Unlocking Business Insights with SAP Predictive Analytics
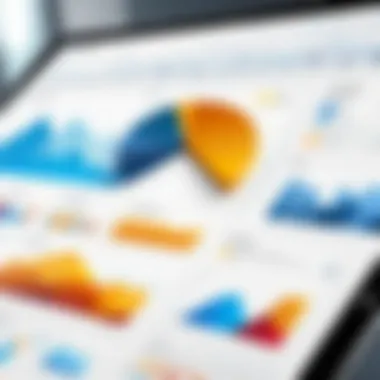
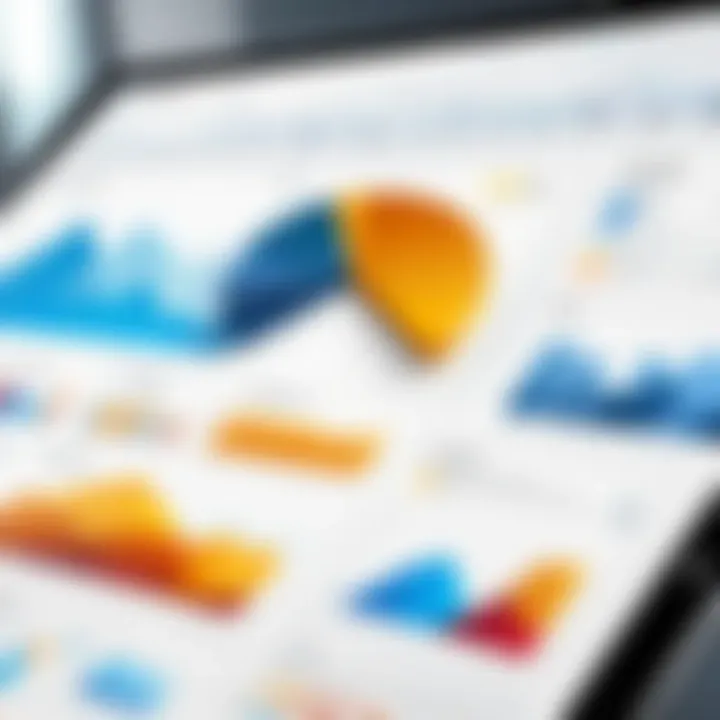
Intro
In a world where data is at the forefront of business strategy, SAP Analytics Cloud's predictive analytics emerges as a pivotal instrument. This section lays the groundwork for an intricate examination of the capabilities offered by this platform. Decision-makers and connected professionals must understand not only the intricate features of this tool but also how they can translate data into foresight and thus into better business outcomes.
The significance of data-driven decision-making cannot be overstated. Leveraging predictive analytics positions businesses to anticipate trends, optimize processes, and respond proactive to changing market dynamics. In the following sections, we will deliberate on the features, integration practices, and some challenges associated with adopting this technology.
Overview of Core Features
SAP Analytics Cloud introduces a suite of core functionalities designed to enhance predictive capabilities within business contexts. Some of the key features include:
- Smart Predict: This feature employs machine learning techniques to provide automated predictions based on historical data. It helps users derive insights without needing extensive knowledge of statistical methods.
- Data Exploration: Users can navigate and visualize their data easily. This allows decision-makers to identify patterns and anomalies that traditional analysis might overlook.
- Collaboration Tools: With integrated tools for collaboration, teams can communicate insights and drive action effectively.
- Scenario Planning: Businesses can create multiple scenarios to understand potential outcomes and impacts on their operations, which is crucial for strategic planning.
- Integration Capabilities: The platform allows for seamless integration with various data sources, ensuring users can analyze data from different environments without hassle.
Comparison of Features Across Top Software Options
When comparing SAP Analytics Cloud’s features with other leading software options like Tableau and Microsoft Power BI, specific differentiators stand out:
- Ease of Use: SAP Analytics Cloud features a user-friendly interface, yet requires a certain level of familiarity with data analytics.
