Understanding Data Collection Apps and Behavior Analysis
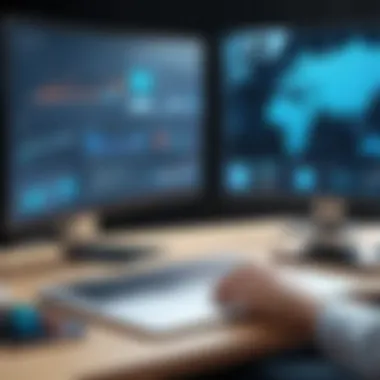
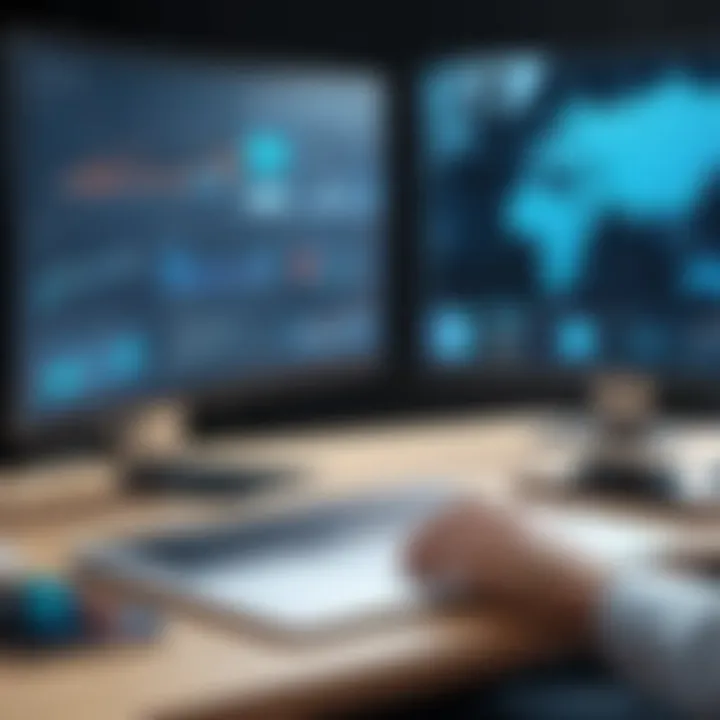
Intro
In today’s world, the collection of data plays a pivotal role across many sectors. The rise of data collection apps has allowed organizations to gather information efficiently, leading to a deeper understanding of behaviors and trends. Behavior analysis, when combined with data collection, forms a foundation for informed decision-making. This article explores how these two elements work together, emphasizing essential features of data collection applications and their implications in various contexts.
Overview of Core Features
Data collection applications are equipped with key functionalities that streamline the gathering and analysis of information. If we examine these core features, they often include:
- User-Friendly Data Input: Ease of data entry ensures that users can efficiently input the necessary information without technical barriers.
- Real-Time Data Processing: Many applications deliver insights in real-time, allowing for immediate responses to emerging trends.
- Comprehensive Reporting Tools: Effective reporting functions enable stakeholders to visualize data and understand findings at a glance.
- Integration Capabilities: Good applications often integrate with existing systems, enabling a smooth flow of data between various tools and platforms.
When comparing available software, one might notice differences in features. For instance, Qualtrics provides advanced survey tools and analytics that can tailor data collection to specific needs, while Tableau excels in data visualization but lacks direct data entry functionalities.
Essential Functionalities
The essential functionalities of data collection apps must cater to diverse sectors, including healthcare, marketing, and education. Some critical functionalities include:
- Filtering and Segmentation: Users should be able to filter data based on specific criteria to analyze targeted segments effectively.
- Data Security: Ensuring the safety of the collected data is crucial. Apps must comply with regulations such as GDPR to protect user privacy.
- Mobile Compatibility: As many users operate on mobile platforms, mobile-friendly designs are important for accessibility.
User Experience and Interface
The user experience (UX) and user interface (UI) significantly impact how users interact with data collection apps. A well-designed interface not only looks appealing but also enhances usability.
- Insights into UI/UX Design Aspects: Clean design with logical navigation creates an intuitive experience. Users should know where to input data and how to retrieve insights without unnecessary confusion.
- Importance of Usability and Accessibility: Creating apps that are easy for many different users is key. This includes accessibility features for individuals with disabilities, ensuring that everyone can benefit from data insights.
