A Deep Dive into KNIME Competitors and Alternatives
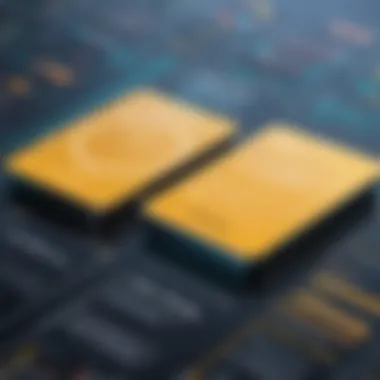
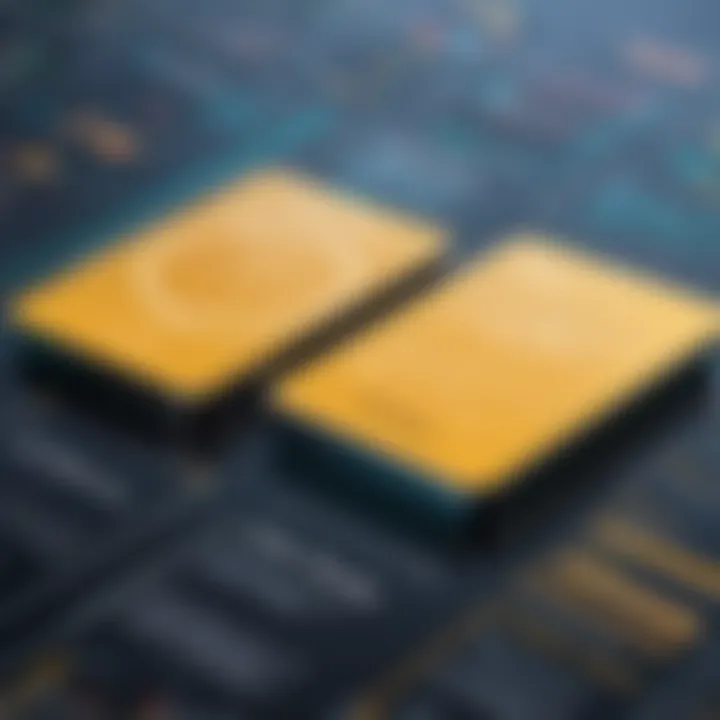
Intro
In recent years, the demand for data analytics tools has surged significantly. As organizations harness the power of data, choosing the right analytical platform becomes critical. KNIME stands out as a notable player in the field. However, it is essential to understand the competitive landscape that surrounds it. This examination not only elucidates the strengths and weaknesses of various KNIME alternatives but also empowers decision-makers with the information necessary to select the most suitable tools for their needs.
By analyzing various platforms, this article aims to present a thorough understanding of core features, user experience, and market position of competing software. The goal is to provide business leaders, IT specialists, and entrepreneurs the insights they need to enhance their data strategies effectively.
Overview of Core Features
Understanding the core features of data analytics platforms is vital for discerning their potential. Most competitors offer functionalities related to data integration, processing, and visualization.
Description of essential functionalities
Key functionalities of data analytics tools include:
- Data Preparation: Efficient data cleaning and transformation tools are necessary for accurate analysis.
- Machine Learning: Many platforms integrate machine learning capabilities that allow for predictive analytics and advanced insights.
- Collaboration: User collaboration through shared workflows increases efficiency in team environments.
- Data Visualization: Visual representations help present findings clearly, assisting stakeholders in understanding complex results.
Comparison of features across top software options
When comparing KNIME with other platforms like Alteryx, Apache Spark, and RapidMiner, we notice distinct feature sets. For instance, KNIME excels in extensibility with numerous open-source extensions. In contrast, Alteryx offers an intuitive drag-and-drop interface that promotes ease of use for non-technical users.
"The key to selecting the right data analytics platform lies in aligning its features with organizational needs."
User Experience and Interface
The user interface and experience (UI/UX) design significantly influence how effectively users can employ the software. A well-designed interface can streamline workflows and improve productivity.
Insights into UI/UX design aspects
Platforms such as Tableau and Power BI emphasize visual appeal, creating dashboards that are both functionally rich and aesthetically pleasing. A clutter-free design fosters ease of navigation.
Importance of usability and accessibility
Usability should not be understated, as it determines how quickly a user can adapt to the tool. Accessibility is also crucial, ensuring that all users, regardless of their technical background, can benefit from the platform. This aspect can affect overall satisfaction and adoption rates within organizations.
Foreword to KNIME and Its Importance
Understanding the role of KNIME is fundamental for any organization keen on leveraging data analytics for competitive advantage. KNIME, which stands for Konstanz Information Miner, is not just a tool; it is a powerful open-source data analytics platform that integrates various components for data mining and machine learning. Its functionality provides businesses with the ability to analyze large sets of data efficiently, leading to better decision-making processes.
The importance of KNIME can be seen in its core capability to transform data visualization and analysis into accessible workflows. Decision-makers, IT professionals, and data scientists find this especially valuable as it streamlines complex processes into manageable segments. By connecting and blending data sources, users can create tailored workflows that cater to specific business needs without extensive programming knowledge.
Aside from enhancing usability, the platform’s emphasis on collaboration and flexibility makes it a cornerstone in many organizations' data strategies. This adaptability allows teams to test and iterate on their analytical models quickly. In fact, the reproducibility of workflows ensures that insights can be easily shared and adapted across departments, enhancing collaboration and maintaining consistency.
