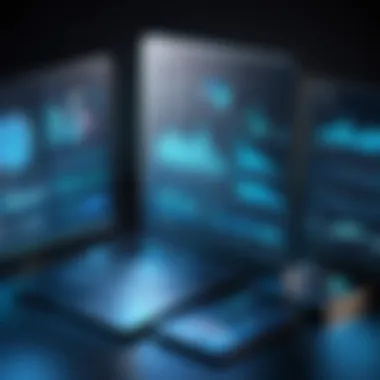
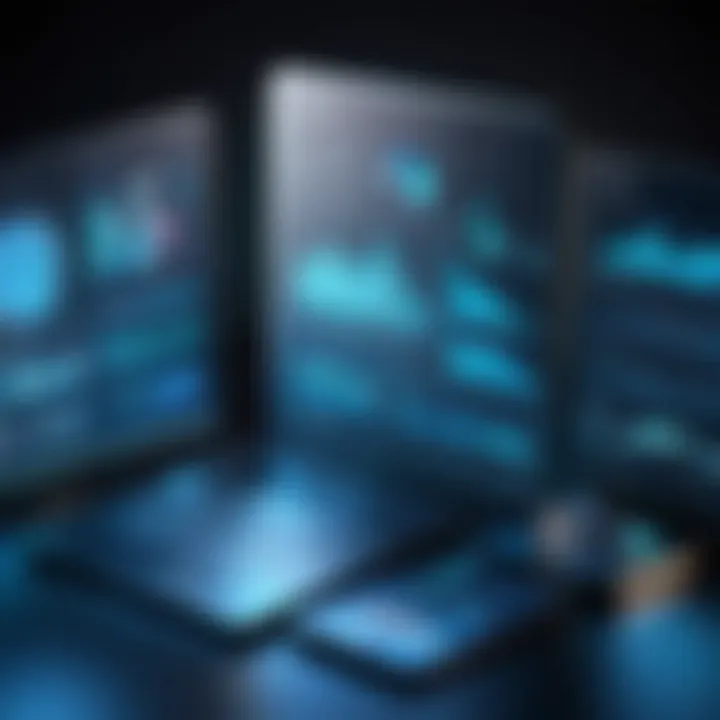
Visualization Features
Visualization features can make or break the data analysis experience. People often say, "A picture is worth a thousand words," and this couldn't be truer for data. Well-designed visualizations can highlight trends and anomalies that raw data might obscure. Tools like Tableau stand out because of their robust visual capabilities, allowing users to create dynamic dashboards and interactive stories out of their data. Meanwhile, other tools may offer limited visual options, making it challenging to convey findings compellingly.
Reporting and Dashboarding
Accurate reporting and intuitive dashboarding are the keystones of effective data analysis. In an ideal world, you would want a tool that not only generates reports with just a click but also allows customization to fit your audience's needs. Excellent dashboarding capabilities help in monitoring key performance indicators (KPIs) and providing a real-time snapshot of business health.
Consider evaluating:
- Report Customization: How much control do you have over report formats?
- Dashboard Interactivity: Can users interact with the data?
- Real-time Updates: Does the tool support real-time data reporting?
In the digital age, where decisions need to be data-driven, ensuring that your data analysis tool excels in functionality and performance is not just beneficial but essential.
When selecting your data analysis tool, keep these considerations in mind. A tool's ability to process data, visualize results, and generate customized reports can significantly influence business decisions. By combing through these functionalities carefully, you’re bound to find a tool tailored to your specific needs.
Industry-Specific Uses of Data Analysis Tools
In today's data-driven world, the relevance of industry-specific data analysis tools cannot be overstated. Each sector has unique demands and challenges, and data analysis tools need to align perfectly with these particularities. Knowing how to tailor your tool selection can make a significant difference. By applying data analysis in ways specific to each industry, organizations can better understand their market dynamics, improve operational efficiencies, and make informed decisions. This section will shed light on various industries, exploring the nuances that come into play regarding data analysis tools.
Data Analysis in Healthcare
Data analysis in healthcare is a monumental game changer. With the continuous influx of patient data, electronic health records, and research statistics, healthcare professionals need robust tools. One key benefit here involves improving patient outcomes through predictive analytics. For instance, hospitals can utilize data to forecast which patients might be at risk of readmission, allowing them to take proactive steps.
