Leveraging Erwin Data Modeler for Superior Data Management
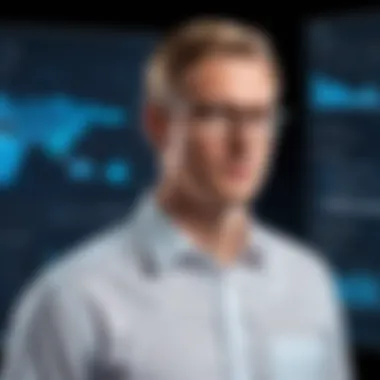
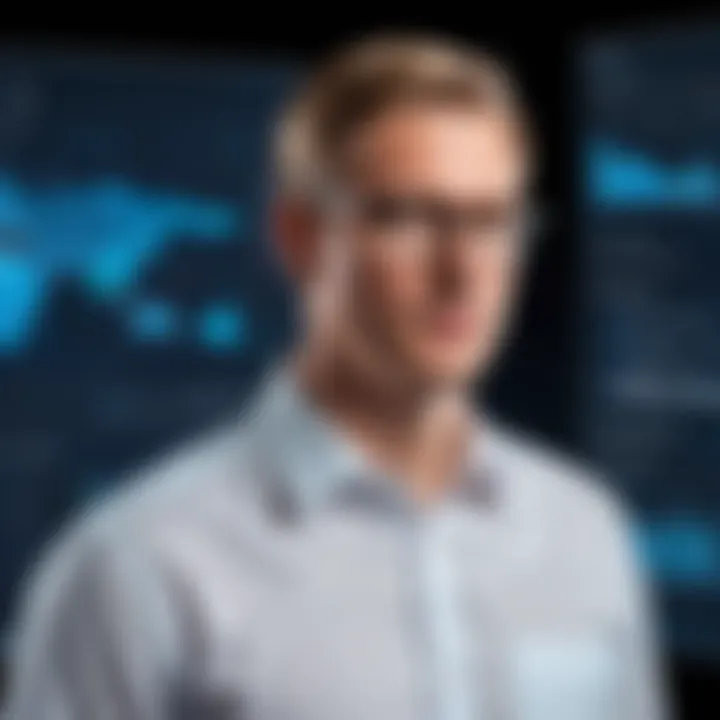
Intro
In the realm of data management, having the right tools can make all the difference. Organizations today face an avalanche of data coming from myriad sources, and the challenge is to manage this data efficiently while maintaining its integrity and usability. Enter Erwin Data Modeler, a robust solution designed to aid professionals in navigating the complex landscape of data architecture. This article probes into the capabilities of Erwin, offering key insights on how to harness its power for effective data management.
Erwin Data Modeler stands at the intersection of functionality and efficiency. It not only caters to the technical requirements of database design but also enhances collaboration among stakeholders. Decision-makers and IT professionals will find value in understanding how this tool can serve as a catalyst for improved data strategy. As we delve deeper into the core features, user experience, and best practices associated with Erwin, it becomes evident that effective data management is no longer a luxury but a necessity in today’s data-centric world.
Overview of Core Features
Description of Essential Functionalities
Erwin Data Modeler boasts a myriad of features geared towards simplifying data management tasks. At its heart, the tool provides a visual interface for designing and managing databases. Users can create data models that represent entities and their relationships. This modeling capability extends to both logical and physical design, allowing for a comprehensive approach to database structuring.
Some notable functionalities include:
- Data Lineage Tracking: Understand where data originates and how it flows through your systems.
- Reverse Engineering: Effortlessly diagram existing databases, gaining insights into current data structures.
- Collaboration Tools: Enable seamless interaction between team members, fostering a culture of transparent communication.
Moreover, the integration of metadata management proves invaluable. It allows users to catalog data definitions and standards, solidifying comprehension across varied teams. This is essential for organizations aspiring to capitalize on their data’s full potential.
Comparison of Features Across Top Software Options
When contemplating the best data modeling software, it’s prudent to benchmark Erwin against its peers in the market. For instance, both Oracle SQL Developer Data Modeler and Microsoft Visio offer alternative approaches but lack some of Erwin's specialized functions like its advanced lineage tracking.
- Erwin Data Modeler: Specializes in data governance features and metadata management.
- Oracle SQL Developer Data Modeler: Less focused on usability, yet strong in database engine compatibility.
- Microsoft Visio: Great for diagramming, but not optimized for complex data architectures.
This comparison encapsulates Erwin's distinctive capabilities effectively. Users seeking a tool that balances complexity with user-friendliness should consider Erwin a front-runner.
User Experience and Interface
Insights into UI/UX Design Aspects
A significant hallmark of Erwin Data Modeler is its intuitive user interface. The layout is purposefully designed to minimize the learning curve for newcomers while offering seasoned users the functionality they expect. The main dashboard conveniently presents tools and options, allowing users to focus on modeling rather than hunting for features.
Visual aids, including drag-and-drop functionalities, simplify the modeling process. This not only enhances productivity but also makes collaboration less cumbersome.
Importance of Usability and Accessibility
In an increasingly remote work landscape, accessibility cannot be overlooked. Erwin facilitates ease of use across various devices, empowering teams to work from different locations while still being connected through a unified platform. This level of usability ensures that even those less tech-savvy can contribute effectively to data modeling efforts.
"The effectiveness of a data model hinges not just on its technical soundness but also on how easily teams can interact with it."
Preamble to Erwin Data Modeler
In the fast-paced world of data management, having the right tools at one’s disposal is essential. Erwin Data Modeler stands out prominently as a critical resource for both businesses and organizations looking to streamline their data architecture. With the ever-increasing volume of data produced daily, understanding the structures and relationships within that data is more vital than ever.