Understanding KNIME's Core Functionality
KNIME's architecture is built around the concept of nodes and workflows. Each workflow consists of several nodes that represent distinct data processing tasks. Users can drag and drop these nodes onto a workflow canvas, visually mapping out their analytical processes. This approach not only demystifies data analytics but also empowers users to manage robust data stories with ease.
Key functionalities of KNIME include:
- Data Integration: Merge and clean data from different sources seamlessly.
- Data Transformation: Apply various techniques to enhance or modify the data set as necessary.
- Machine Learning: Implement machine learning algorithms to draw actionable insights from data.
- Visualization: Generate impactful visual representations of data to facilitate understanding.
This modular design is appealing to businesses looking for a scalable solution that can evolve with their needs. By capturing the entire data processing lifecycle, KNIME enables users to focus not merely on operation but also on strategic insight.
The Role of Data Analytics in Business
Data analytics is increasingly vital for driving business success across industries. Organizations that harness data effectively can identify trends, make informed decisions, and differentiate themselves in crowded markets. As datasets continue to expand exponentially, the ability to analyze data accurately and efficiently becomes imperative.
The integration of data analytics into business practices provides:
- Enhanced Decision Making: Real-time analytical insights enable organizations to act swiftly in response to new information.
- Cost Efficiency: Streamlining analytical processes helps reduce unnecessary spending.
- Customer Insights: Understanding customer behavior through data helps tailor products and services better.
By embracing platforms like KNIME, businesses are acknowledging that data-driven strategies are no longer optional but rather essential. This proactive stance can lead to sustainable growth and profitability in an ever-evolving digital landscape.
"Data-driven decision-making is at the core of any successful modern enterprise."
In summary, the significance of KNIME within the framework of modern data analytics is considerable. As organizations navigate the complexities of data, they require robust tools that not only simplify processes but also deliver valuable insights. The following sections will delve into KNIME's competitors, providing a comprehensive understanding of your options in the data analytics arena.
The Competitive Landscape of Data Analytics Platforms
The realm of data analytics is highly dynamic, characterized by continuous evolution and innovation. Understanding the competitive landscape of data analytics platforms is crucial for organizations looking to implement effective data strategies. This section examines the key players in the market, highlighting their individual strengths, features, and the implications of their competition with KNIME.
The competitive landscape helps organizations make informed decisions by assessing various alternatives that meet specific business requirements. Companies can leverage insights from this analysis to align their data needs with suitable platforms, optimizing both time and resources. Beyond the functionalities, understanding market trends and competitors’ strategies can significantly affect implementation success and user satisfaction.
Overview of Major Competitors
Often, organizations seeking data analytics solutions encounter several platforms. Each contender offers unique capabilities. Significant competitors include Apache NiFi, RapidMiner, Alteryx, Tableau, Microsoft Power BI, SAS Viya, Tibco Spotfire, and Metabase.
- Apache NiFi specializes in data flow management, providing powerful tools for real-time data ingestion.
- RapidMiner is known for its comprehensive data mining capabilities, offering extensive support for various data science tasks.
- Alteryx stands out for its strong focus on data preparation, combining analytics seamlessly.
- Tableau is often associated with advanced visualization techniques while also offering data integration features.
- Microsoft Power BI benefits from tight integration with the Office suite, enhancing usability for businesses already embedded in that ecosystem.
- SAS Viya presents high-grade analytics solutions, particularly in industries demanding sophisticated statistical models.
- Tibco Spotfire combines analytics with data visualization, aiming for enhanced business intelligence.
- Metabase, as an open-source solution, provides a user-friendly interface designed for simplifying analytics tasks.
This diversity in offerings allows organizations to cater to varied analytics needs, from simple reporting to complex machine learning tasks. By reviewing these competitors, organizations can establish a clearer perspective on where KNIME stands among them.
Market Trends Influencing Competition
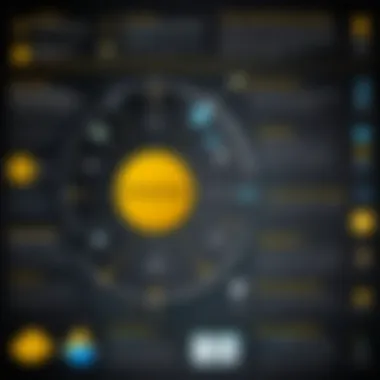
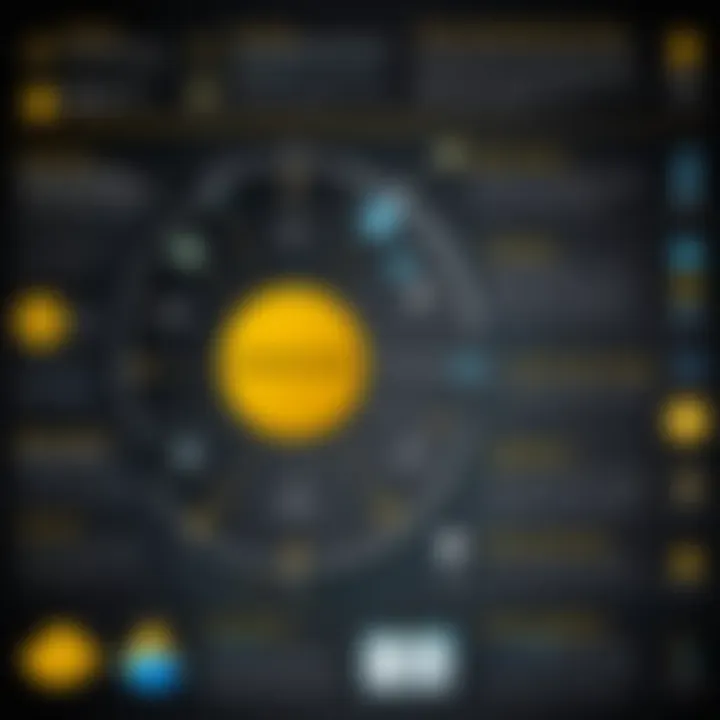
Several key trends are reshaping the competitive landscape in data analytics. One significant trend includes the rise of cloud-based solutions. More companies are migrating their operations to the cloud, which increases demand for platforms offering robust cloud capabilities.
