Navigating Decision Support System Software Guide
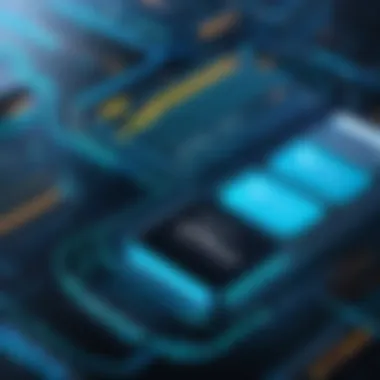
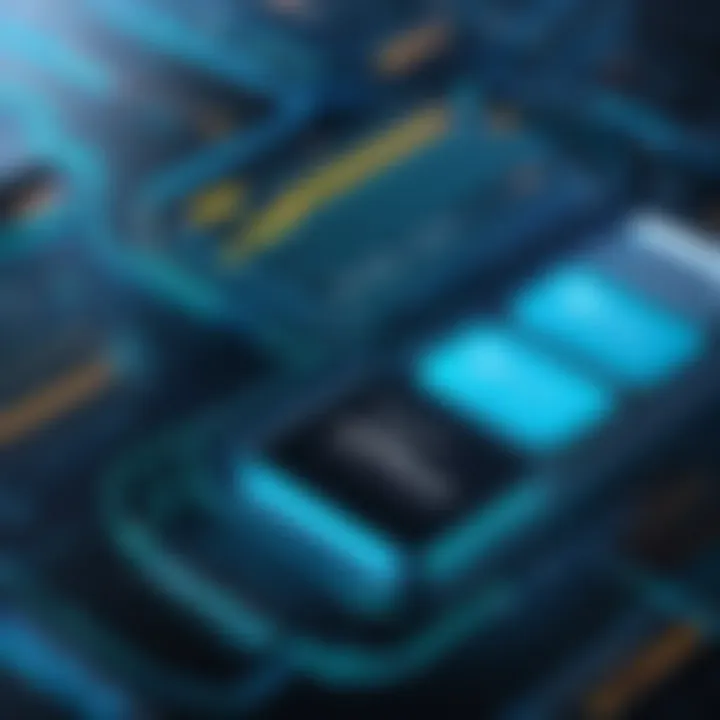
Intro
In today's fast-paced world, the need for effective decision-making tools has never been more critical. Organizations across various sectors are constantly bombarded with data, and making sense of it all can feel like trying to find a needle in a haystack. Decision Support System (DSS) software is designed to aid in slicing through the noise, providing valuable insights that can guide management and operational decisions.
By offering structured analysis and data visualization, DSS software can be a game-changer. Whether it's peering into market trends, overseeing resource allocation, or optimizing business processes, these tools are mounted at the frontline of strategic decision-making. This article will serve as a guide, shedding light on the essential components of DSS software, the best options available, and how to seamlessly integrate them into your organization.
Overview of Core Features
When delving into the realm of Decision Support System software, it's essential to grasp the core features that define these technologies. Here, we will break down those essential functionalities that every decision-maker should consider when evaluating options.
Description of Essential Functionalities
DSS software offers a variety of functionalities designed to support the decision-making process:
- Data Management: The heart of any DSS is its ability to collect, store, and manipulate data from various sources, including databases and data warehouses. This feature ensures that decision-makers have access to relevant and up-to-date information.
- Model Management: DSS allows users to create and use models for analyzing complex data. These models can simulate different scenarios, providing a clearer view of potential outcomes.
- User Interface: An intuitive user interface is paramount. It enables users, regardless of technical expertise, to navigate the software efficiently.
- Report Generation: Automated reporting tools can help in showing findings and insights. This functionality not only saves time but also enhances clarity in communication.
- Visualization Tools: Graphical representations of data are vital for understanding complex information quickly. Charts, graphs, and dashboards can transform raw data into actionable intelligence.
Comparison of Features Across Top Software Options
Not all DSS solutions are created equal; differences in functionality can significantly impact decision-making quality. Below is a comparison of some leading software options:
| Software Name | Data Management | Model Management | Reporting Tools | Visualization Tools | | Tableau | Excellent | Limited | Strong | Superior | | Microsoft Power BI | Good | Moderate | Excellent | Very Good | | IBM Planning Analytics| Moderate | Excellent | Good | Good | | SAS Decision Manager | Excellent | Good | Very Good | Excellent |
This comparison reveals distinct strengths and weaknesses of each software. Depending on your organizationâs needs, certain features may stand out more than others.