Overview of Data Modeling
Data modeling is the process of creating a visual representation of a data system. This visual aid helps users, particularly IT professionals and decision-makers, grasp how information is structured and flows. It forms the backbone for how databases are built and employed. Utilizing Erwin Data Modeler simplifies this intricate task by enabling users to craft comprehensive models that reflect their data’s intricacies.
- Clarity: Clear diagrams make complex data systems easier to understand.
- Efficiency: Designing schemas virtually reduces time spent on data structuring.
- Standardization: Models ensure consistency in data definitions across an organization.
With Erwin’s powerful capabilities, it offers specialized tools tailored for intricate data mapping. Users can define entities, attributes, and relationships in a user-friendly way. Thus, ensuring that even the less tech-savvy individuals can grasp essential concepts surrounding data architecture. An intuitive design reduces the learning curve, empowering teams to hit the ground running.
Importance of Erwin in Today’s Data Landscape
The relevance of Erwin Data Modeler reflects the ongoing digital transformation that organizations are experiencing. In today's data-driven society, insights gleaned from data are invaluable. Companies are investing heavily in data analytics and management, making effective data modeling crucial to derive actionable insights.
"Effective data management is not just about storing information; it's about turning it into a valuable asset."
Key reasons for embracing Erwin include:
- Integration Capabilities: With its ability to integrate seamlessly with various databases, Erwin ensures compatibility with existing data systems.
- Collaboration Features: The development of data models often requires input from multiple teams. Erwin fosters collaboration, allowing stakeholders to share insights and feedback effectively.
- Adaptability to Change: As the business landscape evolves, so do data requirements. Erwin offers flexibility allowing adjustments and expansions in modeling without significant setbacks.
In essence, utilizing Erwin Data Modeler positions organizations to not only manage their data effectively but to also stay competitive in a landscape that demands swift adaptation to new trends and technologies. By leveraging Erwin, decision-makers unlock the potential of their data, paving the way for informed strategies and business decisions.
Installing Erwin Data Modeler
When diving into data management, installing Erwin Data Modeler is a foundational step that lay the groundwork for effective modeling and analysis. Having the right setup means you're not just plugging in a tool, but gearing up for success in an often complex data landscape. So, let’s break down the essentials and get you started on the right foot.
System Requirements for Installation
Understanding the system requirements is crucial. It's not merely about meeting minimum specifications; it's about ensuring optimal performance. Check your system against the following points to prevent any compatibility hiccups:
- Operating System: Ensure you're running a recent version. Preferably Windows 10 or later, as older systems might not support the latest features.
- RAM: At least 8GB of RAM is recommended. This may seem like a lot, but working with large datasets can strain resources.
- Processor: A dual-core processor will do, but higher performance (like Intel i5 or equivalent) can significantly speed up your tasks.
- Storage: A minimum of 10GB of free space is necessary. Also, using an SSD over a traditional hard drive is advisable for quicker access and loading times.
- Database Connections: If connected to databases, ensure you have the necessary drivers installed for proper communication.
By confirming these requirements ahead of time, you’ll smooth the path for installation and avoid surprises.
Installation Steps and Configuration
Now we can get our hands dirty with the actual installation process. The steps may vary slightly depending on your version, but here's a general guide to steer you through:
- Download the Installer: Head to the Erwin website and fetch the latest version of the Data Modeler. Save it in an easily accessible location.
- Run the Installer: Make sure you right-click and select "Run as administrator" to give it the permissions it needs.
- Follow the Prompts: The installation wizard will guide you; just follow the prompts. Accept the license agreement and choose your preferred installation directory. The default location usually works fine, unless you have specific reasons to change it.
- Select Features: You're often given options about which components to install. While the basic modeling tools should suffice, consider additional modules based on your anticipated use cases.
- Configuration Settings: After installation, it's time to configure settings. Launch the application and set up your workspace. Here, you can choose how you’d like your interface to appear and whether to integrate with existing database connections.
- Finalize Installation: Once configured, save your settings and reboot if prompted. This last step ensures that all changes take effect.
Installing Erwin Data Modeler successfully sets the stage for data excellence. Let’s quote a wise man who once said,
"Don’t start from scratch; start from success."
This couldn't be truer in the world of data management.
With this tool now in your arsenal, heavy lifting on data projects becomes easier, allowing you to focus on what truly matters: crafting meaningful insights from data.
Understanding the User Interface
The user interface of Erwin Data Modeler serves as the gateway through which users interact with the rich features of this data modeling tool. A well-structured interface not only enhances productivity but also makes complex data tasks more approachable for users ranging from novices to seasoned professionals. Understanding how to efficiently navigate and utilize this interface is essential for anyone looking to harness the full power of Erwin.