Consider this: the integration of statistical tools like R helps epidemiologists appraise outbreaks or manage public health initiatives more effectively.
- Efficient data management leads to better treatment strategies.
- Real-time analytics can spot anomalies in patient vitals swiftly.
Healthcare data analysis can assist in resource allocation too, ensuring that facilities are equipped for patient needs without overspending.
Financial Services and Risk Analysis
In the financial sector, risk management reigns supreme. Financial institutions leverage data analysis tools to parse through colossal datasets, aiming to predict trends and model scenarios. With tools like Python libraries focused on machine learning, analytics can pinpoint potential risks associated with investments.
"The ability to analyze financial risks with accuracy helps institutions maintain stability and comply with regulatory requirements."
- Predictive modeling assists in evaluating credit risk, determining which clients are likely to default.
- Fraud detection systems utilize algorithms to wade through transactions in real-time, flagging suspicious activity almost instantly.
It’s a race against time, and having the right tools means staying ahead of the curve in maintaining an institution's integrity.
Retail Analytics and Consumer Behavior
The retail landscape is all about understanding customer preference. Tools that analyze shopping patterns reveal what products are hot and what’s been gathering dust. Companies often use tools like Tableau to visualize sales trends and customer demographics.
By harnessing data analytics:
- Retailers can tailor marketing campaigns based on customer insights, leading to targeted promotions.
- Inventory management systems ensure that stock levels are optimized according to seasonal trends, preventing overstock issues.
Analyzing consumer behavior is not just about numbers; it’s about creating a more personalized shopping experience. Being in tune with customers gives retailers a competitive edge.
Manufacturing and Supply Chain Optimization
In manufacturing, efficiency is king. Data analysis tools help in refining supply chain dynamics, allowing companies to track production in real-time. The ability to scrutinize manufacturing processes can lead to substantial reductions in waste and increases in quality.
- Predictive maintenance powered by analytics helps identify when machines require service, thereby minimizing downtime.
- Suppliers’ performance can be assessed through metrics, making negotiations and relationship management more data-driven.
In sum, using data-driven insight can hugely influence profit margins and operational efficacy.
Understanding the specific needs of an industry plays a crucial role in selecting the right data analysis tool. When challenges can be met with tailored solutions, the potential for growth and efficiency is remarkable.
Common Challenges in Data Analysis Tool Selection
Selecting the right data analysis tool isn't always a walk in the park. Various factors complicate the decision-making process, making it crucial for professionals to be aware of potential pitfalls. A deep dive into these common challenges reveals not only their significance but also how they impact the overall effectiveness of data analysis efforts.
One of the major hurdles is data quality and availability. Without quality data, even the best analytical tools struggle to yield meaningful insights. Inaccurate, incomplete, or outdated data can lead to flawed conclusions and misguided decisions. Moreover, ensuring that the required data is readily available and easily accessible is another crucial aspect. Organizations often grapple with silos of information scattered across various departments. This fragmentation hinders comprehensive analysis, making it essential to have a clear data governance strategy in place before tool selection.
Change management and training needs also emerge as prominent challenges. As firms adopt new tools, they often face resistance from team members who are attached to legacy systems or who may feel overwhelmed by the learning curve associated with innovative software. Preparing the workforce for this transition means not only investing in proper training programs but also fostering a culture that embraces change. A successful implementation hinges on communicating the benefits and providing adequate support throughout the training process.