"A well-designed user experience is not just about making things pretty; it’s about making them functional and intuitive."
Prolusion to Data Collection Apps
In today's data-driven world, understanding data collection apps becomes pivotal for any organization looking to leverage insights for strategic decisions. These applications serve as the foundation for gathering essential information that can inform behavior analysis. Organizations can choose from various tools that simplify this process, ensuring data collection is both efficient and effective.
Definition and Purpose
Data collection apps are software tools designed to gather, store, and analyze data from diverse sources. They vary in functions and can include mobile applications, web platforms, or specialized software that caters to specific needs. The primary purpose of these tools is to facilitate the systematic collection of information, making it easier to analyze trends and behaviors over time. They enable users to collect quantitative or qualitative data, depending on their objectives.
The significance of using data collection apps lies in their ability to centralize data entry. By doing so, they minimize human error and reduce the time spent on data management. Organizations can conduct surveys, track customer behavior, and monitor employee performance seamlessly with these platforms. Furthermore, these tools often come equipped with data visualization capabilities, which help users interpret complex data sets more easily.
Evolution of Data Collection Technologies
The landscape of data collection technologies has undergone a profound transformation over the last few decades. Initially, data collection relied heavily on paper-based methods, which were not only labor-intensive but also susceptible to inaccuracies. As technology advanced, electronic forms and spreadsheet programs emerged, offering more efficient data gathering methods.
The introduction of the internet brought about significant changes. Online surveys became prevalent, enabling organizations to reach broader audiences without geographical limitations. Additionally, mobile technology has transformed data collection further. With the proliferation of smartphones, organizations now have the capacity to collect real-time data from users' environments. Users can fill out surveys on their devices, providing immediate feedback that was not possible with traditional methods.
Today, advanced data collection apps also incorporate artificial intelligence (AI) to enhance data accuracy and analysis capabilities. Features such as automatic data cleaning and pattern recognition are now commonplace. These innovations allow professionals to derive valuable insights from their data while minimizing manual intervention, thus increasing operational efficiency. As a result, decision-makers now possess powerful tools enabling them to make informed choices based on robust datasets.
Importance of Behavior Analysis
Behavior analysis plays a critical role in shaping decision-making processes across various sectors. By offering a structured approach to understanding human actions, behavior analysis helps organizations grasp deeper insights into patterns and trends. This understanding enhances their capability to develop tailored strategies, ultimately leading to improved outcomes.
One primary element of behavior analysis is contextual understanding. Observing actions in the environments where they occur offers clarity on the underlying motivations and triggers. For instance, analyzing consumer behavior in retail settings reveals not just preferences but also influences like layout and product placement. This context allows decision-makers to modify their approaches, making them more effective.
Moreover, behavior analysis bridges the gap between abstract data and tangible results. Organizations can systematically gather, analyze, and interpret behavior-related data to inform policies and practices. The direct benefits include increased efficiency, productivity, and innovation. Businesses can prioritize initiatives that yield the best returns based on rigorous analysis.
Key Considerations in Behavior Analysis
- Behavior analysis is adaptable across different fields, including education, marketing, healthcare, and organizational management.
- It provides frameworks for evaluating effectiveness, enabling continuous improvement.
- Evaluating behavioral patterns over time equips organizations with predictive capabilities, aiding in proactive decision-making.
"Understanding behavior is essential for crafting informed strategies that resonate with individuals."
Additionally, behavior analysis supports ethical practices. It encourages transparency and accountability, ensuring that data collection processes respect individual privacy. By incorporating ethical considerations into behavior analysis, organizations foster trust and confidence among stakeholders.
In summary, the importance of behavior analysis cannot be overstated. It provides a foundation for data-driven decision-making, enhances understanding of individual and group actions, and ultimately helps organizations tailor their strategies to meet specific needs. With an emphasis on context, methodologies, and ethical practices, behavior analysis becomes a vital tool for those aiming to navigate the complexities of modern society.
Types of Data Collection Apps
Understanding the various types of data collection apps is crucial in leveraging technology for effective behavior analysis. These apps serve unique purposes, tailored to specific needs within different industries. By recognizing the strengths of each type, users can make informed decisions that improve organizational efficiency, enhance data gathering, and optimize behavior analysis strategies.