Additionally, integration with other tools and systems is becoming a critical factor for decision-making. Companies now prioritize platforms that provide seamless interoperability with existing software stacks. Flexibility and scalability are increasingly essential, particularly as data volumes grow.
Moreover, the emphasis on self-service analytics has risen. Users demand tools that empower them to analyze data without heavy reliance on IT resources. Companies recognizing this trend are designing platforms with intuitive interfaces and guided analytics features.
Organizations must note that adapting to these trends could drastically affect their data capabilities.
Apache NiFi: A Robust Alternative
Apache NiFi stands out as a significant competitor to KNIME in the data analytics ecosystem. Its ability to handle the complexities of data flows makes it an attractive option for organizations looking to improve their data management capabilities. This section will provide a detailed exploration of what Apache NiFi offers and how it compares to KNIME.
Key Features of Apache NiFi
Apache NiFi brings a variety of features that cater to the needs of businesses managing large volumes of data. Some of the most notable ones include:
- Data Flow Automation: NiFi allows users to design and control data flows through a user-friendly interface. This function is crucial for organizations where real-time data routing is required.
- Provenance Tracking: This feature enables tracking the lineage of data from its origin to its current state. It is integral for compliance and audit purposes.
- Data Transformation: NiFi can transform data in various formats, offering support for JSON, XML, CSV, and more. This flexibility allows organizations to integrate diverse data sources.
- Security: With built-in security features such as authentication and encryption, Apache NiFi ensures that data is secure throughout its lifecycle.
- Scalability: NiFi’s architecture allows for horizontal scaling, which is beneficial for organizations that expect their data needs to grow rapidly.
Comparative Analysis with KNIME
When comparing Apache NiFi with KNIME, several factors come to light. Below are key points that delineate their strengths and weaknesses:
- User Interface: While KNIME is known for its visual programming, Apache NiFi also offers a robust graphical interface, but it emphasizes data flow management. Users familiar with data integration may find NiFi’s approach more intuitive.
- Workflow Management: KNIME provides comprehensive analytical tools, but NiFi specializes in the orchestration of data flows. For organizations where data pipeline efficiency is a priority, NiFi may present a superior solution.
- Integration Capabilities: Both platforms support integrations with various data sources and applications. However, NiFi’s focus is primarily on ensuring seamless data ingestion and delivery, which could provide an edge in environments with extensive data requirements.
- Performance: NiFi excels in speed and performance when processing large data streams, while KNIME might lag in scenarios involving real-time data processing given its focus on batch processing.
It is essential for decision-makers to carefully evaluate their specific needs when considering Apache NiFi against KNIME. Apache NiFi might be a better fit for companies that need robust data flow management and transformation capabilities, while KNIME excels in comprehensive data analytics.
RapidMiner: An Established Player
RapidMiner has solidified its position as a key player in the data analytics domain. Its importance in unlocking valuable insights from data cannot be understated. This section examines RapidMiner's offerings, looking closely at its capabilities and limitations compared to KNIME. Decision-makers can make informed choices by understanding what RapidMiner brings to the table.
Strengths of RapidMiner in the Market
RapidMiner stands out due to several strengths. One of its main advantages is its user-friendly interface, which caters to both technical and non-technical users. This accessibility encourages wider adoption across organizations.
- Comprehensive Platform: RapidMiner supports a range of data processing tasks, from data preparation to model deployment. This makes it a one-stop solution for analytics needs.
- Robust Community Support: With an active community, users can share knowledge, provide feedback, and collaborate on projects. This support network is crucial for problem-solving and innovation.
- Integration Capabilities: RapidMiner easily integrates with various data sources and platforms, including cloud services. This enhances its flexibility in diverse environments.
- Versatile Analytics Tools: The platform offers diverse analytical methods, from classic statistics to modern machine learning techniques. This versatility allows users to explore various avenues for data analysis.
"RapidMiner’s flexibility allows companies to harness their data with ease, making it a crucial tool for innovation in analytics."
Limitations relative to KNIME
While RapidMiner has its strengths, it is important to recognize its limitations in comparison to KNIME. Understanding these differences can guide users in their tool selection process.
- Cost Considerations: RapidMiner's licensing fees can be significant, especially for small enterprises. In contrast, KNIME offers a more cost-effective solution as it is open-source.