User Experience and Interface
The effectiveness of a DSS isnât solely determined by its functionalities. The user experience (UX) and user interface (UI) are just as important. A well-designed interface can enhance user engagement and lead to more effective decision-making.
Insights into UI/UX Design Aspects
The design aspects should not be overlooked. Ideally, DSS software should feature:
- Clarity: Information should be clearly presented without any unnecessary clutter.
- Responsiveness: Users should be able to navigate through the software quickly without delays.
- Customization: The ability for users to tailor dashboards and reports according to their preferences enhances usability.
Importance of Usability and Accessibility
In a world where time is money, the usability of DSS software can make all the difference. Employees must quickly grasp how to use the tools at their disposal. Furthermore, making software accessible to a broader audience, including those with disabilities, cannot be understressed.
"The right decision support system is worth its weight in gold; it is not just about data, itâs about the insight you glean from it."
As organizations continue to operate in increasingly complex environments, selecting the appropriate DSS software becomes a crucial task. In the subsequent sections, weâll further explore implementation strategies, challenges, and emerging trends in the field.
Understanding Decision Support Systems
The realm of decision support systems (DSS) stands as a crucial pillar in strategic decision-making across various industries. Gathering, analyzing, and interpreting vast amounts of data can be overwhelming, yet these systems simplify that complexity. Instead of diving into a ocean of raw numbers, decision-makers can leverage purposeful insights that aid in reaching firm and confident conclusions. Grasping the fundamentals of decision support systems sets the stage for exploiting their full potential, thereby leading to improved organizational performance.
Definition and Purpose
A decision support system is designed to assist in judgment and decision-making processes. It provides a comprehensive platform that integrates data, complex calculations, and analytical models to offer actionable intelligence. Simply put, a DSS acts as an intelligent partner that crunches the numbers and provides clear, interpretable recommendations for various scenarios. Companies often adopt DSS not only to expedite decision-making but also to enhance accuracy by minimizing human errors. Furthermore, these systems permit users to test out hypothetical scenarios, offering valuable insights into potential outcomes without incurring any real-world risk.
Historical Context
Tracing back to their inception, decision support systems emerged in the 1960s as a response to the increasing need for organizations to leverage data in their strategic operations. In the early days, these systems primarily focused on managerial support in large corporations, primarily in finance and logistics sectors. As computing technology evolved, so did the sophistication of DSS. By the 1980s, the advent of personal computers democratized access to these systems, allowing smaller businesses to harness complex analytics without requiring extensive technology infrastructure. This period marked a shift from rudimentary spreadsheet models to more advanced systems that utilized database integrations and simulation models. Today, decision support systems continue to evolve rapidly, incorporating artificial intelligence and machine learning algorithms that drive even deeper insights.
Importance in Modern Business
In the current fast-paced business environment, the ability to quickly adapt and make informed choices is vital for success. Decision support systems play a significant role in various sectors, whether it's finance making data-driven investment decisions or healthcare allocating resources efficiently.
- Enhanced Decision Quality: Businesses can rely on data-backed recommendations, rather than intuition or guesswork.
- Increased Efficiency: By streamlining analysis, teams can focus on strategic initiatives rather than getting bogged down in spreadsheets and manual calculations.
- Scenario Analysis: Managers can model different outcomes based on various scenarios to predict potential impacts before committing resources.
"In todayâs data-driven world, successful companies leverage DSS not just as tools, but as critical components of their strategy."
Additionally, decision support systems facilitate effective collaboration by allowing teams to analyze data together, fostering a culture of transparency and collective decision-making. This democratic approach to utilizing information often leads to more innovative solutions, as diverse perspectives contribute to a holistic understanding of issues.
Embracing decision support systems is not merely an option for modern enterprises, but rather a necessity in harnessing the full potential of their data assets.
Key Components of Decision Support Systems
The effectiveness of any decision support system (DSS) lies in its core components, which work collaboratively to convert raw data into actionable insights. Understanding these components not only enables decision-makers to grasp the architecture of DSS but also helps in enhancing the quality of decisions made across various industry sectors. The importance of these parts cannot be understated, for they each serve a unique function in the decision-making ecosystem, ensuring accuracy, relevance, and user-friendliness.