Navigating the Main Workspace
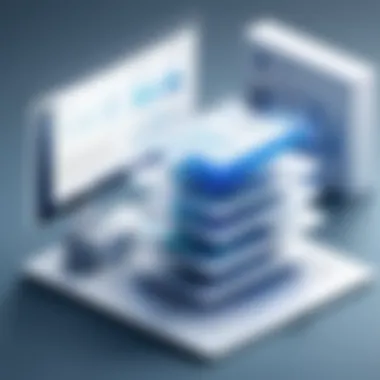
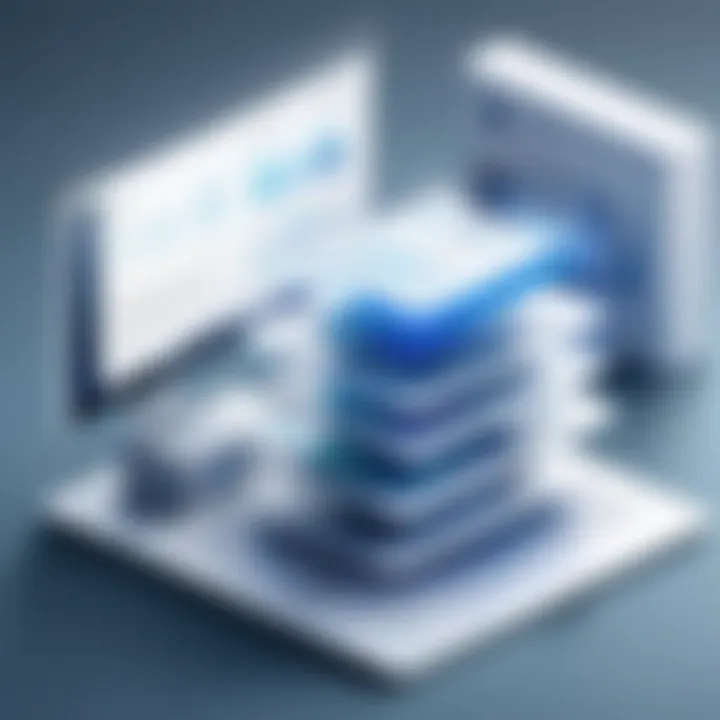
When you first open Erwin Data Modeler, you're greeted by a versatile main workspace that can seem daunting at first glance. However, once you become familiar with its components, you ’ll quickly see how it facilitates effective data management.
- Menus and Toolbars: At the top sits the menu bar, where you’ll find the usual suspects — File, Edit, and View. But don't overlook the specialized toolbars tailored for diagramming, model management, and data operations. Familiarizing yourself with these options is crucial as they directly impact the efficiency of your workflow.
- Diagrams Pane: This is the heart of your modeling experience. Here you can create, edit, and visualize your data models. As you draw entities and establish relationships, the diagrams pane provides a view that fosters understanding from a bird’s eye perspective.
- Property Panel: On the side, you will find the property panel, a pivotal area for editing details regarding the entities and relationships you're defining. Each selection you make in the diagrams pane dynamically updates this panel, allowing for seamless adjustments and additions.
Through consistent practice, navigating these components becomes second nature, paving the way for more fluid modeling processes.
Exploring Tools and Features
Erwin Data Modeler is packed with tools designed to assist in various data modeling tasks. Understanding these tools elevates your modeling capabilities:
- Entity Relationship Tools: The arsenal includes a range of symbols and notations, such as Crow’s foot notation, that simplifies the depiction of relationships between entities.
- Validation Features: One powerful feature is the ability to set validation rules for data integrity, giving your work a safety net against inconsistencies. This can save a truckload of headaches when it comes to data quality later on.
- Reverse Engineering: This feature can pull existing databases into the modeling environment, allowing you to visualize and optimize pre-existing structures, reducing the time spent on building from scratch.
While diving into these tools, take the time to experiment and see how each feature enhances your modeling process. It's like being a chef in a kitchen – the more familiar you are with your tools, the better your dish will turn out.
Customizing the Layout
Customization of the user interface can drastically improve your productivity. Erwin grants users the discretion to tweak the layout in a way that aligns with their individual workflows:
- Panel Arrangement: You can adjust the positions of the toolbars and panels. If you find that the property panel takes up too much space or is hard to reach, you can dock or float it in a way that feels comfortable.
- Theme Selection: Aesthetic choices also play a role. Changing themes can lessen eye strain, particularly during long hours spent modeling. You might prefer a darker interface while others might lean towards a traditional light layout.
- Shortcuts and Hotkeys: Mastering keyboard shortcuts can save precious seconds that add up over time, enhancing your overall efficiency. Configuring hotkeys for frequently used actions enables a fluid experience, allowing for quicker transitions between tasks.
In short, take your time to adjust the layout. A tailored workspace can significantly impact how you perceive and interact with your models.
"The right interface not only gets your work done; it makes you enjoy the ride."
In summary, mastering the user interface of Erwin Data Modeler is a key step to achieving effective data management. By understanding how to navigate, explore, and customize the tools at your disposal, you lay down a solid foundation for successful data modeling projects.
Creating a New Data Model
Creating a new data model is akin to laying the foundation of a skyscraper. Without a solid base, the structure risks instability. This section delves into the intricacies of formulating a data model utilizing Erwin Data Modeler. Proper modeling is key for data consistency, accuracy, and efficiency. It ensures that organizations can derive meaningful analysis and insights from their data. Therefore, understanding how to create these models efficiently is fundamental for IT professionals, decision-makers, and any entrepreneur involved in data management.
Setting Up the Model Environment
The first step in crafting a new data model revolves around setting up the right environment. Here are a few key aspects to consider:
- System Configuration: Make sure your workstation meets the system requirements for the Erwin Data Modeler. Proper setup ensures smoother operations without unnecessary hiccups.
- Project Structure: Create a logical structure for your projects. This can often mirror the organizational framework where, for instance, different departments have separate model segments.
- Data Standards: Establish data standards early on. This includes naming conventions, formatting, and guidelines for what types of data entries are allowed.
Setting up the environment effectively not only improves workflow but also lays a strong groundwork for future model enhancements.
Defining Entities and Attributes
Once the model environment is established, the next big leap is defining entities and attributes. Think of entities as key players in your model, the main characters in your story. Each entity represents a distinct object or concept, such as Customer or Product. Attributes, on the other hand, serve as descriptive elements that provide further context about these entities.
- Identify Core Entities: It’s crucial to recognize which entities are pivotal to your business operation.
- List Attributes Clearly: For each entity identified, outline relevant attributes. For instance, a Customer entity may need attributes like Name, Contact Information, and Purchase History.
- Utilize Data Types: Assign appropriate data types to each attribute. This not only defines the kind of data allowed but also influences data integrity down the road.