- Customization Limitations: Unlike KNIME, which allows deep customization through its modular architecture, RapidMiner may feel restrictive for advanced users looking to tailor their workflows extensively.
- Performance Bottlenecks: In extensive data environments, some users report performance challenges with RapidMiner, particularly when scaling operations. KNIME often performs better in handling large datasets, thanks to its distributed computing capabilities.
- Learning Curve: While user-friendly, some users report a learning curve, especially when delving into its advanced features. KNIME may provide a more straightforward experience due to its visual programming approach.
Alteryx: Combining Analytics with Data Preparation
Alteryx represents a significant option for businesses seeking to merge data preparation with advanced analytics. In a world where data is vast and varied, organizations need platforms that not only offer analytical prowess but also streamline the data preparation process. Alteryx provides this powerful blend, making it a strong competitor to KNIME.
The importance of focusing on Alteryx lies in its unique capabilities which are critical for decision-makers and IT specialists. By understanding these features, businesses can enhance their analytical process and better align it with corporate goals.
Alteryx's Unique Offerings
Alteryx stands out within the landscape of data analytics due to its user-centric design and functionality. Its key offerings include:
- Self-Service Data Preparation: Users can easily prepare and blend data from various sources without needing extensive coding knowledge. This reduces dependency on IT staff while promoting agility in business processes.
- Robust Analytics Tools: Alteryx provides tools for statistical analysis, predictive modeling, and geospatial analytics. Such features allow businesses to perform complex analyses that are essential for informed decision-making.
- Integration Capabilities: The platform can connect to multiple data sources, such as Excel, SQL databases, and cloud data platforms like Salesforce. This interoperability ensures that users can work with their preferred tools seamlessly.
- Collaboration Features: Alteryx promotes collaboration through its shared workflows and analytics apps. Teams can work together more efficiently, leveraging collective insights across the organization.
These features illustrate why Alteryx captures the attention of many organizations striving for data competency and analytical excellence.
Use Cases and Performance Benchmarks
Alteryx has diverse use cases, particularly in industries such as finance, healthcare, and retail. Here are several notable applications:
- Financial Services: Alteryx assists in risk modeling and financial forecasting, enabling banks to assess potential risks and opportunities effectively.
- Healthcare Analytics: Professionals use Alteryx to analyze patient data and outcomes. This application supports informed decisions in treatment protocols and resource allocation.
- Marketing Analytics: Marketing teams leverage Alteryx for campaign analysis, customer segmentation, and performance tracking, ensuring that strategies are data-driven.
Performance benchmarks show that users experience a significant reduction in time spent on data preparation. Reports indicate that the time needed for data wrangling can decrease by about 60% when utilizing Alteryx’s functionalities. Such efficiency is crucial for organizations that operate in fast-paced environments and require timely insights.
Alteryx's integration of analytics with data preparation provides a compelling argument for companies deciding among analytics platforms. By exploring Alteryx, decision-makers can identify how these elements can improve their data strategy, ensuring they choose the best tools for their operations.
Tableau: Beyond Visualization
Tableau is commonly recognized for its ability to provide powerful data visualizations, yet its role extends far beyond mere aesthetics. This section emphasizes how Tableau integrates seamlessly into the broader data analytics ecosystem, showcasing its capabilities in data integration, analytics, and collaborative work. It is crucial for decision-makers to understand these aspects as they evaluate Tableau against KNIME and other platforms. By leveraging Tableau's advanced features, users can optimize their data workflows significantly, resulting in comprehensive insights that drive business strategies.
Tableau's Data Integration Capabilities
One of the standout characteristics of Tableau is its robust data integration capabilities. It allows users to connect with various data sources including structured and unstructured data from databases, cloud services, and web applications. This includes compatibility with major data storage solutions such as SQL Server, Google BigQuery, and Amazon Redshift.
- Flexible Connectivity: Users can leverage both live connections and data extracts, which makes it easy to manage large datasets efficiently.
- Data Preparation: Tableau offers features that allow for preliminary data cleaning and transformation, although these capabilities are less extensive compared to those found in KNIME.
- Integration with Other Tools: Tableau also supports integration with tools like R and Python through TabPy. This allows analysts to conduct advanced statistical analysis within the platform.
These attributes position Tableau as a practical solution for organizations looking to unify data from diverse sources, promoting enhanced data-driven decision-making processes.