Surveys and Questionnaires
Surveys and questionnaires remain foundational tools for collecting data on attitudes, opinions, and preferences. These methods enable researchers to reach a wider audience, gathering quantitative data efficiently. Typical platforms include Google Forms and SurveyMonkey. These tools provide customizable templates that allow for diverse question formats, such as multiple choice, rating scales, and open-ended questions. The flexibility of these tools is an advantage, as they can be quickly adapted to fit various research goals.
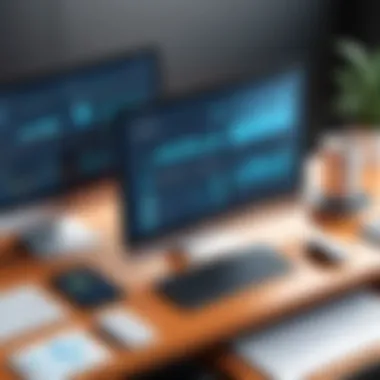
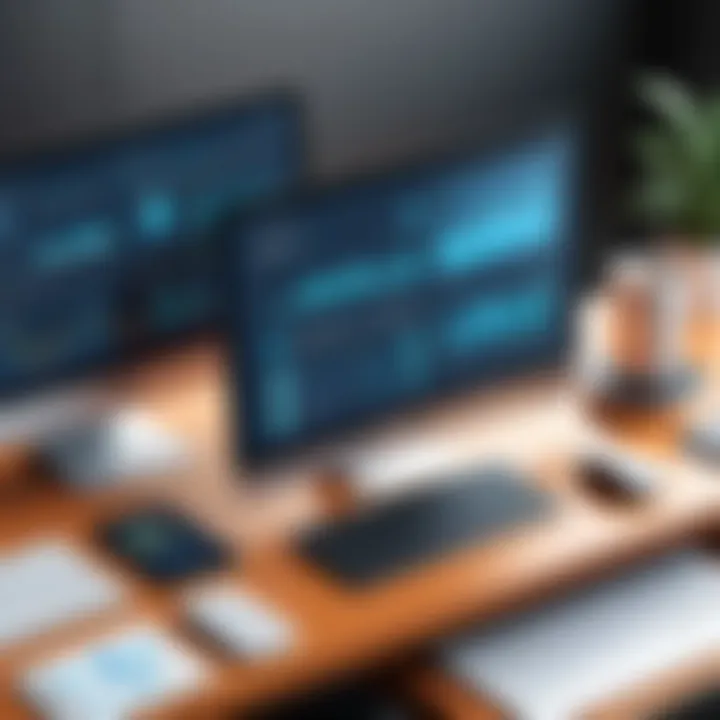
Key benefits of surveys and questionnaires include:
- Scalability: They can reach thousands of respondents simultaneously.
- Cost-Effectiveness: Many platforms offer free versions, reducing the overall budget for data collection.
- User Control: Respondents can complete surveys at their convenience, providing a better user experience.
However, designing effective surveys requires careful consideration of questions. Poorly framed questions may lead to biased responses. Thus, piloting the survey with a small sample can be useful to refine questions before full deployment.
Mobile Apps for Behavior Tracking
Mobile apps designed for behavior tracking offer real-time data collection capabilities. These applications allow users to monitor behaviors on-the-go, which is particularly beneficial in fields like psychology, healthcare, and marketing. Apps such as MyFitnessPal or Habitica exemplify how mobile technology can encourage behavioral change through self-monitoring and immediate feedback.
The effective use of mobile apps presents several advantages:
- Accessibility: Users can input data from anywhere, improving engagement.
- Instant Feedback: Many apps provide users with immediate insights about their behavior changes, promoting motivation.
- Data Visualization: Visual representations of data trends help users understand their behavior over time.
Nonetheless, it is essential to assess data security features when choosing a mobile app. Personal health or behavioral data is sensitive; therefore, ensuring that the app adheres to privacy laws is paramount.
Web-Based Tools
Web-based tools are increasingly popular for data collection due to their versatility and extensive features. These platforms typically allow for real-time collaboration, making them ideal for team projects and large-scale analyses. Examples include Qualtrics and Typeform, which facilitate the creation of detailed surveys and allow for complex data integration.
Benefits of these tools encompass:
- Integration Capabilities: They can easily integrate with other software, enhancing the data analysis process.
- Collaboration: Teams can work simultaneously, leading to faster project completion.
- Comprehensive Data Analysis: Many tools come with built-in analytics that help users interpret data trends effectively.