By taking the time to define entities and attributes carefully, you assure that the model reflects your business logic accurately and can be enhanced as your needs evolve.
Establishing Relationships
The final layer of creating your new data model is establishing relationships among entities. This aspect might seem straightforward, but it’s crucial to understand how different elements interact. In data modeling, relationships illustrate how entities relate to one another.
- Types of Relationships: Recognize that relationships can be one-to-one, one-to-many, or many-to-many. For example, one customer can place many orders, indicating a one-to-many relationship with the Orders entity.
- Cardinality and Participation: Clearly define the cardinality (how many instances of one entity relate to another) and the participation (mandatory or optional) for each relationship. This detail helps guide future data handling and queries.
- Visual Representation: Erwin Data Modeler provides tools to graphically illustrate these relationships, ensuring clarity. Utilizing diagrams helps stakeholders instantly grasp the model's design and its implications.
Establishing these relationships is vital for data integrity and navigation; it dictates how users of the system will interact with the data at hand.
"In data modeling, clarity is king. A well-defined relational structure paves the way for optimized data retrieval and reporting.”
In summary, the process of creating a new data model within Erwin Data Modeler is not merely a technical task; it’s an essential aspect that shapes how organizations leverage data for strategic decisions. With a well-set environment, clear entities with well-defined attributes, and carefully established relationships, the path to effective data management is not just a goal but a reachable reality.
Advanced Data Modeling Techniques
In the fast-paced world of data management, mastering advanced data modeling techniques is crucial for creating robust data architectures. These techniques not only enhance the quality of your models but can also significantly improve decision-making processes within an organization. Here, we'll highlight a few essential methods that can help you leverage Erwin Data Modeler to its fullest potential.
Incorporating Business Rules
Business rules serve as essential guidelines that govern the operation and behavior of systems and processes within an organization. Integrating these rules into data models is not simply an academic exercise; it holds real-world value. They ensure that the data design aligns with business objectives, enhancing data integrity and relevance.
For example, if your organization operates under specific regulatory requirements, embedding these rules directly into your data model can help ensure compliance right from the ground up.
- Benefits of Business Rules Integration:
- Increased Consistency: By establishing standardized rules, you can minimize the chances of discrepancies that might arise from various interpretations of requirements.
- Enhanced Communication: Clear business rules communicate the expected outcomes of certain conditions, simplifying discussions among stakeholders.
- Adaptability: Modifying business rules within the model can be done without significant additional effort, making the system more flexible to changes.
As the saying goes, "A stitch in time saves nine." Addressing business rules early can save a lot of headaches later.
Utilizing Reverse Engineering
Reverse engineering is a technique that allows you to gain insight into an existing database model. With Erwin Data Modeler, this process can transform an unclear schema into a detailed data model that is comprehensible and documented. Why is this important? Well, many organizations find themselves dealing with legacy systems that lack proper documentation or are poorly understood. By reverse engineering these systems, professionals can:
- Identify Data Relationships: Reveal how entities link together in the database, making it easier to understand the flow of information.
- Improve Migration Processes: Smoothly transition to new software systems by outlining the existing structure and addressing potential pitfalls before they arise.
- Reinforce Legacy Understanding: Build a foundation of knowledge that helps bridge the gap between old and new systems, ensuring that vital information is not lost in transition.
Implementing Version Control
Version control is often overlooked when discussing data modeling, yet its inclusion can vastly improve the management of data models. It provides a mechanism for tracking changes and maintaining historical records. Without it, one wrong change can lead to confusion or worse—data loss. Here's how Erwin simplifies this:
- Controlled Collaboration: Multiple team members can work on different aspects of the data model without fear of overwriting each other's contributions. This is especially useful in larger teams.
- Change Tracking: Keep tabs on who made each change, what alterations were made, and when they occurred.
- Rollback Capabilities: Should an error slip through the cracks, version control allows for quick restoration of earlier functional states.
Utilizing these advanced techniques in Erwin Data Modeler dramatically enhances your data strategies. Embracing business rules, reverse engineering, and version control can set your organization on a path to greater efficiency and improved outcomes. Understanding and applying these techniques not only contributes to individual project success but also fosters a culture of continuous improvement within the organization.
Data Validation Techniques
Data validation is a cornerstone in the realm of data management. It ensures that the data created within a model is accurate, complete, and meaningful. When utilizing Erwin Data Modeler, data validation becomes crucial as it safeguards the integrity of your data, minimizing errors that can cascade through analyses and decision-making processes.
When you implement effective data validation techniques, you not only enhance the reliability of your models but also foster confidence among stakeholders. Without proper validation, the consequences can be dire, leading to faulty insights and misguided strategies. Thus, grasping the methodologies of data validation is paramount for IT professionals and decision-makers who rely on high-quality data for informed decision-making.
Conducting Data Quality Checks
Conducting data quality checks involves a thorough examination of the data to gauge its accuracy and consistency. This process typically includes scrutiny of various data attributes, such as data types, ranges, and format consistency. Imagine you're the captain of a ship navigating through stormy seas; if your charts are faulty, your journey may quickly come to an end.