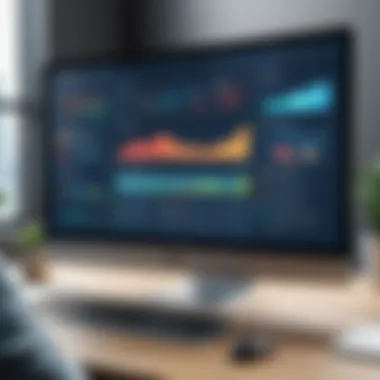
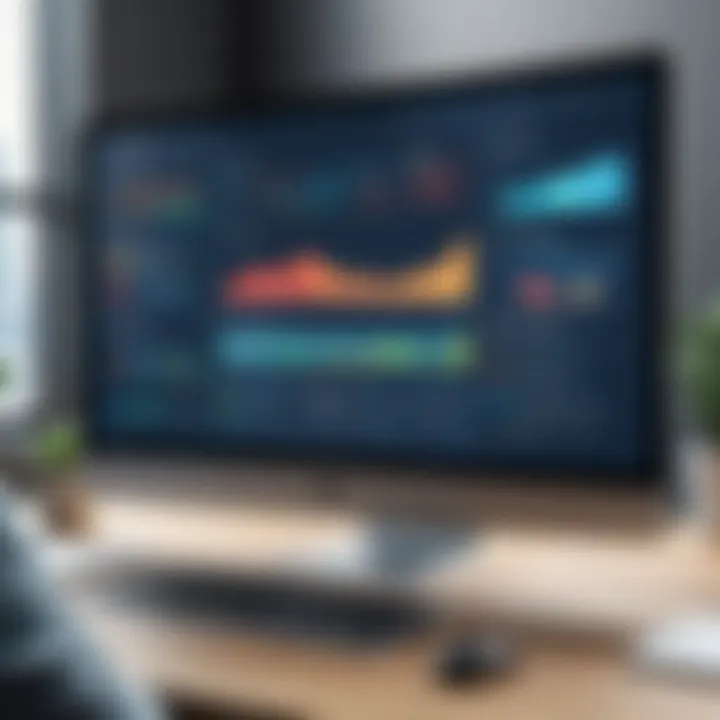
Data Management
Data management stands at the heart of decision support systems. It involves the collection, storage, processing, and retrieval of data which ultimately influences the decisions an organization can make. With vast amounts of data generated every day, having a robust data management framework is essential.
- Quality Over Quantity: Organizations can curate high-quality data inputs and eliminate noise by focusing on what data directly impacts their objectives. This practice improves decision quality immensely.
- Integration Capabilities: Successful data management allows for seamless integration with existing databases or data lakes. Systems like Microsoft SQL Server and Oracle Database can be pivotal in this aspect.
- Real-Time Access: A contemporary DSS must provide users with real-time access to data. This timely information can mean the difference between seizing an opportunity and missing it.
Model Management
Model management refers to the tools and methodologies used to analyze data and generate predictions. The importance of model management transcends mere computation; itâs about how effectively these models can interpret fluctuating data points into business strategies.
- Diverse Modeling Techniques: Different business scenarios call for various modeling techniques. Regression analysis, forecasting methods like ARIMA, and optimization models each serve their respective purposes.
- Version Control: Keeping track of model iterations ensures that decision-makers have the latest and most validated analyses at their disposal. This practice bolsters confidence in strategic choices.
- User Customization: Decision-makers often need to tweak models to fit specific conditions. Thus, flexible modeling platforms enhance usability and applicability.
User Interface
The user interface (UI) of a decision support system plays a crucial role in facilitating user adoption and satisfaction. A well-designed UI should be intuitive and accessible to users with varying levels of technical expertise.
- Ease of Navigation: A clear layout that guides users to relevant data and analysis tools will save time and reduce frustration. This includes well-structured menus, clear labeling, and intuitive workflows.
- Visual Data Representation: Visual aids like graphs, charts, and dashboards can enhance comprehension. Users can quickly grasp complex data relationships when presented visually rather than through dense tables of raw numbers.
- Personalization Options: Allowing users to customize their dashboards according to their preferences can greatly improve their experience and yield better decision-making outcomes.
Knowledge Components
The knowledge components of a DSS encompass the rules, procedures, and insights embedded within the system. These elements not only provide guidance on how to interpret data but also contribute to situations requiring heuristic approaches to problem-solving.
- Expert Systems Integration: By incorporating expert knowledge into decision processes, organizations can benefit from tailored recommendations and best practices that may not be apparent from data alone.