While these applications are powerful, users should ensure they have a robust internet connection for optimal performance. Moreover, selecting a tool that fits organizational workflows can lead to significant efficiencies in the data collection process.
"Choosing the right type of data collection app is vital. It can influence not only the quantity of data gathered but also the quality of insights derived from that data."
Key Features of Data Collection Apps
Understanding the features of data collection applications is crucial for decision-makers and IT professionals. These features determine how effectively the applications can serve their purpose in gathering and analyzing behavior-related data. The right tools can streamline processes, enhance user experience, and ensure security, all of which directly impact productivity and decision-making.
Real-Time Data Collection
Real-time data collection is one of the most significant features of modern data collection apps. This capability allows users to gather information as it happens, which can be critical for timely decision-making. The immediacy of data can lead to more informed choices that stem from current trends rather than past occurrences.
Here are some key advantages of real-time data collection:
- Timeliness: Decisions based on the latest data yield more relevant insights.
- Flexibility: Organizations can adapt strategies quickly in response to new information.
- Enhanced Engagement: Users can interact with data in a manner that reflects real-time changes in behavior.
"Real-time data collection empowers organizations to act immediately on insights, fostering a more dynamic work environment."
User-Friendly Interfaces
A user-friendly interface is essential for data collection apps aimed at a broad audience. Apps designed with simplicity and intuitiveness can encourage widespread use across various skill levels. This factor minimizes training time and maximizes data accuracy by allowing users to focus on the task rather than the tool.
The importance of user-friendly interfaces is underscored by several considerations:
- Accessibility: Simple navigation enables users to find and enter data quickly.
- Training: Reduces the need for extensive training, saving time and resources.
- Error Reduction: Intuitive designs can decrease the likelihood of user errors.
Data Security and Privacy
In an age where data breaches are a common concern, data security and privacy are paramount features of data collection apps. Protecting sensitive information is not only a legal obligation but also essential for maintaining user trust and credibility. Good applications must comply with data protection regulations and implement strong security measures.
Key aspects of data security and privacy to consider include:
- Encryption: Safeguarding data during transmission to prevent unauthorized access.
- Access Controls: Ensuring only authorized staff can view sensitive information.
- User Anonymity: Collecting data in a manner that respects user privacy, making it more acceptable to participants.
By focusing on these features—real-time data collection, user-friendly interfaces, and robust data security—organizations can select data collection apps that effectively serve their needs while upholding ethical standards.
Methodologies in Behavior Analysis
Behavior analysis relies on established methodologies to effectively understand and manipulate behavior in various contexts. By selecting appropriate methodologies, professionals can enhance the reliability and validity of their findings. This segment discusses key methodologies, focusing on quantitative and qualitative methods, observational studies, and experimental designs. Understanding these methodologies equips decision-makers and IT professionals with the tools needed to draw meaningful conclusions from data, ultimately driving better decisions and strategies.
Quantitative vs. Qualitative Methods
Quantitative methods involve the collection and analysis of numerical data. This approach offers a structured and statistical perspective on behavior, allowing analysts to discern patterns and correlations. For instance, surveys may yield data points that reveal trends across large populations.
On the other hand, qualitative methods focus on understanding the underlying reasons and motivations behind behaviors. These methodologies might include interviews or focus groups that provide insights into individual thoughts and feelings. The balance between quantitative and qualitative methods can enrich the analysis. While quantitative data help in measuring a wide array of behaviors, qualitative data introduce context that numbers alone cannot convey.