- Depth of Predictive Functionality: While Tableau provides visual analytics, SAP's Machine Learning capabilities are more integrated, offering more robust predictive functions.
- Collaboration: Unlike Microsoft Power BI, which may necessitate third-party tools for collaboration, SAP’s built-in features streamline communication and insight sharing.
These distinctions are vital for organizations evaluating their analytics tools.
User Experience and Interface
Usability is an essential component in how professionals interact with predictive analytics. SAP Analytics Cloud emphasizes an intuitive UI/UX design that prioritizes the user experience. Users can seamlessly navigate through complex datasets without much technical knowledge.
A clear and responsive interface enhances productivity and ensures that users spend more time discovering insights than wrestling with the tool itself. Accessibility features also come into play here; ensuring that data can be analyzed and interpreted efficiently by diverse teams is critical.
The design choices in SAP Analytics Cloud underscore the importance of making data analytics approachable, allowing organizations to harness powerful insights without overwhelming their teams.
"The ability to translate data into actionable insights depends significantly on the accessibility and intuitiveness of the analytical tools employed."
In summary, understanding the core features, user experience, and the element of usability in SAP Analytics Cloud is crucial for any business aiming to adopt predictive analytics successfully. The subsequent sections will delve into potential challenges faced during implementation, best practices for optimal usage, and future trends worth considering.
Prologue to SAP Analytics Cloud
SAP Analytics Cloud is a critical component in the realm of data analytics and business intelligence. It integrates various functionalities such as business planning, predictive analytics, and data visualization in a single cloud-based platform. Companies today face mountains of data, and SAP Analytics Cloud helps in transforming this data into actionable insights. It empowers decision-makers to steer their organizations towards a data-driven future.
The importance of understanding SAP Analytics Cloud cannot be overstated, especially given the increasing reliance on digital transformation across industries. Organizations today require tools that not only analyze past performance but also predict future trends, thus ensuring they remain competitive in a fast-paced market. As businesses navigate complexities in their operations, predictive analytics becomes a game changer. It provides foresight into potential challenges and opportunities, allowing for proactive decision-making.
Considered a comprehensive analytical tool, SAP Analytics Cloud offers numerous benefits:
- Real-time insights: With real-time data processing, organizations can make timely decisions.
- User-friendly interface: It features an intuitive design that enhances user experience, even for those with limited technical skills.
- Collaboration features: Teams can work together seamlessly, ensuring varied perspectives are considered in decision-making.
- Advanced functionalities: Predictive analytics capabilities allow businesses to analyze trends and patterns, generating forecasts that can lead to informed strategies.
As organizations adopt SAP Analytics Cloud, they face critical considerations. Data security remains a priority, as sensitive information flows through the system. Additionally, integrating this tool with existing infrastructures can be challenging. However, the long-term benefits often outweigh these initial hurdles, especially as businesses capitalize on advanced analytics to refine their strategies.
"In a world increasingly characterized by data, those who learn to harness its power will lead the way."
Ultimately, SAP Analytics Cloud serves as a pivotal resource for organizations aspiring to enhance their predictive analysis capabilities. By facilitating data-driven decisions, it supports strategic growth and operational efficiency, making it an essential focus in today’s landscape.
Understanding Predictive Analytics
Predictive analytics is an essential component in modern business environments. It employs statistical algorithms and machine learning techniques to analyze historical data and provide forecasts about future events. This approach to data analysis is not merely a trend but a significant paradigm shift in how organizations make decisions.
The importance of predictive analytics lies in its ability to uncover patterns and trends which might not be evident through traditional analysis. For instance, companies utilize these insights to anticipate customer behavior, forecast sales, and optimize operational processes. By transforming data into actionable insights, predictive analytics fosters informed decision-making and strategic planning.
The Benefits of Predictive Analytics
Organizations that adopt predictive analytics can enjoy a range of benefits, including:
- Improved Decision-Making: Management can make strategic choices based on data-driven insights rather than intuition or outdated information.