- Documentation and Protocols: Maintaining comprehensive documentation can serve as a valuable guide for users who are strategizing on decisions. It ensures consistency in decision-making processes across departments.
- Continuous Learning Systems: Implementing adaptive systems that evolve based on new data and outcomes keeps the DSS relevant. This continuous learning ensures that the knowledge base grows and becomes more refined over time.
"A decision support system is not merely about technology; itâs about aligning the tool to the human thought process it seeks to support."
Types of Decision Support Systems
Understanding the various types of decision support systems (DSS) is essential for organizations looking to enhance their decision-making capabilities. Each type has unique features and applications that can provide significant benefits in different scenarios. Tailoring the decision support system to specific needs is crucial, and comprehending these distinctions makes all the difference.
Data-driven DSS
Data-driven decision support systems focus primarily on the collection, organization, and analysis of data. Their core functionality lies in transforming vast amounts of raw data into actionable insights. Businesses often find themselves inundated with data, making it a daunting task to extract relevant information. This is where data-driven DSS steps in, allowing users to sort through data sets efficiently.
One key benefit is the ability to conduct advanced statistical analyses and forecasts. For instance, retail chains can analyze customer purchasing behaviors, leading to informed recommendations on stock adjustments or marketing strategies. This adaptability is something that many decision-makers find invaluable in todayâs fast-paced environment.
Moreover, data-driven DSS can be integrated with data visualization tools, making it easier for users to grasp complex data relationships at a glance. The straightforward insights drawn from these systems generally facilitate quicker and more confident decision-making.
Model-driven DSS
Next up, model-driven decision support systems take a different approach by utilizing mathematical and analytical models to assist in the decision-making process. This type marries data with models to simulate potential outcomes and examine scenarios without the need for direct experimentation in the real world.
For example, financial institutions might employ model-driven DSS to assess lending risks. By inputting various parameters into a specific model, they can forecast potential returns or detect risks associated with loans under different market conditions.
Additionally, these systems often incorporate sensitivity analysis, allowing users to understand how different variables impact outcomes. The capacity to modify inputs and observe various scenarios helps organizations prepare for multiple futures, offering a strategic edge.
Knowledge-driven DSS
Knowledge-driven decision support systems are all about leveraging expert knowledge and inference rules to aid decision-making. Unlike data or model-driven systems, knowledge-driven DSS emphasizes the use of expert knowledge and heuristics to provide recommendations.
Take the healthcare sector, for instance. A knowledge-driven DSS can assist physicians by analyzing symptoms alongside a vast database of medical knowledge to suggest diagnoses or treatment plans. This combination of historical knowledge with real-time data enhances the decision-making process significantly.
These systems can also engage in what is known as ârule-based reasoning,â ensuring that decisions made align closely with best practices. This feature is particularly important in environments where compliance and quality control are paramount.
Document-driven DSS
Lastly, document-driven decision support systems are designed to manage and analyze unstructured data such as emails, memos, reports, and other documents. In an age where communication and documentation grow increasingly digital and vast, these systems help sift through information overload.
For example, a legal firm may utilize a document-driven DSS to quickly find pertinent case law documentation or relevant contracts. This saves hours of searching and allows lawyers to focus more on the nuance of their cases rather than clerical work.
Furthermore, the ability to cross-reference multiple documents increases the richness of insights drawn from past interactions and decisions. Such capabilities enable organizations to better leverage their collective knowledge and history in decision-making.
"Understanding the unique functionalities of various decision support systems empowers organizations to make informed and strategic decisions that can shape their future."
In summary, the types of decision support systems cater to different needs and scenarios, providing organizations with the necessary tools to navigate complexities in decision-making environments. By recognizing the strengths of each system, businesses can make more informed choices, ultimately enhancing their operational efficiency.
Analyzing Decision Support Software Options
When it comes to making complex decisions, the right decision support software can be a game changer. In this section, we delve deep into analyzing the options available in the market today. Understanding these software options matters because they can significantly influence efficiency, improve outcomes, and enhance the decision-making process in any organization. This exploration prepares decision-makers to choose wisely, balancing features, costs, and usability to suit specific needs.