Integration Challenges with KNIME
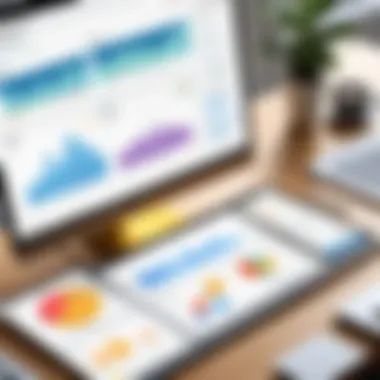
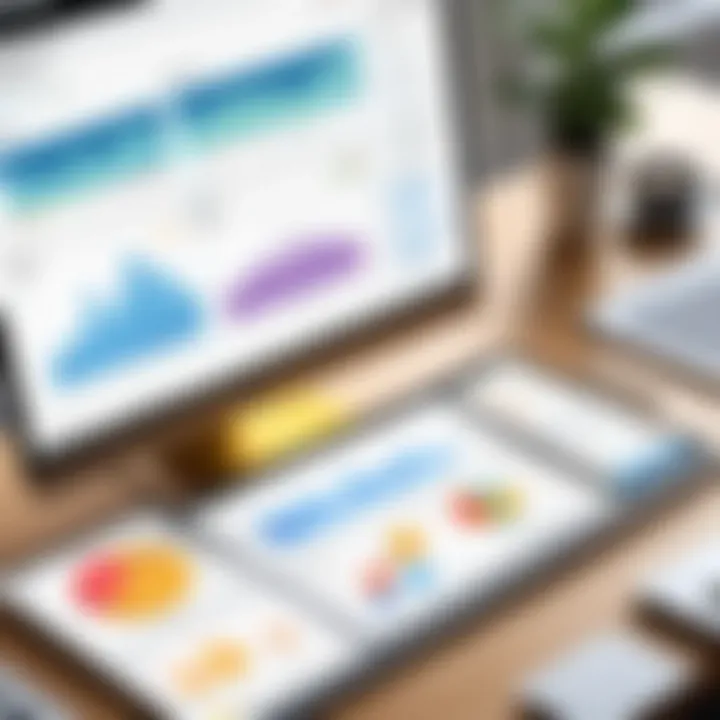
While Tableau offers numerous data integration advantages, users may encounter specific challenges when integrating it with KNIME. These challenges can impact efficiency, especially in complex analytics workflows.
- Data Synchronization: Ensuring that data is consistently synchronized between Tableau and KNIME can be difficult. This synchronization is vital for real-time analytics.
- Complex Workflow Management: Users may find it challenging to maintain an intuitive workflow when combining Tableau’s visualization strength with KNIME’s data preparation capabilities. Effectively managing these workflows requires a thorough understanding of both platforms.
- Licensing and Cost Considerations: Licensing Tableau can also pose a challenge for some organizations. Compared to KNIME, which has an open-source option, Tableau generally requires a subscription, which can strain budgets.
Overall, while both Tableau and KNIME offer exceptional capabilities, aligning their strengths effectively can require strategic planning and additional resources.
Microsoft Power BI: A Business Intelligence Giant
Microsoft Power BI has emerged as a leading tool in the sphere of business intelligence, carving a niche for itself in an environment replete with various data analytics platforms. This section will explore the significance of Power BI in the context of KNIME and its competitors. By showcasing specific elements such as integration, features, and market positioning, this analysis aims to provide insights critical for decision-makers considering analytics solutions for their organizations.
Power BI's Integration with Existing Microsoft Products
One of the prominent advantages of Power BI is its seamless integration with other Microsoft products. Organizations already utilizing Microsoft tools such as Excel and Azure can leverage Power BI effectively. The tight coupling with Excel allows for easy data importation and report generation. Additionally, the integration with Azure services enhances data accessibility and management capabilities.
- Excel Import: Users can pull data from Excel spreadsheet while maintaining the familiar interface.
- Azure Connection: This allows users to analyze cloud data, thereby supporting larger datasets and advanced analytics.
- Teams Collaboration: Power BI reports can be shared directly through Microsoft Teams, enabling collaborative decision-making.
These integrations ultimately contribute to a smoother workflow, enhancing productivity and reducing the learning curve for those familiar with the Microsoft ecosystem.
Assessing Its Impact on KNIME Users
The arrival of Microsoft Power BI into the analytics domain poses certain implications for users of KNIME. Businesses that already adopt Power BI may find a lower threshold for switching to or considering alternatives like KNIME.
- User Experience: The familiar Microsoft interface could lead users to prefer Power BI for ease of use.
- Cost Considerations: Companies utilizing other Microsoft services may find the total cost of ownership for Power BI strikingly favorable, especially when compared to KNIME, which may introduce extra costs depending on workflow complexity.
- Advanced Dashboarding: Power BI provides powerful visualization options and dashboard capabilities, which can potentially overshadow KNIME’s graphical outputs.
However, it is essential to note that KNIME offers a different value proposition focused heavily on data analytics and machine learning. For organizations needing advanced predictive capabilities, KNIME may still hold relevance despite the allure of Power BI.
"The choice between Power BI and KNIME ultimately hinges on the specific analytical needs and existing infrastructure of the organization."
As organizations navigate their analytics strategy, understanding these distinctions will be pivotal. The integration of Power BI with Microsoft products presents a strong case for its adoption, yet KNIME must not be overlooked for its robust analytical capabilities.
SAS Viya: Advanced Analytics Solutions
SAS Viya has emerged as a significant player in the realm of advanced analytics solutions. Understanding its capabilities and how it compares to KNIME is essential for any organization aiming to leverage data for informed decision-making. This section delves into the specifics of SAS Viya, highlighting its strengths, functionalities, and why it should be considered as a viable alternative to KNIME.
Capabilities of SAS Viya
SAS Viya is built on a cloud-native architecture, enabling seamless scalability and deployment. Its capabilities span across various domains, including data preparation, advanced analytics, and machine learning. Organizations can utilize it for complex data analysis, allowing data scientists and business analysts to work collaboratively in a unified environment.
Some key features of SAS Viya include:
- Visual Data Preparation: Users can clean and transform data easily using a drag-and-drop interface.
- Advanced Analytics: Support for AI, machine learning, and deep learning algorithms makes it versatile.