Here are a few key steps in conducting effective data quality checks:
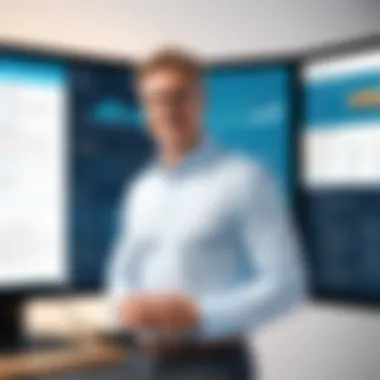
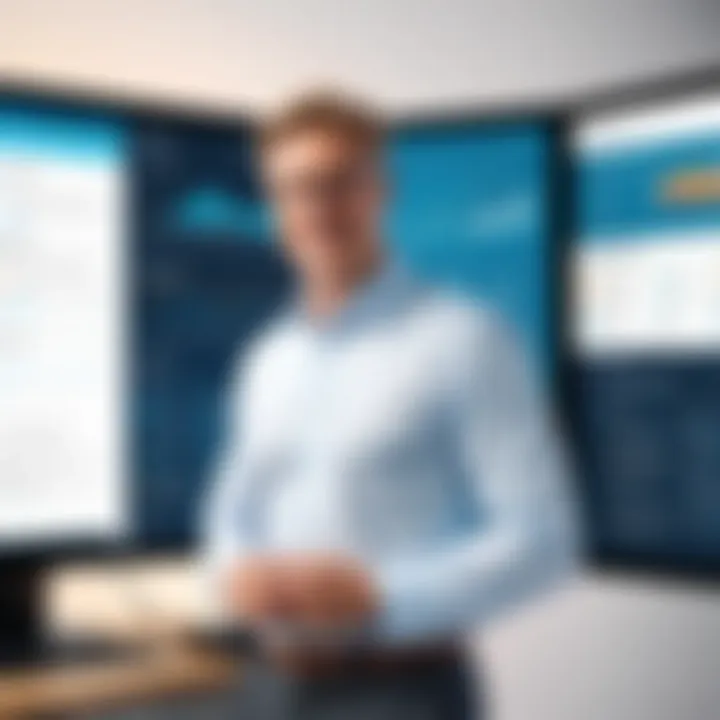
- Define Quality Criteria: Set clear standards for what constitutes high-quality data in your specific context.
- Use Profiling Tools: Utilize Erwin’s profiling capabilities to analyze existing data and reveal anomalies that need addressing.
- Perform Consistency Checks: Look for discrepancies within the data set. Ensure that nothing contradicts established norms.
- Automate Where Possible: Take advantage of automation for regular checks, allowing staff to focus on analysis rather than manual verification.
Using Validation Rules
Validation rules are the guiding principles that help maintain the data’s quality throughout its lifecycle. By applying these rules within your Erwin Data Modeler, you create a robust framework to prevent invalid data entries from slipping through the cracks.
To effectively use validation rules, consider the following:
- Specify Data Formats: Rules can enforce specific formats for data entries. For instance, validating date formats as YYYY-MM-DD prevents confusion.
- Set Range Limits: For numerical values, ensure the data only falls within a predetermined range. This avoids absurd values that skew analyses.
- Implement Referential Integrity: When establishing relationships between different data entities, ensure that references are valid and enforceable.
- Testing: Regularly review and test your validation rules for effectiveness. If a rule is too stringent, it can hinder necessary data inputs; too lenient, and it risks introducing errors.
"Good data is a treasure; poor data is a curse. Validating data is akin to having a map while trekking through uncharted territory."
Incorporating these data validation techniques not only streamlines the modeling process but also fortifies the resulting data structures, enabling clearer insights and more accurate predictions. The efficacy of any data-driven strategy hinges on the integrity of the data it utilizes, making validation an essential aspect of any data management initiative.
Documenting the Data Model
In the realm of data management, documentation serves as the bedrock upon which successful projects are built. Think of documenting the data model as creating the roadmap that guides users through the complexities of their data landscape. This aspect often gets overshadowed by the glamour of data analysis. Yet, it’s in the documentation that clarity emerges. It not only aids in understanding the structure and usage of datasets but also facilitates communication amongst team members, stakeholders, and future users.
When a data model is well-documented, it outlines the entities, attributes, relationships, and rules governing the data. This forms a detailed map that can help in troubleshooting, onboarding new team members, and ensuring regulatory compliance. Plus, it enhances the transferability of knowledge, which is vital in today’s fast-changing environments.
Undoubtedly, the benefits of effective documentation are numerous:
- Eases Maintenance: A clear documentation helps in quickly identifying areas requiring updates or fixes.
- Improves Collaboration: Everyone on the team, regardless of experience level, can understand the data model, reducing bottlenecks in communication.
- Promotes Consistency: It ensures that all team members adhere to the same definitions and guidelines, which mitigates misunderstandings.
- Facilitates Compliance: It serves as evidence that the organization takes measures to manage data responsibly, which is increasingly crucial in data governance.
As we proceed, it’s essential to look closely at how to create this documentation efficiently.
Generating Documentation Automatically
One of the significant features of Erwin Data Modeler is its ability to generate documentation automatically, saving both time and effort. This functionality is a breeze to use and allows for creating up-to-date reports that encapsulate the complete analysis of a data model.
Using Erwin, you can automatically produce:
- Data Dictionary: A comprehensive listing of all entities and their attributes, which can prove invaluable when aligning common terminologies across the board.
- Entity-Relationship Diagrams: Illustrative diagrams that showcase how the entities interact, which can help visualize complex relationships at a glance.
- Change Logs: Records of modifications made over time, which help keep all parties informed about the evolution of the model.
This automation is not just about convenience; it’s also about accuracy. Manual documentation can introduce human error, whereas automated outputs reduce this risk significantly.
Best Practices for Effective Documentation
Documenting data models is not merely a task to tick off a list; it’s an ongoing process requiring attention and diligence. Here are some best practices to consider:
- Be Consistent: Ensure that terminology and formatting are uniform throughout the documentation. This makes it easier to follow and interpret.
- Update Regularly: Data models evolve, and so should their documentation. Regular updates post-modifications ensure that insights remain relevant.
- Engage Stakeholders: When drafting the documentation, involve key stakeholders to gather their insights and ensure it meets their needs. Diverse input can greatly enhance the comprehensiveness of the documentation.