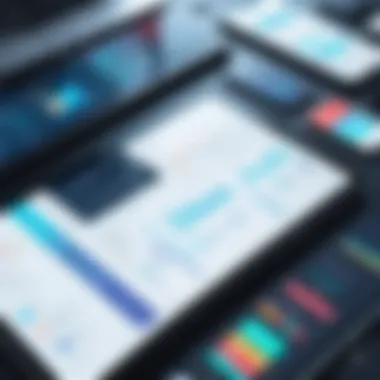
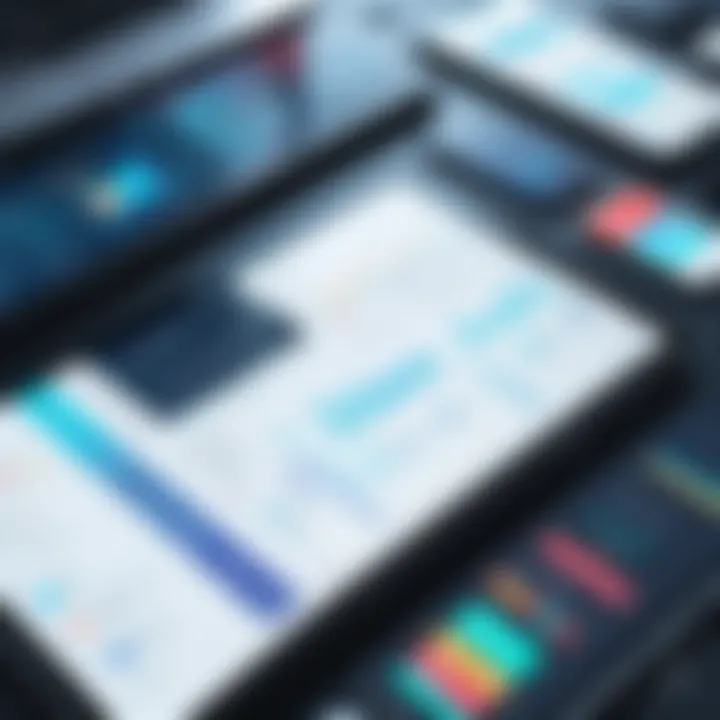
In the high-stakes world of data analysis, overlooking these challenges can spell disaster. Thus, equipping teams with the right tools requires a thoughtful approach that considers these common obstacles.
Data Quality and Availability
Data quality is not just a metric; it's the bedrock upon which sound decisions stand. When one considers the myriad of data sources available today—from IoT devices to social media feeds—one quickly realizes that ensuring data quality becomes both a priority and a challenge. Inaccurate data can mislead analyses and, consequently, decision-makers. Organizations must focus on setting up data validation processes and quality checks at the onset.
Availability of data adds another layer to this complexity. A tool might be star-studded with features but if it doesn't connect to the data sources you rely upon, its value diminishes rapidly. Professionals must meticulously assess whether the selected tool can interface effectively with existing databases or data streams. Organization-wide cooperation is vital to know where data is kept, its formats, and how to access it efficiently.
Change Management and Training Needs
Implementing a new data analysis tool isn't merely about purchasing software; it’s about shifting mindsets. Teams accustomed to previous workflows may resist new systems if they perceive them as overly complicated or redundant. This reluctance can delay the realization of significant benefits promised by the new tool. As such, having a robust change management plan is non-negotiable.
Training becomes a pivotal aspect of the transition. Organizations should not underestimate the value of tailored training programs designed to address specific needs of different user groups within the organization. From hands-on sessions to comprehensive guides, the goal is to ensure users gain confidence and competence in navigating the new tool. This ensures the innovation becomes a boon rather than a burden.
As decision-makers strategize on tool selection, acknowledging and addressing these challenges upfront fosters smoother transitions and maximizes the potential of data analysis initiatives.
Future Trends in Data Analysis Tools
As we dive into the world of data analysis, it’s crucial to stay ahead of the curve. Technologies and methodologies are changing at a pace once thought impossible. Future trends in data analysis tools are not just fascinating; they’re essential for organizations aiming to remain competitive. Understanding these trends can shape strategies, empower decision-makers, and enhance analytical capabilities.
Incorporating new tools can lead to efficiencies that reverberate across an organization. For instance, embracing advanced analytics puts businesses in a better position to identify patterns, predict trends, and derive actionable insights from vast data sets. The integration of cutting-edge technologies transforms the way we handle data, making future trends a pivotal consideration in tool selection.
Artificial Intelligence Integration
AI is not just a buzzword; it's empowering the next wave of data analysis tools. The marriage of artificial intelligence and data analytics paves the way for smarter, faster decision-making. AI-driven tools can handle complex data sets more efficiently, identifying correlations and anomalies that a human analyst might miss. As companies gather more data than they can practically analyze, these capabilities are invaluable.
A few key benefits of AI integration include:
- Predictive Analysis: With machine learning algorithms, tools can anticipate future trends based on historical data, allowing businesses to strategize accordingly.