- Enhanced Accuracy: Predictive models can increase the accuracy of forecasts, allowing businesses to prepare for scenarios that may affect operations.
- Resource Optimization: Businesses can allocate resources more effectively by understanding potential outcomes through predictive modeling techniques.
Considerations Regarding Predictive Analytics
However, integrating predictive analytics into existing workflows requires careful consideration. Companies must ensure they have access to quality data, as the accuracy of predictive outcomes directly depends on the data used to train models. Furthermore, there is a need for skilled personnel who can interpret analytics results. This skill gap can hinder the effective implementation of predictive analytics in organizations.
Additionally, not all data is suitable for predictive modeling. Organizations need to identify and accumulate relevant data that can contribute to a robust analysis. Lack of alignment on the business objectives can also lead to ineffective usage of the predictive analytics tools.
"Predictive analytics transforms data into foresight. It's about understanding the future through the lens of the past."
In summary, predictive analytics is a powerful tool that helps businesses navigate through uncertainty by providing valuable foresight. The implications of effectively utilizing predictive analytics are significant, as companies can take proactive measures to address challenges and seize opportunities. This capability makes predictive analytics not merely an ancillary function but a core component of strategic business practices.
Key Features of SAP Analytics Cloud
The Key Features of SAP Analytics Cloud play a significant role in understanding its predictive analytics capabilities. These features are not just technical specifications; they provide the framework for an agile, responsive, and insightful analytics environment. Organizations increasingly rely on data to drive decisions, and SAP Analytics Cloud offers essential tools to harness that data effectively.
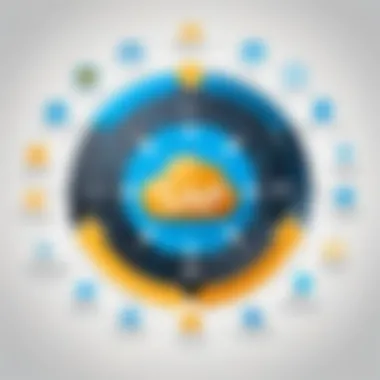
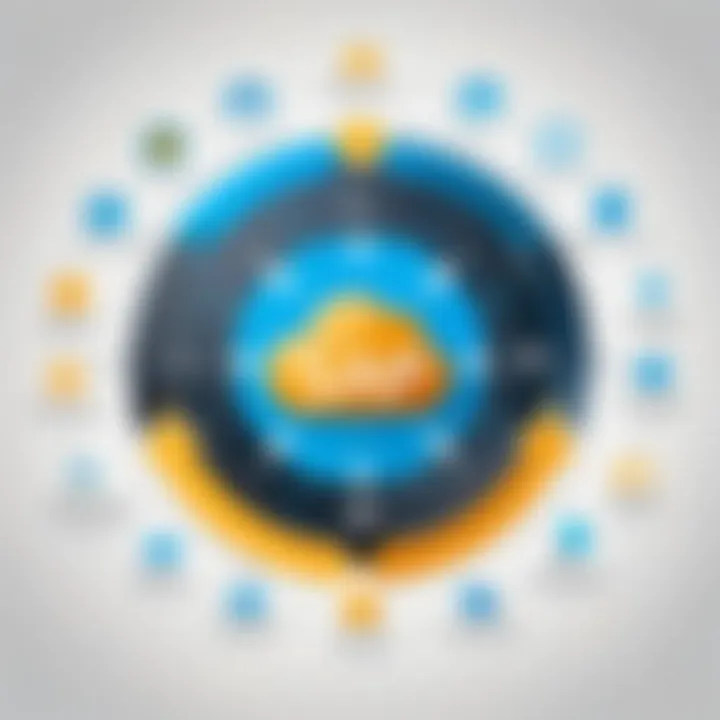
Key features can elevate business performance. They facilitate the synthesis of data from multiple sources, enabling a more comprehensive view of operations. The significance of these features extends beyond mere data analysis; they empower analysts and business leaders to make data-driven decisions. The seamless integration and user-friendly layout are pivotal in removing barriers to efficient data consumption.