- Leverage Visual Aids: Utilize charts, diagrams, and graphs where applicable. Visual representations often convey complex ideas more effectively than text alone.
- Focus on Clarity: Write in a straightforward manner, avoiding jargon unless it's commonly understood within the context. This reduces ambiguity, ensuring that everyone can grasp the details.
In summary, effective documentation is not just an accessory to data modeling; it is a crucial component that can significantly enhance the usability and longevity of data assets. By adopting automated tools and adhering to best practices, organizations can solidify their data management frameworks.
Collaboration Features in Erwin
Collaboration is the keystone of successful data management. In environments where decisions pivot on accurate data modeling, it’s crucial that teams work in tandem, sharing insights effortlessly. Erwin Data Modeler’s collaborative features are designed not just to support teamwork; they elevate it to a new level. Let’s delve into how these functionalities can benefit organizations, empowering users to streamline processes and ensure everyone is on the same page.
Sharing Models with Stakeholders
One of the standout features of Erwin is its capacity to share data models with stakeholders in a seamless manner. This capability is indispensable for professionals looking to foster transparency and encourage collaborative dialogue among project participants. By enabling team members to view and interact with models directly, Erwin reduces the back-and-forth that often plagues project management.
Using the sharing features, users can:
- Present Models in Diverse Formats: Stakeholders often have varying preferences for how they receive information. Erwin accommodates this by allowing models to be shared in different formats, including PDF and HTML, making it easier for everyone to access the necessary details.
- Collaborative Feedback Loops: Stakeholders can provide real-time feedback directly on the models. This feature not only saves time but also ensures that vital inputs are integrated promptly, paving the way for a more agile development cycle.
- Accessible Across Locations: With cloud integration capabilities, users can share models with anyone, anywhere. This is particularly beneficial for organizations with remote teams, fostering a sense of unity even when working from different corners of the globe.
In short, sharing models effectively transforms how teams interact with data, reducing friction and enhancing productivity.
Version Control and Change Tracking
Managing change is a vital part of data modeling, and Erwin excels in providing robust version control and change tracking features. These capabilities serve as a safeguard against potential mishaps that can arise during the modeling process, ensuring the integrity of data is maintained as projects evolve.
Key aspects of version control in Erwin include:
- Historical Tracking: Every change to a model is recorded, allowing users to revisit previous versions if needed. This is particularly useful when a recent modification raises questions or issues that require reverting to a prior state.
- Collaborative Editing: Multiple users can work on the same model, and Erwin efficiently keeps track of changes made by each individual. This multilingual aspect means no one is left in the dark; everyone knows what has been altered and who made the changes.
- Audit Trails: Comprehensive logs help in audits, giving organizations clarity in their modeling processes. This kind of transparency is invaluable for compliance and regulatory requirements, as it documents all decisions made along the way.
In essence, version control not only enhances accountability but also mitigates risks associated with collaborative data modeling, making it a critical feature for teams relying on Erwin.
By utilizing Erwin's collaboration features, teams can bridge gaps between stakeholders and data models seamlessly, ensuring collective growth and performance in data management.
Integrating Erwin Data Modeler with Other Tools
The significance of integrating Erwin Data Modeler with other tools cannot be overstated. In today’s landscape where data is both a valuable asset and a challenging resource to manage, the ability to seamlessly connect multiple platforms facilitates better data handling and utilization. If you think about it, just as a carpenter wouldn’t use only one tool to build a cabinet, data professionals need a suite of tools working in harmony to create robust models and architectures.
Data Source Integration
When it comes to data source integration, the capacity to link various data repositories effectively means you’re opening up a treasure chest of insights. Erwin Data Modeler has built-in connectors that allow it to interface with databases, cloud storage solutions, and other data sources. This is crucial because it enables users to pull real-time data rather than relying on static snapshots.
How can you make the most of this? For starters, ensure that your data sources are clearly defined within the model. Often times organizations face hiccups due to unclear data lineage.
- Here’s a quick guide to integrating data sources:
- Identify the data sources: Know where your data lives (e.g., SQL databases, NoSQL solutions, Excel files).
- Map out connections: Draw a clear picture of how data from various sources will flow into Erwin.
- Test the integration: Ensure that data pulls happen smoothly by performing some test runs.
For professionals keen on minimizing fragmentation and maximizing data fidelity, integrating sources is akin to ensuring that all cogs in a machine are well-oiled and turning together.
Exporting Models to Various Formats
Exporting models is another pillar of effective data management in Erwin. The ability to share your models in various formats can lead to a more collaborative ecosystem. Whether it's exporting to PDF for easy sharing or single-click options to HTML for online access, being adaptable with your output significantly enhances communication among stakeholders.
So, what should you keep top of mind when exporting?
- Choose the right format: Depending on your audience, some formats may be more useful than others. Technical teams might appreciate XML, while executives likely prefer a polished PDF format.
- Maintain clarity and accuracy: Document everything well. Miscommunication due to poorly exported models can lead to misunderstandings down the line.
- Automate regularly: Set up automated exports if you frequently share models, making it part of your workflow.
As with data source integration, exporting isn't merely about pushing a button; it's about ensuring that the resultant output aligns with the needs of the audience, ensuring clarity in the communication of complex data structures.