Observational Studies
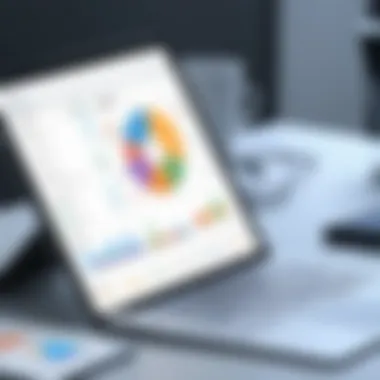
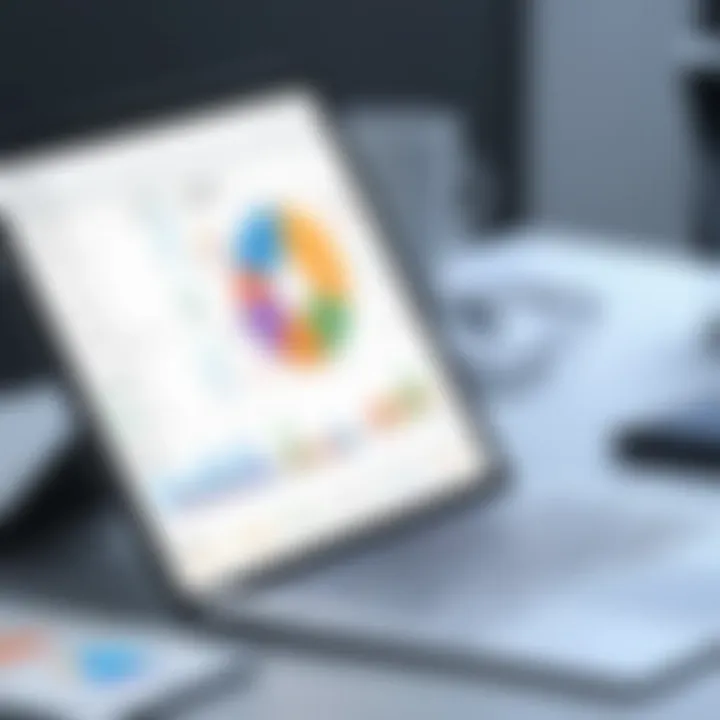
Observational studies are a powerful tool in behavior analysis. They involve watching and recording behaviors in real-time without interaction. This passive data collection means the behavior is not influenced by the observer, resulting in more authentic data.
These studies can be particularly useful in settings like classrooms or workplaces, where understanding interactions can lead to improvements in dynamics and productivity. However, observational methods come with challenges. Observers must be vigilant to avoid bias and ensure consistent data recording. Therein lies the importance of training for observers to recognize when their perceptions may alter their reporting.
Experimental Designs
Experimental designs take behavior analysis further by introducing manipulation of variables to observe effects. This method is foundational in establishing cause-and-effect relationships. In a typical experiment, researchers will create conditions to test a hypothesis, utilizing control and experimental groups for comparison.
For example, in marketing, a company might change an element of its campaign to see if the alteration influences consumer behavior. This design can yield strong insights, but careful consideration is necessary to ensure that all variables are accounted for. Proper design can clearly demonstrate how specific changes impact behavior, guiding future strategies effectively.
Successful applications depend on a fundamental understanding of methodologies, as this shapes the precision and applicability of the findings.
Each of these methodologies contributes uniquely to the broader field of behavior analysis. By integrating quantitative and qualitative methods, utilizing observational studies, and implementing experimental designs, analysts can form a comprehensive view of behavioral trends. This holistic approach is essential for crafting informed strategies in various sectors.
Integrating Data Collection with Behavior Analysis
Integrating data collection with behavior analysis plays a crucial role in enhancing the accuracy and effectiveness of behavioral insights. This integration allows organizations to gather comprehensive data that captures the complexities of human behavior. Without this synergy, data may lack context. Hence, the conclusions drawn might be misleading or incomplete.
The combination of qualitative and quantitative data is one of the most effective methods in this integration process. By blending both types of data, researchers and analysts can paint a fuller picture of behavioral patterns. Qualitative data often provides rich insights into motivations and feelings, while quantitative data offers measurable evidence of behaviors and trends. This dual approach enables organizations to develop well-rounded strategies that are grounded in actual behavior rather than assumptions.
Combining Qualitative and Quantitative Data
Combining qualitative and quantitative data is an essential factor for informed decision making. Each type of data contributes distinct advantages. Qualitative data often highlights an individual's perspective. This can include interviews, open-ended survey responses, or observational notes. These insights can reveal underlying feelings that numbers alone cannot uncover.
On the other hand, quantitative data offers rigorous statistical analysis. It uses numerical data, such as survey scales and metrics, to provide objective evidence about trends or patterns. Merging both data forms generates a more robust analysis. For example, if a behavioral study shows that employees feel overworked, the qualitative narrative can support the statistics revealing lower productivity rates. Using both types of data not only adds depth but also broadens understanding, allowing for more effective solutions.
Creating Comprehensive Reports
After integrating data collection with behavior analysis, creating comprehensive reports becomes vital. A well-structured report synthesizes the findings from both qualitative and quantitative analyses. This structure fosters clearer communication of insights and recommendations. Reports should include methodologies, data sources, key findings, and actionable recommendations.