- Integration Capabilities: SAS Viya integrates with multiple data sources efficiently, enhancing its accessibility.
- Real-Time Analytics: Users can perform analytics on streaming data, facilitating real-time insights.
Additionally, its open architecture allows for interoperability with popular programming languages, such as Python and R. This is particularly beneficial for teams that already have expertise in these languages, aligning well with existing skills in organizations.
Comparative Advantages over KNIME
When examining the comparative advantages of SAS Viya over KNIME, several aspects stand out. First, SAS Viya offers a more robust environment specifically designed for cloud computing. While KNIME is effective, especially for data workflow automation, SAS Viya excels in its analytics capabilities.
Here are some considerations:
- Scalability: SAS Viya's cloud-based design allows for greater scalability when handling large datasets compared to KNIME's local installations.
- Performance: In certain scenarios, SAS Viya can achieve faster processing times due to its optimized computing resources.
- Comprehensiveness: SAS offers a more extensive suite of built-in algorithms and tools than KNIME, allowing users to tackle a wider range of analytical challenges.
- Support and Community: SAS has a longstanding reputation with a supportive community and extensive documentation, which can ease the learning curve for new users.
"Organizations looking for a platform that combines advanced analytics with ease of use should closely consider SAS Viya alongside KNIME."
While KNIME provides a strong alternative for workflow automation, SAS Viya’s focus on advanced analytics solutions and its cloud capabilities can make it a more strategic choice for businesses aiming to harness data effectively. Decision-makers should weigh these advantages against their unique needs when considering analytics tools.
Tibco Spotfire: A Comprehensive Platform
Tibco Spotfire stands out in the crowded landscape of data analytics platforms for its multifaceted approach to data analysis and visualization. As businesses increasingly rely on data-driven decisions, the need for robust analytical tools has grown. Spotfire offers a sophisticated yet user-friendly solution that caters to a diverse range of analytical needs. It provides organizations with capabilities that help in not just analyzing data but also in making informed decisions based on actionable insights.
Strengths of Tibco Spotfire
Tibco Spotfire comes with several strengths that make it an attractive option for organizations seeking an analytical platform:
- Advanced Visualization: Spotfire is well-known for its rich and interactive visualizations, allowing users to explore data intuitively. Users can easily create dashboards that communicate insights effectively through attractive graphs and charts.
- Integration Capabilities: Its ability to connect seamlessly with various data sources, including databases, spreadsheets, and cloud services, makes it versatile. Users can pull data from multiple platforms into a single dashboard.
- Real-time Data Analysis: Spotfire supports real-time data analysis which is crucial for businesses that need immediate insights to respond to changing conditions in the market.
- User Customization: Users can customize their dashboards and analytics experiences extensively, enabling tailored insights that cater to specific business needs.
- Collaboration Features: The platform offers strong collaboration tools, allowing teams to share insights easily and work together to solve problems based on data analyses.
Market Position Relative to KNIME
In the context of its market position compared to KNIME, Tibco Spotfire has carved out a significant niche. While KNIME is renowned for its open-source approach focused on data workflows, Spotfire targets organizations that prefer out-of-the-box functionality with a polished user experience.
- Target Audience: Spotfire generally appeals to business leaders who want straightforward analytics without extensive customization. In contrast, KNIME attracts data scientists and IT users who favor a more flexible, code-based environment.
- Deployment and Usability: Tibco Spotfire prioritizes a user-friendly design that can often be deployed quickly, compared to the steeper learning curve associated with KNIME’s extensive capabilities.
- Pricing Model: Spotfire's pricing structure is typically subscription-based, which can be a consideration for organizations mindful of their budget. In comparison, while KNIME is open-source, it may require additional investment for enterprise features.
Ultimately, Tico Spotfire presents distinct advantages that cater to specific user needs. Businesses choosing between the two platforms should carefully consider the specific advantages of each tool relative to their unique operational goals.
Metabase: An Open-Source Solution
Metabase has emerged as a noteworthy contender in the landscape of data analytics platforms. Its open-source nature sets it apart from many proprietary tools, catering to organizations that value flexibility and customization. This section explores the features and benefits of Metabase, enabling decision-makers to consider it as an alternative to KNIME while evaluating their analytical needs.
Metabase's User-Friendliness
One of Metabase's most significant advantages lies in its user-friendliness. Designed with non-technical users in mind, it provides an intuitive interface that simplifies data exploration. Users can easily create visualizations and generate reports without extensive coding knowledge. This democratization of data allows teams across various functions to derive insights without always relying on IT departments.