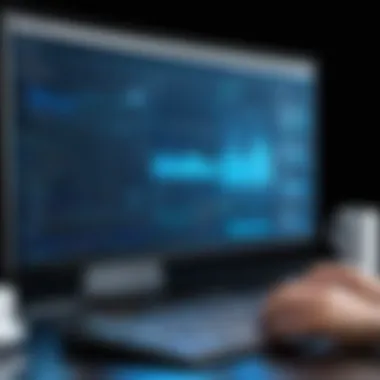
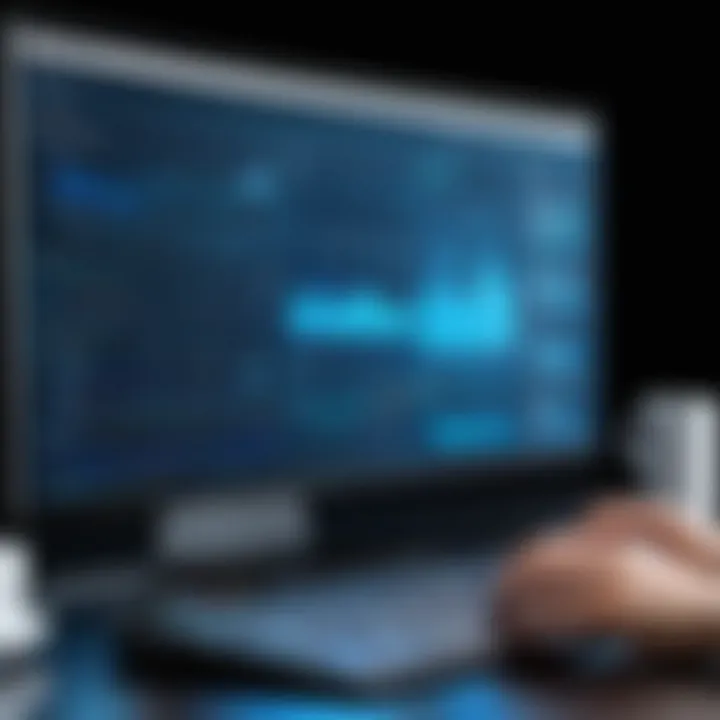
"The integration of tools within your data environment isn't just a convenience; it's a necessity for operational efficiency and better decision-making."
By tying together various tools with Erwin Data Modeler, you can harness an ecosystem that not only improves your data management strategy but also promotes a culture of collaboration within your teams.
Common Challenges and Solutions
In any data management endeavor, challenges are often a part of the journey. When working with Erwin Data Modeler, understanding these challenges and their solutions can make the difference between a smooth operation and a frustrating experience. This section explores common issues users face, along with practical solutions that can lead to efficient data modeling.
Troubleshooting Model Issues
Every user of Erwin Data Modeler has likely encountered a range of model-related hurdles. These issues can stem from various sources, such as corrupted files, missing relationships, or even improperly configured settings. Effective troubleshooting involves a systematic approach:
- File Integrity: Regularly check the integrity of your model files. Sometimes, you might find that saving or importing a file can result in corruption. Keeping a backup of versions can really save the day.
- Validating Relationships: Ensure that relationships between entities are correctly established. For instance, if an entity appears in a model but doesn't relate to others, it might lead to confusion down the line. Running integrity checks built into Erwin can help identify these problems.
- Configuration Settings: Sometimes, issues arise from configuration settings not being applied consistently. Double-check that your settings align with the project requirements. It's easy to miss a tick box or an option when setting things up.
By identifying root causes and applying techniques to counteract them, users can smooth out the bumps that pop up in their modeling processes.
Avoiding Common Pitfalls
Potential pitfalls are often the result of negligence or a genuine misunderstanding of the tools at hand. Here are some common ones:
- Overcomplicating Models: A frequent misstep is making models more complex than necessary. An overly complicated model can lead to confusion both in the design phase and later when it comes time to implement. Strive for clarity and simplicity, as a straightforward design tends to be more effective.
- Neglecting Documentation: While it may seem tedious, documenting every change and decision made during the modeling process is crucial. Lack of documentation can lead to ambiguities, especially when bringing in new team members or revisiting projects after some time has passed.
- Ignoring User Feedback: Engaging other stakeholders during the development phase is key. They might provide different perspectives that can highlight blind spots or areas for improvement that may not be apparent to the modeling team.
By being aware of these pitfalls, users can proactively manage their modeling practices, ensuring that the end output is clear and reliable.
"Awareness of common challenges helps you navigate the data modeling landscape with greater confidence and clarity."
Implementing these troubleshooting techniques and avoiding common pitfalls not only enhances the quality of data models but also contributes to smoother workflows and encourages a culture of continuous learning within teams.
Evaluating the Output of Models
In the realm of data modeling, evaluating the output of models is a critical step that often determines the success or failure of data management initiatives. This process involves scrutinizing how well the model aligns with business needs, its ability to convey meaningful insights, and its overall performance in a real-world context. Without thorough evaluation, there is a risk of misinterpretation, which can lead to flawed decision-making and inefficiencies. The stakes are high; after all, data drives decisions, and poor models can spell disaster for stakeholders.
Interpreting Model Diagrams
Model diagrams serve as the visual representation of data structures and relationships. They are the backbone of any data model, providing clarity on how entities relate to one another. However, interpreting these diagrams is not merely about understanding where the boxes and arrows point. It requires a keen eye to see the bigger picture—the logic behind the design.
When stakeholders look at a data model diagram, they should focus on critical aspects such as:
- Entity Relationships: Understanding how entities interact is vital. For instance, a product may be linked to customer data, reflecting how sales data affect inventory levels.
- Cardinality: This information helps define how many instances of one entity can be associated with another. Misreading it can lead to improper assumptions about capacity and resources.
- Attributes: Each entity comes with its characteristics. Recognizing which attributes are essential and which are not can streamline processes and improve data governance.
Consequently, when interpreting these diagrams, it’s crucial to ask questions like: Are the relationships logical? Do the attributes convey the necessary information? Such inquiries help refine the model and ensure it meets the specified requirements.