User Interface and Experience
The user interface of SAP Analytics Cloud is designed with the end-user in mind. It adopts a clean and intuitive layout that minimizes the cognitive load for users. This design not only improves the experience but also enhances productivity. Users can navigate through dashboards, access analytics tools, and visualize data with ease.
"An intuitive user interface can significantly reduce the time required to derive insights from data."
Key elements of the user interface include customizable dashboards that allow users to tailor their views based on their specific needs. This personalization enables users to focus on the metrics that matter most to them. Moreover, collaborative features provide a space for teams to share insights and analytics, fostering an environment of collective intelligence and teamwork.
Data Connectivity Options
Data connectivity is a vital component of SAP Analytics Cloud. It supports a wide variety of data sources, making it flexible for different business environments. This capability allows users to integrate datasets from several origins, including cloud data services, on-premise environments, and even external third-party sources.
Users can connect to sources such as SAP HANA, Microsoft Azure, and other essential databases. This ensures that the analytics are not conducted in isolation but rather reflect the wider organizational data landscape.
The ability to connect seamlessly promotes consistency and accuracy in reporting. Additionally, it enables real-time data analysis, allowing organizations to stay ahead of trends and respond to market dynamics effectively. Integrating diverse data sources enhances predictive analytics outcomes, providing richer insights and fostering more informed decision-making.
Integration with Other SAP Products
Integration with other SAP products is a crucial aspect of utilizing SAP Analytics Cloud for predictive analytics. By seamlessly linking various SAP platforms, organizations can enhance their analytical capabilities and drive insightful decision-making. This integration facilitates data consistency, enables real-time analysis, and maximizes the value of existing SAP data sources. The interconnectedness of these systems creates a robust ecosystem that supports advanced analytics and boosts overall operational performance.
SAP S/4HANA Integration
SAP S/4HANA integration plays a pivotal role in the predictive analytics landscape. As a cornerstone of enterprise resource planning (ERP), S/4HANA streamlines business processes and enhances data accessibility. When connected with SAP Analytics Cloud, S/4HANA allows for immediate data retrieval and analysis, which is crucial for timely decision-making.
- Data Synchronization: The integration ensures that the data is continually updated between systems, thus maintaining accuracy and relevance. This becomes particularly important for predictive models that rely on recent data trends to generate forecasts.
- Enhanced Analytical Capabilities: With S/4HANA's advanced analytics embedded within, users can execute predictive algorithms directly on operational data, uncovering actionable insights efficiently.
- User Experience: Integration simplifies the user experience by providing a unified interface, allowing stakeholders to access predictive analytics features without the need for switching between multiple platforms.
This synergy not only improves productivity but also supports strategic initiatives by equipping users with the tools to perform complex analyses effortlessly.
Compatibility with SAP Data Warehouse
The compatibility between SAP Analytics Cloud and SAP Data Warehouse is another significant consideration for businesses looking to implement predictive analytics. A well-architected data warehouse serves as a centralized repository that stores both structured and unstructured data from various sources. Integrating it with SAP Analytics Cloud enhances analytical capabilities.
- Data Journey: Utilizing the data warehouse in conjunction with the analytics cloud streamlines the data journey, from extraction to analysis. The seamless flow of information allows organizations to harness their data assets efficiently.
- Centralized Reporting: The integration enables centralized reporting, making it easier for businesses to generate insights from a wide range of data sources. Decision-makers can access consolidated reports and visuals captured in real-time.
- Scalability and Flexibility: As business needs evolve, the combination of these tools offers scalability. Organizations can adjust their analytics strategies accordingly, without compromising on performance or insights.
Integrating SAP Data Warehouse with SAP Analytics Cloud allows businesses to unleash the full power of their data, driving transformative insights and innovative strategies.
The Predictive Analytics Lifecycle
The predictive analytics lifecycle represents a structured approach to leveraging data for business insights. By understanding this lifecycle, organizations can effectively manage their analytics processes, ensuring that they derive the most value from their data initiatives. This section centers on the key components of the lifecycle and describes its importance in the broader context of SAP Analytics Cloud.