Market Overview
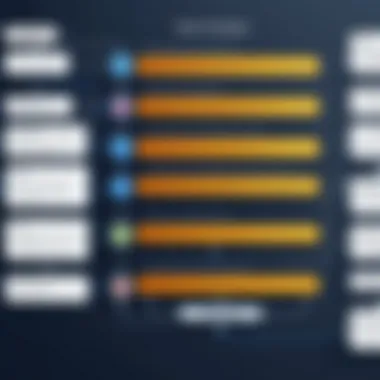
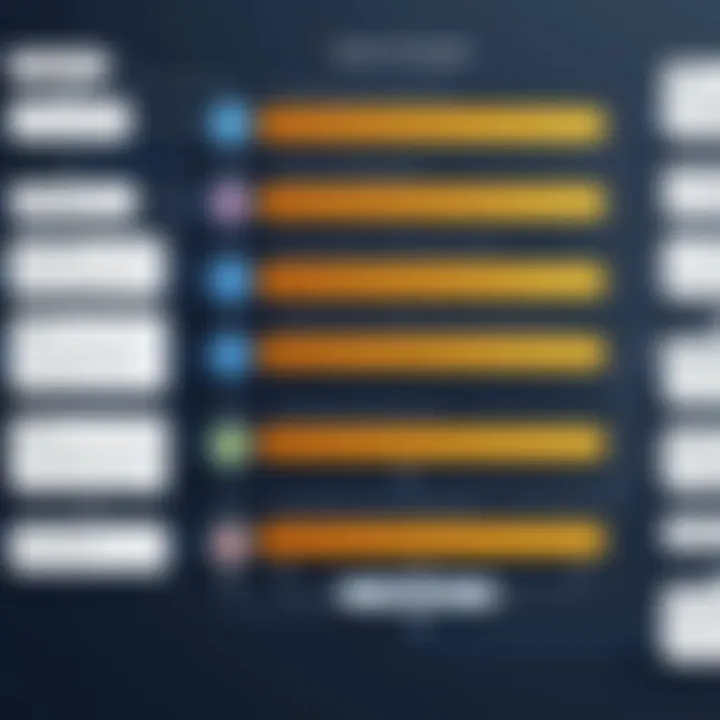
The decision support software market is vast and ever-evolving, with numerous players and a multitude of offerings. This competitive landscape highlights the importance of understanding each option's specifications and unique advantages. Companies are increasingly recognizing the value of these systems, leading to an influx of innovative solutions.
These software tools broadly range from simple spreadsheet programs to sophisticated platforms powered by artificial intelligence. For instance, platforms like Tableau and Microsoft Power BI focus heavily on data visualization, whereas others, such as IBM Watson Analytics, delve into predictive insights.
- Growth Trends: Reportedly, the market is anticipated to grow exponentially due to increasing reliance on data-driven decisions.
- Predominant Industries: Sectors such as healthcare, finance, and logistics are shaping this growth, relying on tailored tools that meet their unique demands.
This overview underscores the essential nature of staying updated on the latest software trends and capabilities.
Comparative Analysis of Popular Software
In evaluating decision support software, comparing popular choices helps demystify the options available. This analysis aims to juxtapose some leading tools in the market based on essential criteria such as functionality, ease of use, and interoperability.
Popular Choices:
- Tableau: Renowned for its powerful data visualization options, Tableau enables users to create interactive dashboards with ease. Its intuitive interface is particularly well-suited for data analysis and storytelling.
- Microsoft Power BI: A robust option that integrates seamlessly with other Microsoft products. It also offers impressive data modeling capabilities.
- IBM Watson Analytics: This software leverages AI to uncover patterns and provide predictive insights, making it immensely valuable for complex decision-making scenarios.
Features like data sharing, mobile access, and cloud services become key differentiators in this competitive field. Decision-makers can enhance organization-wide visibility by selecting software that aligns with their needs and existing infrastructure.
Vendor Selection Criteria
Choosing the right vendor is as critical as selecting the software itself. Several factors must be taken into consideration to ensure alignment with organizational goals and challenges.
Important Factors:
- Support and Training: Ensure the vendor provides comprehensive support and training resources. A software solution is only as effective as the users' ability to utilize it.
- Customization Capabilities: Assess how well the software can be tailored to meet unique organizational requirements.
- Scalability: Evaluate if the solution can grow alongside your organization. Future-proofing the software investment is crucial for long-term success.
- Reputation and Reviews: Research any available customer feedback and case studies. Vendors with a strong track record usually provide more reliable software solutions.
Choosing a vendor shouldn't be a shot in the dark; thorough research and deliberation are pivotal. "An informed choice today can save you headaches tomorrow." This sage advice resonates particularly true in navigating the complexities of decision support systems.