When creating these reports, it is important to consider the audience. Decision-makers often prefer concise, targeted data visualizations, while stakeholders may require a more detailed narrative. Additionally, creating an executive summary at the beginning of the report ensures that the essential insights are visible right away.
Utilizing data visualization tools, such as Tableau or Power BI, can enhance the report's presentation. These tools can help in transforming complex data sets into more accessible formats, allowing for a quicker grasp of critical insights.
Integrating both qualitative and quantitative data leads to richer insights and more effective behavior analysis practices. By effectively communicating these insights through comprehensive reports, organizations can drive better decision-making.
Selecting the Right Data Collection App
Selecting the right data collection app is crucial for organizations that seek to make informed decisions based on reliable data. Each app comes with unique features and capabilities. Thus, understanding which app aligns best with an organization’s goals can significantly impact the quality of the data collected and the subsequent analysis. Investing time into this selection process will yield better insights and opportunities for improvement.
Assessing Organizational Needs
Assessing organizational needs involves a thorough evaluation of what data is necessary to fulfill specific objectives. Different organizations may have different requirements based on their industry, size, and operational goals. When choosing an app, consider the following elements:
- Data Type: Identify what type of data is crucial. For instance, companies focusing on customer satisfaction might prioritize survey tools, while those concerned with employee performance may need tracking applications.
- Integration: Examine how the app will fit into existing systems. Compatibility issues can lead to inefficiencies and increased costs, so choose an app that integrates seamlessly with current tools.
- User Access: Understand who will be using the app. The app should be user-friendly and suitable for the varying levels of tech-savviness among staff. A complicated interface may deter users and affect data quality.
- Budget: Align the app's costs with your available budget. Evaluate both initial purchases and ongoing costs, like subscriptions or maintenance fees.
"Understanding your organizational needs is the first step towards effective data collection."
Taking inventory of these factors ensures that the app selected not only meets immediate data collection requirements but also adapts to future needs as the organization grows.
Comparative Analysis of Available Tools
With numerous data collection apps available, comparative analysis is essential. Focus on aspects such as:
- Features: Look at the functionalities offered by each app. Some may provide advanced analytics, while others focus solely on collecting data.
- Ease of Use: Test the user interface. An intuitive design can lead to higher user engagement and thus more accurate data collection.
- Customer Support: Investigate the support options available. Reliable customer service can assist in fixing issues promptly, minimizing downtime.
- User Reviews: Read user testimonials and reviews to gain insight into others' experiences. This can help identify any potential drawbacks that may not be immediately apparent.
Make a list of potential options and evaluate them against your organizational needs. This practice not only narrows down the choices but also highlights the pros and cons of each option, making the final decision more informed.
In summary, the selection of an appropriate data collection app involves careful consideration of organizational needs and a detailed comparative analysis of available tools. This systematic approach leads to better data-driven results and fosters a culture of informed decision-making within the organization.
Ethical Considerations in Data Collection
In the realm of data collection apps and behavior analysis, ethics plays a crucial role. As organizations increasingly rely on data to inform decisions, the need to navigate complex ethical landscapes becomes vital. Ethical considerations ensure that the data collection processes respect the rights of individuals, maintain integrity, and foster trust. The growing awareness of these issues influences public perceptions and organizational reputation, making ethical practices non-negotiable in research and analytics.
Informed Consent
Informed consent is a bedrock principle of ethical data collection. This notion implies that participants should be fully aware of the data being collected, how it will be used, and any potential risks. Providing clear and concise information is essential in this process. \
Organizations should take steps to ensure understanding, and they must obtain explicit permission before collecting any data. A transparent consent form helps participants make informed decisions while promoting trust in the data collection process. Additionally, continuous dialogue about data use may strengthen the relationship between data collectors and participants.