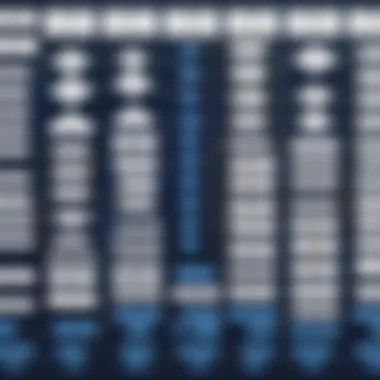
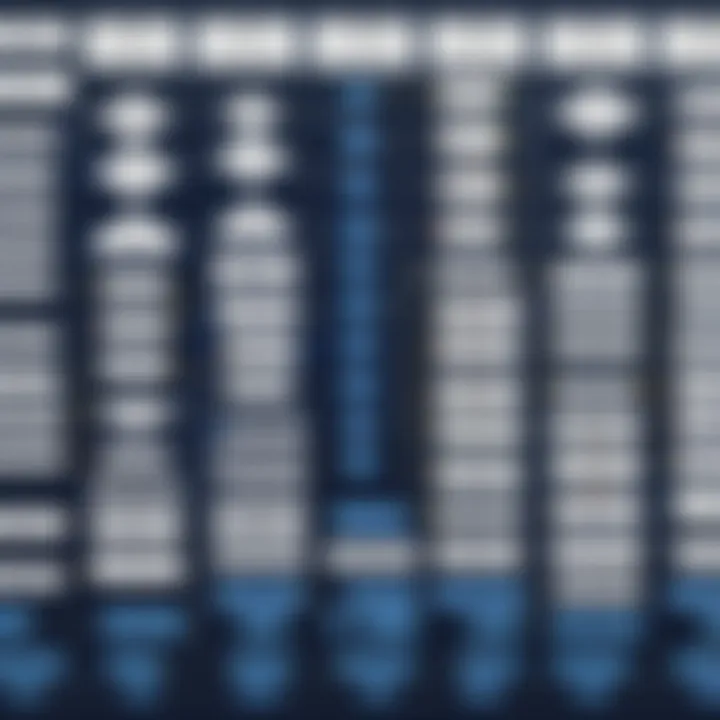
Key aspects of Metabase's user-friendliness include:
- Simple Setup: Installing Metabase can be accomplished with minimal effort, often requiring just a few clicks.
- Custom Dashboards: Users can create customized dashboards tailored to their specific needs, allowing for quick access to essential metrics.
- In-App Guidance: The interface offers helpful tooltips and guidance, making it easy for new users to navigate and utilize the platform effectively.
- Query Builder: Non-technical users can leverage a straightforward query builder to extract data from various sources without SQL expertise.
Overall, these features contribute to a smooth onboarding experience, facilitating a quicker return on investment for organizations that adopt Metabase.
Strengths and Weaknesses Compared to KNIME
When comparing Metabase to KNIME, it is crucial to consider both its strengths and weaknesses. This helps in understanding its fit within an organization's broader analytics strategy.
Strengths of Metabase over KNIME:
- Cost-Effective: As an open-source tool, Metabase can reduce total ownership costs. Organizations can implement it without incurring high licensing fees.
- Speed of Use: Metabase allows users to set up and generate insights relatively quickly, thanks to its straightforward design. In contrast, KNIME may require more time to create complex workflows.
- Quick Visualizations: Users can easily create visualizations in Metabase, helping to communicate findings without extensive preparation.
Weaknesses of Metabase compared to KNIME:
- Limited Advanced Analytics: While Metabase excels in visualizing data, it lacks the robust data processing and modeling capabilities found in KNIME. Users needing advanced analytics may find Metabase insufficient.
- Customization Challenges: Although Metabase is customizable, making extensive alterations to the software may require deeper technical expertise, as its capability limits are more pronounced than those of KNIME.
- Dependency on Data Sources: Metabase relies heavily on the performance and structure of connected data sources, which may hinder performance if those sources are not optimized.
In summary, Metabase offers an appealing set of features for organizations seeking a user-friendly, cost-effective solution for data analytics. However, those requiring advanced data modeling and extensive customization may prefer KNIME or other competitors. The choice ultimately depends on the specific needs of the organization and the expertise of its users.
Considerations for Selecting a KNIME Alternative
Selecting an alternative to KNIME requires thorough contemplation of various factors. This process is crucial for businesses aiming to sustain growth while optimizing data analytics capabilities. Without careful consideration, organizations risk misalignment between their needs and what the chosen tool provides.
Choosing a data analytics platform involves understanding specific business requirements, exploring available features, and assessing how well these fit into current workflows. The right alternative can lead to enhanced productivity, improved data management, and more insightful analytics. Here are vital elements to consider:
- User Requirements: Different teams have unique needs based on their analytical goals. Understanding these requirements is vital for ensuring the selected platform can accommodate different users.
- Feature Specificity: Each tool has its strengths. A keen analysis of features allows businesses to match those strengths with their operational needs or project demands.
- Scalability: It is essential to evaluate whether the alternative can grow with the organization. Scalability ensures that as data needs evolve, the platform can handle increasing volumes and complexities.
- Integration Capabilities: Any data analytics platform must be able to harmonize with existing systems. A seamless integration minimizes disruption during the transition and allows teams to maintain their workflows.
"An informed choice can significantly enhance your analytics capabilities, making it an essential step in leveraging data for strategic advantages."
Evaluating User Needs and Business Goals
When assessing user needs, organizations should first understand what they aim to achieve through a data analytics platform. This starts with gathering feedback from different teams involved in data analysis, such as data scientists, business analysts, and IT professionals. Each group may prioritize different aspects of analytics, like ease of use, advanced analytical capabilities, or specific features like data visualization.
Conducting workshops or surveys can be beneficial in collecting this information. Understanding specific business objectives provides clarity on necessary functionalities. For instance, if real-time data processing is a priority, selecting a platform that excels in this feature is paramount. Aligning user needs with business goals informs a more tailored and effective evaluation process.
Understanding Total Cost of Ownership
Total cost of ownership (TCO) encompasses not only upfront costs but also ongoing costs associated with the software. It is critical to consider various factors that contribute to TCO before selecting a KNIME alternative.