In essence, a comprehensive analysis of decision support software options is vital for organizations that intend to improve their decision-making processes. By systematically understanding what is available and practicing due diligence in the selection process, organizations can capitalize on the benefits that the right software delivers.
Best Practices for Successful Implementation
Implementing a decision support system (DSS) is not just about rolling out software; it's a holistic process that can dictate how effectively your organization uses data to make decisions. A well-executed implementation can significantly enhance the value this technology brings to the table, while a poorly managed process can lead to wasted resources and missed opportunities. With the right best practices, companies can harness the full power of their DSS, ensuring that the system serves its purpose effectively and yields long-term benefits.
Requirement Analysis
Requirement analysis serves as the foundation of successful DSS implementation. This phase involves deeply understanding the needs of the organization and what specific problems the DSS will resolve. The process should include:
- Stakeholder Interviews: Engaging with various users, from executives to field workers, helps gather diverse insights that influence system design.
- Defining Objectives: Clearly articulating what decisions the DSS needs to support can guide the selection of features and functionalities. Ask questions like: What data will be critical? What outputs do we expect to generate?
- Assessing Current Processes: Reviewing existing decision-making methods ensures that the DSS is tailored to fit or improve upon those processes rather than complicate them.
Ultimately, sufficient time and effort put into requirement analysis will clarify expectations and set the stage for successful deployment.
Engaging Stakeholders
Engaging stakeholders throughout the implementation process canât be overstated. Everyone who has a stake in the decision-making process should feel involved and informed. This includes not only top management but also end-users who may ultimately hold the keys to successful utilization. Hereâs how to facilitate engagement:
- Regular Communication: Keeping stakeholders in the loop helps build trust and ensures everyone understands the system's goals and their roles in the implementation.
- Collaborative Decision-Making: Encourage stakeholders to provide input during system selection and deployment phases, thereby fostering ownership of the new system.
- Incentives for Participation: Recognizing contributions, offering visible roles in the change management process, and even providing training can motivate stakeholders to take an active part.
Involving the key players from the get-go not only minimizes resistance but can also unveil insights that might have been overlooked otherwise.
Training and Support
Training is where the rubber meets the road. No matter how sophisticated a DSS is, if users don't know how to navigate it, its potential withers away. An effective training program should include:
- Hands-On Workshops: Instead of a one-size-fits-all tutorial, bespoke training programs designed for different user levels can cater to specific needs.
- Documentation: Providing easy access to user manuals, both digital and printed, offers a reference point as users become more acquainted with the system.
- Ongoing Support: Post-implementation support is vital. Setting up help desk resources or fostering a community of users who can share tips can make a big difference in how quickly individuals adapt.
Investing in comprehensive training programs not only enhances user confidence but significantly reduces the likelihood of errors during the decision-making process.
Evaluating System Performance
Once the DSS is up and running, itâs important to keep a finger on the pulse of its performance. Evaluating how well the system meets the organizational goals ensures its continued relevance. Hereâs how evaluation can be structured:
- Feedback Mechanism: Set up channels for users to share experiences regarding system efficiency, ease of use, and any hiccups encountered during operation.
- Performance Metrics: Identify key performance indicators pertinent to the DSS objectives. It could encompass factors like decision turnaround time, accuracy of predictions, and user satisfaction rates.
- Regular Review Meetings: Schedule periodic reviews with stakeholders to discuss findings, assess the softwareâs effectiveness, and identify areas requiring adjustments or enhancements.
With a continuous evaluation approach, organizations can not only troubleshoot issues as they arise but also adapt to the ever-changing business landscape.
Challenges in Decision Support Systems
In the realm of decision support systems, navigating the challenges is often as intricate as guiding a ship through foggy waters. These systems are designed to aid decision-making processes, but various hurdles can dampen their effectiveness and adoption. Understanding these challenges is crucial not just for IT professionals, but for decision-makers who rely on these systems to steer organizational strategies. When organizations overlook the significant hurdles that can arise, they may find themselves at a crossroad, unable to extract the full potential from their investments.