A comprehensive predictive analytics lifecycle includes several critical steps: data preparation, modeling techniques, and validation processes. Each of these stages contributes to the overall effectiveness of predictive analytics in informing business decisions. In an age where data-driven strategies define competitive advantage, mastering this lifecycle becomes essential.
Data Preparation for Predictive Models
Data preparation is the foundational step in predictive analytics. This process involves the collection, cleaning, and transformation of raw data into a structured format suitable for analysis. Without adequate preparation, models may yield inaccurate results or insights that do not align with business goals. It is crucial to ensure that data is both relevant and high quality.
Important considerations include:
- Data Cleaning: Removing duplicates and correcting errors ensures accuracy in the dataset.
- Feature Selection: Identifying the most relevant variables allows the model to focus on what truly matters.
- Transformation: Normalizing data can help in making disparate datasets comparable, improving model performance.
Effective data preparation can significantly enhance the predictive capability of models, thereby influencing business outcomes. Properly prepared data serves as a robust foundation for subsequent modeling processes.
Modeling Techniques and Algorithms
Once data is prepared, the next stage in the lifecycle is selecting appropriate modeling techniques and algorithms. The choice of technique directly impacts the predictions and insights derived from the data. Different algorithms serve different needs, and understanding these distinctions is key for effective analysis.
Some common modeling techniques include:
- Regression Analysis: Useful for predicting a continuous outcome based on one or more predictor variables.
- Classification: Techniques such as decision trees or logistic regression focus on categorizing data into classes.
- Time Series Analysis: Ideal for forecasting future values based on past data points.
Implementing the right algorithm requires balancing model complexity with interpretability. Businesses should seek models that not only predict accurately but also provide insights into how decisions can effectively be made based on the output.
Validation and Testing of Models
Validation is a critical phase in the predictive analytics lifecycle. It determines the reliability of the model's predictions and helps in fine-tuning its parameters. Businesses must rigorously evaluate their models to identify potential inaccuracies and biases.
Key steps in validation include:
- Cross-Validation: This technique involves dividing the data into training and test sets. By evaluating model performance on unseen data, organizations can better assess its robustness.
- Performance Metrics: Metrics such as accuracy, precision, and recall help gauge how well the model performs relative to its objectives.
- Iteration: Predictive models may require multiple iterations of tuning and re-evaluation before they are deployed in a live environment.
Ultimately, a sound validation process boosts the confidence of decision-makers in using predictive analytics in strategic planning. Ensuring that models are reliable leads to more effective use of insights drawn from analyses, promoting data-driven cultures in organizations.
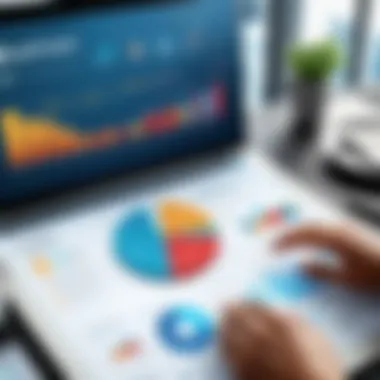
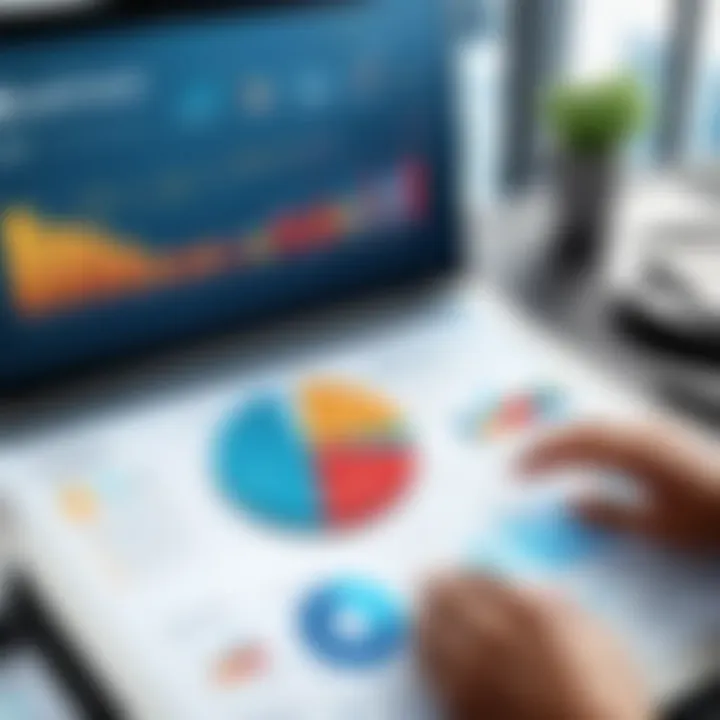
Applications of Predictive Analytics in Business
Predictive analytics is becoming essential for businesses seeking to gain a competitive edge. Integrating this capability into strategic planning allows organizations to make data-driven decisions. Through sophisticated algorithms and statistical models, companies can forecast future trends and optimize their operations. Understanding these applications can lead to significant benefits, allowing firms to allocate resources more effectively and enhance customer satisfaction.