Analyzing Performance Metrics
With models created and diagrams drawn, the next step involves analyzing performance metrics. This process allows one to assess how effectively the model functions in practice. Performance metrics can range from how quickly data can be retrieved to how accurately it matches expected outcomes. Here are a few key areas to consider during this phase:
- Response Time: How fast can the system retrieve data based on the model? Delays can cripple data-driven projects.
- Accuracy: Does the model produce reliable output? It’s essential to cross-check model predictions with real-world data.
- Scalability: As the organization grows, can the model handle increased loads? Future-proofing is often overlooked but is necessary for sustainable growth.
*"Without metrics, you're just guessing."
To effectively analyze these performance metrics, one must leverage appropriate tools and techniques that can extract meaningful insights from data outputs. Further, establishing baseline metrics from the get-go allows for proper comparisons and evaluations later on. By frequently reviewing performance, stakeholders can make timely adjustments to improve both the modeling process and its outcomes.
Best Practices for Using Erwin Data Modeler
When it comes down to utilizing Erwin Data Modeler effectively, establishing a set of best practices can make all the difference. These practices not only enhance the overall efficiency of data management but also contribute to a smoother workflow, ultimately leading to more robust data architectures. Adhering to these guidelines encourages informed decision-making and strategic insights, which can be vital for professionals handling vast amounts of data.
Regular Updates and Maintenance
Keeping Erwin Data Modeler updated is akin to giving it a bite of the proverbial apple—it's essential. Regular updates ensure that you’re not only utilizing the latest features but also benefitting from important bug fixes and performance improvements. Without updates, your data models may miss the mark in terms of accuracy and functionality.
Conducting regular maintenance is another piece of the puzzle.* It’s important to periodically check the settings, configurations, and overall setup of the software. *
- Backup your models: Make it a routine to save copies of your data models. This acts as a safety net against unforeseen data losses.
- Review and clean up old data: Outdated models can clutter your workspace and skew results. Remove or update what’s no longer relevant.
- Verify integration functionalities: Ensure your connections with other tools or databases are seamless and functioning as intended.
Regular tinkering—adjusting settings, refreshing integrations—can save you from future headaches. Remember, a stitch in time saves nine.
Training and Continuing Education
The world of data management isn't static; it's always evolving. Therefore, investing in training and continuing education should be on every professional's radar. It's one thing to know how to navigate Erwin Data Modeler; it’s another to understand its depth and nuances.
Regular workshops or training sessions can empower your team with updated knowledge on the software's features, data management concepts, and industry best practices.
Key benefits of ongoing training include:
- Enhanced Knowledge Base: Keeping yourself and your team abreast of changes allows for quicker adaptation and better utilization of functionalities.
- Increased Productivity: Familiarity with the software leads to streamlined workflows, reducing errors and time spent on model creation.
- Network Opportunities: Engaging in training often opens doors for connecting with other professionals in the field, fostering collaboration.
"An investment in knowledge always pays the best interest." - Benjamin Franklin
Staying educated not only helps in fostering a culture of learning but also encourages innovation within your organization. Whether you choose to invest in workshops, online courses, or even knowledge-sharing sessions among peers, continuously enhancing your expertise ensures that your utilization of Erwin Data Modeler remains cutting edge.
The Future of Data Modeling with Erwin
As businesses continue to evolve in the face of an ever-changing technological landscape, the future of data modeling holds immense implications for organizations seeking agility and insight. The Erwin Data Modeler has positioned itself as an essential tool in the ongoing journey towards effective data management. Recognizing this importance allows decision-makers to leverage Erwin not just as a tool, but as a strategic asset for their business objectives.
Emerging Trends in Data Management
In the dynamic field of data management, several trends have begun to shape the ways that organizations handle their data ecosystems. Staying ahead of these trends is vital for IT professionals as they strive to harness the potential of data effectively. Some significant trends include:
- Data Democratization: More businesses are empowering non-technical staff to access and analyze data. This shift encourages innovation and agility, challenging a once strictly siloed approach.
- AI and Automation: Artificial intelligence is increasingly integrated into data modeling processes. Automation tools enhance productivity and accuracy, allowing teams to focus on high-level strategy rather than manual tasks.
- Cloud Adoption: As companies migrate their operations to the cloud, the need for robust data modeling solutions that can seamlessly interact with cloud architectures becomes paramount.
- Data Governance and Compliance: With regulations tightening, maintaining a strong data governance framework is now essential for compliance and quality assurance.
Erwin Data Modeler is prepared to tackle these emerging trends by enabling organizations to adjust quickly to changing landscapes. The tool’s adaptability ensures that it remains relevant, catering to these evolving needs.
Innovative Features on the Horizon
Looking ahead, Erwin Data Modeler is anticipated to roll out a series of innovative features aimed at enhancing its capability as a powerful data management solution. Some of the potential new features could include:
- Improved AI Integration: Advanced AI capabilities may offer insights into data patterns, making it easier to predict and respond to changes in business environments.
- Real-time Collaboration Tools: Features that facilitate seamless coordination amongst teams, regardless of location, would enhance productivity and knowledge-sharing.
- Expanded Data Source Connectivity: Future updates might include the ability to connect to more diverse data sources, streamlining the integration of data from various environments.
- Enhanced Visualization Tools: Greater visualization features will allow users to transform complex data sets into accessible insights, crucial for effective decision-making.
By adopting such innovative features, Erwin Data Modeler aims to empower organizations in maximizing their data potential and driving informed business decisions.
"In the world of data, staying ahead is not just beneficial; it's essential. Organizations must evolve along with their data tools to ensure they remain competitive."
The future of data modeling with Erwin is not just about keeping pace with technological advancements. It’s also about understanding that data is a vital resource that, when managed properly, fuels growth and innovation. As the landscape becomes increasingly complex, Erwin’s continual improvements and feature expansions will serve as a cornerstone for effective data management strategies.