Data Quality Issues
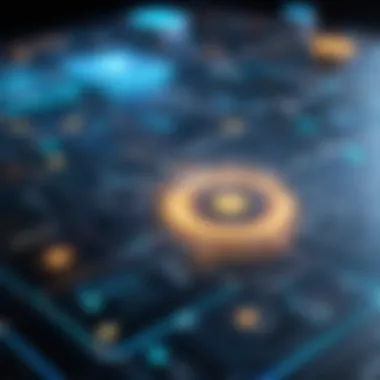
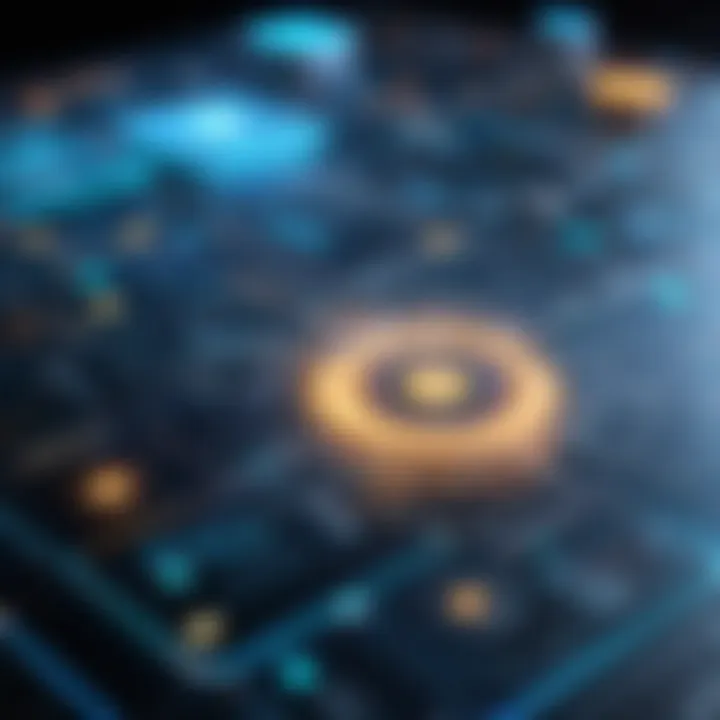
The foundation of any reliable decision support system is the quality of the data it processes. Poor data quality can lead to misleading insights, which ultimately drive suboptimal decisions. A common pitfall many face is assuming that all data collected is inherently valuable; however, this isnât always the case. Data may be outdated, incomplete, or even incorrect, creating a domino effect that undermines the trust placed in the system.
Ensuring data accuracy requires ongoing diligence, including:
- Regular audits to identify inaccuracies and discrepancies.
- Standardization of data entry protocols to maintain consistency.
- User training on the importance of data accuracy to foster a culture that values quality.
In an age where data is often considered the new oil, stakeholders must remain vigilant about where their "oil" comes from and how it is refined before being put to use.
Integration with Existing Systems
Another stumbling block emerges when organizations attempt to integrate new decision support systems with existing infrastructure. This can be akin to fitting a square peg into a round hole. The nuances involved in blending diverse technologies can lead to functionality gaps, data silos, or even significant downtime.
To combat integration issues, consider these strategies:
- Conduct thorough assessments of current systems to understand compatibility.
- Involve stakeholders early in the integration process to gather insights and address concerns.
- Utilize middleware solutions to enhance the communication between systems.
Managing integration effectively means creating a synergetic ecosystem where systems elevate one another, rather than create friction.
User Adoption Barriers
No matter how sophisticated a system is, if its users resist it, the technology's benefits will never be realized. This issue often arises due to a lack of proper training or a communication gap about the system's advantages. To enhance user adoption, organizations should consider these aspects:
- Provide comprehensive training sessions tailored to different user groups.
- Foster a user-friendly interface that invites exploration rather than intimidation.
- Encourage feedback and address concerns rapidly to enhance the user experience.
When users feel invested in the system, their objections turn into advocacy, transforming initial hesitations into operational benefits.
To thrive in the face of these challenges, itâs essential for organizations to actively engage in proactive strategies to mitigate risks associated with data quality, integration, and user adoption. By addressing these concerns head-on, they can carve a path toward successful implementation and maximize the potential of their decision support systems.
Future Trends in Decision Support System Software
The landscape of decision support systems (DSS) is rapidly transforming, driven by technological advancements and emerging business needs. Recognizing the future trends in decision support system software is crucial for organizations aiming to stay ahead in the decision-making game. This section outlines three significant trends shaping the evolution of DSS, delving into artificial intelligence integration, big data analytics, and cloud-based solutions.
Artificial Intelligence Integration
Incorporating artificial intelligence into decision support systems stands as a pivotal trend. AI enhances the capability of DSS by automating routine tasks, analyzing large datasets quickly, and providing actionable insights with a level of precision that manual processes struggle to achieve.