Data Anonymity and Confidentiality
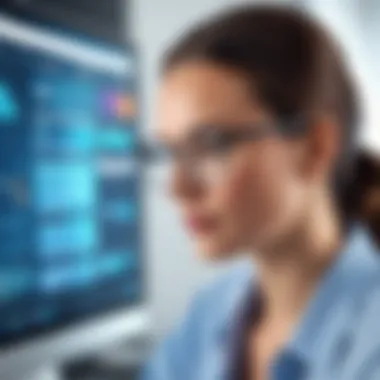
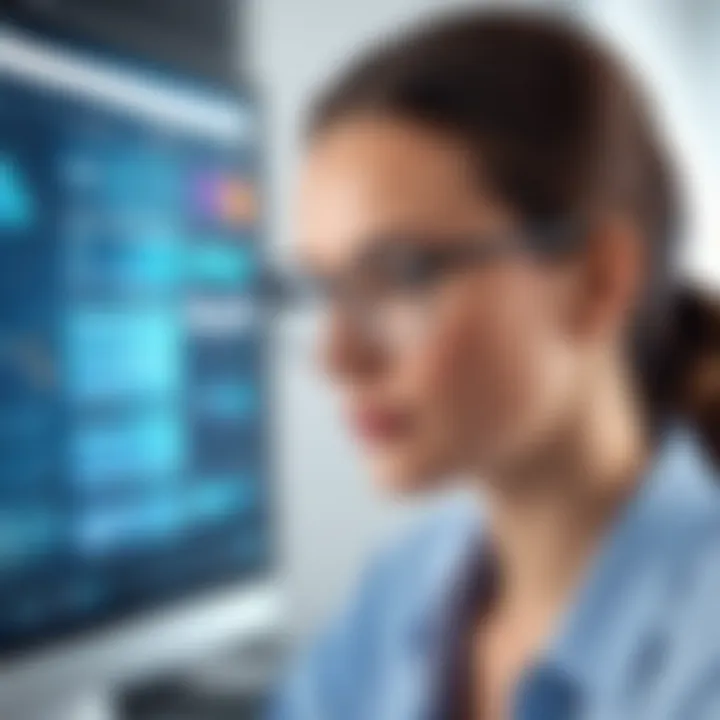
Data anonymity and confidentiality are interrelated concepts that safeguard individual privacy. Maintaining anonymity means that a participant's identity cannot be linked to the data they provide. This approach is particularly important when sensitive information is involved. By allowing individuals to contribute data without revealing their identity, organizations can encourage participation and obtain honest feedback.
Confidentiality goes a step further by ensuring that any identifying information collected during the research remains protected. Organizations must implement strong data protection protocols and restrict access to authorized personnel only. Failure to uphold these standards can lead to breaches of trust and potential legal consequences. \
"Respect for data subjects’ privacy is a key tenet of ethical behavior in data collection."
Establishing policies surrounding data handling and sharing helps build confidence in the data collection process. Organizations that prioritize ethical considerations are more likely to achieve long-term success, fostering a culture of integrity that enhances data-driven decision-making.
Future Trends in Data Collection and Analysis
Data collection apps are evolving rapidly in the face of technological advancements and changing user needs. This section will explore two prominent trends that are shaping the future of data collection and analysis, namely artificial intelligence and predictive analytics. Understanding these trends is essential for decision-makers who aim to leverage data effectively for strategic initiatives.
Artificial Intelligence in Data Processing
The incorporation of artificial intelligence (AI) into data processing represents a significant shift in how data is collected, analyzed, and interpreted. AI enhances data collection apps by automating various processes, leading to increased efficiency and accuracy. Through machine learning algorithms, these applications can identify patterns and derive insights from large volumes of data that were previously difficult to analyze manually.
Some key benefits of implementing AI in data processing include:
- Enhanced Data Accuracy: AI algorithms can minimize human error in data entry and analysis, ensuring more reliable outputs.
- Real-Time Processing: With AI, data can be processed in real-time, allowing organizations to make quicker, more informed decisions based on the latest information.
- Scalability: AI systems can scale easily as data volume increases, ensuring that organizations can handle larger datasets without a decrease in performance.
However, organizations must consider some factors when integrating AI into their data collection strategies. This includes ensuring data quality, addressing privacy concerns, and establishing robust frameworks for ethical AI usage. As data privacy laws become more stringent, businesses will face challenges in maintaining user trust while utilizing AI technologies.
Predictive Analytics
Predictive analytics is another key trend in the data collection landscape, enabling organizations to forecast future outcomes based on historical data. This analytical approach utilizes statistical algorithms and machine learning techniques to identify patterns and trends that can inform decision-making processes.
The significance of predictive analytics lies in its ability to:
- Improve Decision-Making: By understanding potential future scenarios, organizations can make better strategic choices, reducing risks associated with uncertainty.