- Licensing Fees: Look at both initial and recurring licensing costs. Different platforms have diverse pricing structures based on functionality and user numbers.
- Operational Costs: Include expenses related to maintenance, training, and support. An alternative requiring extensive training can increase the overall costs considerably.
- Time to Value: Assess how quickly the tool can be deployed and start producing actionable insights. A longer ramp-up period may add significant indirect costs.
Taking the time to calculate the TCO will provide a clearer financial picture and support informed decision-making. Organizations should not only focus on the cost but also weigh these aspects against the benefits the tool offers.
The Future of Data Analytics Platforms
The future of data analytics platforms holds significant importance for businesses aiming to remain competitive in an increasingly data-driven world. As organizations continue to collect vast amounts of data, the ability to effectively analyze that information is crucial. Platforms that can adapt and integrate new technologies will provide organizations the insights they need to make informed decisions. This section explores emerging technologies and innovations shaping the field, as well as predictions about the market's trajectory.
Emerging Technologies and Innovations
Emerging technologies are redefining the landscape of data analytics platforms. Machine learning and artificial intelligence are at the forefront. These technologies enhance predictive analytics, allowing businesses to forecast trends with more accuracy.
With the proliferation of the Internet of Things (IoT), analytics tools now have access to real-time data from various sources. This capability enables organizations to react swiftly to changes in the environment. Another important trend is the rising importance of cloud computing. It offers flexibility, scalability, and as well as cost-effectiveness in handling data analytics tasks.
Some of the key innovations to consider include:
- Automated analytics: Reduces reliance on skilled analysts by applying algorithms that can automatically generate insights.
- Natural Language Processing (NLP): Makes data analytics more accessible by allowing users to query systems using everyday language.
- Data visualization tools: Have evolved, enabling users to create sophisticated visual representations of data, thus making insights easier to understand.
In this rapidly evolving landscape, companies must remain vigilant. Keeping pace with advancements will not only improve analytics capabilities but also enhance organizational efficiency.
Predictions for Market Developments
Looking ahead, several trends are likely to shape the future of data analytics platforms. Greater integration of AI into analytics processes can be expected, resulting in platforms that provide actionable insights faster than ever. Real-time analytics will likely become the norm, enabling companies to harness data as it is generated, which is critical for operational efficiency.
Another prediction is an increased focus on data privacy and security. As regulations become stricter, platforms will need to prioritize data governance and compliance, which in turn will shape their development roadmaps.
Moreover, we might see a shift towards more collaborative analytics environments. Increase in remote work and cross-departmental collaboration may necessitate tools that allow for shared insights and joint data exploration.
In summary, the future of data analytics platforms is intricately tied to technological advancements, market demands, and the evolving needs of businesses. Keeping abreast of these changes will be essential for decision-makers aiming to choose the right analytics solution for their needs and ensuring that their organizations remain competitive.
Ending: Choosing the Right Tool for Your Needs
In today's data-driven world, selecting the appropriate analytics tool is a critical decision for any organization. A wrong choice can lead to inefficiencies, increased costs, and missed opportunities. As discussed throughout this article, KNIME has numerous competitors each offering unique features and capabilities. The importance of this conclusion lies in synthesizing the insights gained from analyzing these alternatives. Decision-makers must understand how each platform aligns with their specific business requirements and objectives. This analysis not only assists in navigating the competitive landscape but also helps in weighing the trade-offs inherent in each option.
Summarizing Competitor Insights
The competitors analyzed in this article—Apache NiFi, RapidMiner, Alteryx, Tableau, Microsoft Power BI, SAS Viya, Tibco Spotfire, and Metabase—each bring distinct advantages and limitations. For example,
- Apache NiFi excels in its dataflow automation capabilities.
- RapidMiner is known for its user-friendly interface designed for rapid prototyping.
- Alteryx stands out for its robust data preparation functionality.
- Tableau is a leader in data visualization yet has integration challenges with KNIME.
- Microsoft Power BI integrates seamlessly with Microsoft products, appealing to existing Microsoft users.
- SAS Viya offers strong advanced analytics capabilities, while Tibco Spotfire provides a comprehensive platform for data analytics.
- Metabase, as an open-source solution, promotes accessibility and ease of use.
Each of these platforms serves distinct business needs. Decision-makers must consider factors such as ease of integration, scalability, user support, and overall costs when evaluating these alternatives.
Final Recommendations for Decision Makers
Given the diversity of options, here are some final recommendations for decision-makers:
- Evaluate Internal Requirements: Organizations should first assess their specific data analytics needs and the skill level of the team that will use the tool.
- Conduct Pilot Tests: Engaging in pilot projects with a couple of selected platforms can provide practical insights into usability and compatibility with existing systems.
- Consider Future Growth: Choose a platform that not only meets current needs but can also scale as data analytics demands evolve.
- Total Cost Analysis: Evaluate not just the purchase price but also maintenance, training, and potential delays in productivity during the transition.
- Engage Stakeholders: Include key users from various departments in the decision-making process to ensure the chosen solution meets cross-functional requirements.
By focusing on these aspects and integrating the insights derived from this article, decision-makers can make informed choices that align with their data strategy and operational goals. As data analytics continues to evolve, staying adaptable and informed will be crucial.