With AI, decision-makers can expect:
- Predictive Analytics: By leveraging machine learning algorithms, organizations can forecast future trends based on historical data. For instance, retailers may predict consumer behavior during holiday seasons, allowing them to optimize stock levels.
- Natural Language Processing (NLP): Users can interact with systems using human language, making the tools more intuitive. This could be particularly beneficial for those less tech-savvy, as they engage with DSS through conversational interfaces.
- Enhanced Scenario Analysis: AI can simulate different scenarios based on variable data inputs. This allows companies to conduct what-if analyses that support strategic planning.
"Using AI in decision support systems does not just enhance efficiency; it revolutionizes the core of how data is interpreted and leveraged."
Big Data Analytics
The explosion of big data is prompting organizations to seek sophisticated decision support software that can handle vast volumes of information. Big data analytics allows companies to sift through and make sense of data that was previously overwhelming.
Organizations now face the possibility of:
- Real-time Data Processing: Immediate analytics enable timely decisions. For example, banks using big data can assess transaction risks in seconds, effectively preventing fraud.
- Data Visualization Tools: These tools present complex data in digestible formats, fostering better understanding. Dashboards and charts help executives grasp information quickly and take informed actions.
- Improved Customer Insights: By analyzing large datasets from various sources, businesses can understand customer preferences more deeply, leading to more targeted marketing campaigns and enhanced customer satisfaction.
Cloud-based Solutions
Shifting to the cloud is another trailblazing trend in the DSS arena. Cloud-based solutions entail several advantages that appeal to a diverse range of organizations, particularly those emphasizing flexibility and scalability.
Key benefits include:
- Accessibility: Stakeholders can access the decision support system from anywhere at any time, using internet-enabled devices. This increases collaboration, especially for remote teams.
- Cost-effectiveness: With cloud solutions, organizations can reduce expenses associated with on-premises hardware and IT support. Subscription models enable businesses to pay for what they use, making it financially sustainable.
- Scalability: As business needs evolve, cloud infrastructure allows for easy integration of additional resources or tools without significant downtime or investment.
Culmination
The conclusion chapter serves as a pivotal element of the article, weaving together the intricate threads of knowledge discussed throughout. It encapsulates the essence of decision support system software and its profound impact on decision-making across various sectors. With the rapid evolution of industries, comprehending the nuances of these systems has never been more critical.
Recap of Key Points
To summarize, some of the cornerstone ideas highlighted include:
- Definition and Purpose: Decision support systems act as frameworks that assist organizations in making informed choices by analyzing data and presenting actionable insights.
- Components and Functionality: Core elements such as data management, model management, and user interface design define the robustness and usability of these systems.
- Types of DSS: We examined different varieties such as data-driven and model-driven decision support systems, showcasing their unique advantages.
- Implementation Best Practices: Emphasis was placed on thorough requirement analyses and the importance of user training, ensuring that stakeholders are well-equipped to leverage these tools.
- Challenges and Future Trends: Identifying potential obstaclesâlike data quality and integration issuesâas well as exploring future trends like AI integration and cloud solutions, sets organizations up for long-term success.
By distilling these points, decision-makers can reflect on how decision support system software can elevate their operations.
Strategic Insights
The key takeaway for decision-makers and IT professionals is to recognize that leveraging decision support systems goes beyond mere technology adoption. It involves a strategic shift in mindset where data is viewed not just as numbers, but as a vital asset that informs every decision.
In practical terms, organizations should focus on:
- Holistic Integration: Rather than viewing these systems as standalone tools, itâs essential to integrate them within a broader organizational framework. This creates a synergistic effect, enhancing cooperation among departments and aligning objectives.
- Continuous Evaluation: The effectiveness of decision support software is not a one-time check. Organizations must periodically assess system performance against set benchmarks to ensure they remain responsive to changing needs.
- User-Centric Design: Prioritizing an intuitive user interface can enhance user adoption and engagement. Collecting user feedback and iterating on design can foster a culture of continuous improvement.
Ultimately, the journey of mastering decision support system software is ongoing. By keeping abreast of current developments and aligning their strategies accordingly, businesses can enhance not only their decision-making capabilities but also their competitive edge in an increasingly data-driven world.
In the words of Albert Einstein, "The measure of intelligence is the ability to change." Adaptation to changing technologies like decision support systems is crucial for sustained success.