- Automated Insights: AI systems can generate reports without human intervention, saving significant time and reducing the risk of human error.
- Natural Language Processing: This technology helps users query data simply by talking or typing in natural language, making data analysis accessible to non-technical staff.
However, organizations must also consider the challenges of AI in data analysis. Concerns about data privacy, bias in algorithms, and the need for skilled personnel can complicate a straightforward adoption. Therefore, thoughtful planning becomes paramount to harness AI’s full potential and avoid pitfalls.
Real-Time Data Analysis
The demand for real-time data analysis is on the rise. In an age where instantaneous decision-making can mean the difference between success and failure, organizations are investing in tools that provide real-time analytics. Information today is not just about what happened; it’s about acting on what’s happening now. This shift emphasizes the importance of having tools that can process and analyze data on-the-fly.
Benefits of real-time data analysis include:
- Improved Decision Making: Real-time insights allow businesses to pivot strategies rapidly in response to market changes.
- Enhanced Customer Experience: Companies can react to customer behaviors instantly, optimizing engagement and satisfaction levels.
- Operational Efficiency: Automation of analysis processes in real time reduces downtime and improves productivity.
Despite its perks, organizations must tread carefully. Implementing real-time analytics might mean higher costs and demand for robust infrastructure. Coupled with the need for constant monitoring, the pursuit of real-time insight can strain even well-resourced companies.
"Embracing trends like AI and real-time analysis is not merely about adopting new tools; it's about reshaping business strategies and rethinking how organizations leverage data."
In summary, organizations looking to invest in data analysis tools must fully understand the forthcoming trends. Integrating AI and adopting real-time capabilities are no longer optional but rather vital in maintaining relevance and fostering growth. By approaching these trends thoughtfully, decision-makers can ensure they are well-positioned to tap into the full potential of their data.
Culmination and Recommendations
In the realm of data analysis, arriving at the right conclusions is crucial for both operational efficiency and strategic decision-making. A well-chosen tool not only supports users in their immediate analysis needs but also future-proofs their investment in technology. The outcome of any analysis hinges on the integrity of the data and the functionalities of the tools at hand. Hence, users must take a methodical approach when selecting their data analysis tools.
User engagement is paramount. Often, it is not merely about the tool’s capabilities but how intuitive and user-friendly it is for the team members who will be engaging with it daily. Tools that align with the team’s workflow can enhance productivity—saving both time and resources.
On the other hand, the integration of the chosen tool into the current tech ecosystem is significant. Seamless interoperability can facilitate collaboration and data sharing, eliminating unnecessary friction. This connection extends across platforms to ensure that the analyses conducted are not only reliable but also actionable.
Cost-effectiveness is a key consideration. In many scenarios, the most robust tool may not be the one that fits the budget, which often leads to decisive compromises. Companies need to evaluate not just the upfront costs but also the long-term value that a tool can provide.
Ultimately, the goal is to strike a balance between features, usability, and cost. With a careful assessment utilizing the insights drawn throughout this article, decision-makers will be well-equipped to navigate the complex landscape of data analysis tools.
"Choosing the right tool is a bit like picking the best horse in a race—it's not always about the one with the flashiest looks, but who can actually deliver results."
Summarizing Key Insights
In summarizing our findings, this article sheds light on several pivotal aspects surrounding the selection of data analysis tools:
- Functionality vs. Usability: While a tool loaded with features might seem enticing, if it’s not user-friendly, it may hinder productivity.
- Integration Capabilities: Tools must be compatible with existing systems; hence, assessing integration options is crucial.
- Economic Factors: The total cost of ownership should be calculated, comprising setup, operational, and maintenance costs.
By analyzing these factors, professionals can make informed decisions that align with their unique analytical needs and business goals.
Guidance for Final Tool Selection
When it comes to finalizing a tool for data analysis, a few guiding principles can steer professionals in the right direction:
- Define Your Requirements: Clearly outline what your analysis needs are. Engage relevant stakeholders in this discussion to gain insights on what functionalities are critical for them.
- Trial and Testing: Leverage free trials or limited versions to test tools in a live environment. Actual experience will reveal a tool’s practicality and compatibility.
- Seek Feedback: Regularly consult users post-selection to gather feedback regarding the tool’s performance. Their insights can aid in further optimizing the usage of the tool.