- Enhance Customer Experiences: Predictive analytics can help organizations anticipate customer needs and preferences, leading to tailored services and improved satisfaction.
- Optimize Operational Efficiency: By predicting trends and outcomes, businesses can allocate resources more effectively and enhance overall productivity.
Organizations are adopting predictive analytics to remain competitive and responsive to market dynamics. However, it is vital to recognize the limitations and possible inaccuracies in predictions, ensuring that these tools support, rather than dictate, business strategies.
In summary, the future of data collection and behavior analysis will be significantly shaped by advancements in artificial intelligence and predictive analytics. Embracing these trends can provide a competitive edge while also necessitating careful consideration of ethical and operational challenges.
Case Studies
Case studies serve as an essential component in understanding the practical applications of data collection apps and behavior analysis. They provide real-world examples that illuminate how theories translate into action. Through case studies, one can see the effects of data-driven approaches in diverse environments. Exploring these outcomes allows professionals to appreciate the tangible benefits and challenges faced during implementation.
Success stories showcase the promise of behavior analysis. They reveal how certain techniques led to improved results. On the other hand, less successful cases teach valuable lessons. Such insights refine future practices, ensuring better preparedness in approaching similar situations. This balance of success and struggle helps in forming a well-rounded understanding of the landscape.
Additionally, case studies often highlight the methodologies employed. They can include both qualitative and quantitative measures, demonstrating a comprehensive approach to data gathering. As readers engage with these narratives, they can derive inspiration while contemplating their unique context. Importantly, recognizing the ethical considerations in these implementations is crucial, as they ensure respect for privacy and integrity in data handling.
"Case studies are not just about success; they provide a complete landscape by showcasing both achievements and obstacles."
Successful Implementation in Business
In the realm of business, successful implementations of data collection apps often become benchmarks for others to follow. A notable example is how companies like Starbucks use these applications to gather customer feedback and operational data effectively. By analyzing this data, Starbucks has optimized their menu offerings and improved the overall customer experience.
The benefits of successful implementations are multifaceted. They can lead to enhanced productivity, increased customer satisfaction, and better decision-making processes. For instance, organizations that utilize behavior analysis can identify patterns in customer preferences, allowing for trends to guide marketing strategies. Furthermore, aligning business goals with real-time data empowers teams to work more efficiently.
Educational Applications
In educational settings, data collection apps are transforming how institutions approach learning and development. Schools and universities are beginning to harness these tools to track student performance and engagement. For instance, tools like Google Classroom allow educators to collect and analyze data regarding student participation and assignment completion.
This data can reveal insights into learning patterns. Such patterns help teachers adjust their methods to better meet students' needs. Moreover, educators are utilizing analytics to identify at-risk students and providing early interventions.
By employing behavior analysis in education, institutions are improving learning outcomes. Through proper application of data, they are driving innovations in pedagogy and curriculum development. This approach results in a more personalized educational experience, catering to individual learner needs.
Ending
The conclusion is a critical part of this article as it encapsulates everything discussed regarding data collection apps and behavior analysis. A well-structured conclusion ties together the insights shared throughout the text while emphasizing the importance of effectively using data in decision-making processes.
Summarizing Key Insights
In examining the interplay between data collection applications and behavior analysis, several key insights emerge:
- Integration of Technology and Behavior: Understanding behavior through technology enhances data accuracy. This intersection is vital for organizations looking to adapt and respond to user needs.
- Diverse Methodologies: The use of both qualitative and quantitative methods provides a comprehensive view of behavior. Each method offers unique advantages and insights, making them valuable when applied appropriately.
- Ethical Considerations: It is essential to prioritize ethical practices in data collection. Ensuring data anonymity and obtaining informed consent are not just legal imperatives, but critical to building trust with users and stakeholders.
These insights reinforce how data collection apps can evolve the way organizations analyze behavior, leading to informed decision-making.
Final Thoughts on Data Collection and Behavior Analysis
As we move forward, the landscape of data collection and behavior analysis continues to evolve, influenced by rapid technological advancements and changing societal norms. For decision-makers, IT professionals, and entrepreneurs, embracing these changes is important for sustainable success.
Investing in the right data collection tools can lead to significant improvements in understanding consumer behavior, market trends, and organizational efficiency.
Moreover, the significance of data privacy and ethical considerations cannot be overstated. Organizations must remain vigilant in how they handle data to maintain credibility and foster positive relationships with clients and users.