Sales Forecasting
Sales forecasting is one of the most impactful applications of predictive analytics. By analyzing historical sales data, companies can identify patterns and predict future sales performance. This process minimizes uncertainty and enables businesses to set realistic targets.
Employing techniques such as time series analysis and regression models, organizations can observe seasonal trends and shifts in consumer behavior. For instance, retailers might notice an increase in sales during holiday seasons and adjust inventory levels accordingly.
The main benefits of effective sales forecasting include:
- Improved inventory management
- Enhanced resource allocation
- Increased sales efficiency
By making informed forecasts, businesses can tailor their marketing strategies and boost sales opportunities while mitigating risks.
Customer Relationship Management
Predictive analytics plays a pivotal role in customer relationship management (CRM). By leveraging data about customer interactions, preferences, and behaviors, firms can create more personalized experiences. This approach enables businesses to anticipate customer needs, leading to higher satisfaction.
Analyzing data from various sources, such as social media and purchase histories, allows for better segmentation of the customer base. As a result, targeted marketing campaigns can be designed, improving engagement and loyalty.
The critical components of this application include:
- Churn prediction: Identifying at-risk customers before they disengage.
- Product recommendations: Tailoring suggestions based on past purchases.
- Sentiment analysis: Understanding customer opinions to refine service.
Predictive analytics can transform CRM from a reactive process into a proactive strategy.
Inventory Management
The significance of predictive analytics in inventory management cannot be overstated. By forecasting demand, businesses can maintain optimal inventory levels. This practice minimizes excess stock while ensuring products are available when needed.
Techniques like machine learning algorithms help analyze trends from various data points, enabling businesses to forecast demand more accurately. Factors such as market cycles, promotional events, and even weather conditions can be incorporated to improve predictions.
Key advantages include:
- Reduced carrying costs
- Fewer stockouts and lost sales
- Enhanced operational efficiency
Incorporating predictive analytics into inventory management equips businesses to respond swiftly to market changes, ultimately supporting improved profitability.
Predictive analytics in business is not just about knowing what will happen but acting on that insight before it occurs.
Benefits of Using Predictive Analytics
Predictive analytics has become a cornerstone for many organizations seeking to refine their decision-making processes and operational strategies. This section will delve into some critical benefits that predictive analytics can bring to businesses, particularly when integrated within SAP Analytics Cloud. Understanding these advantages is essential for professionals who wish to exploit data for strategic gain.
Enhanced Decision-Making
One significant benefit of using predictive analytics is its potential to enhance decision-making. Businesses today face a deluge of data, and making sense of it can be overwhelming. Predictive analytics enables organizations to analyze historical data trends and forecast future outcomes. By providing insights drawn from data patterns, decision-makers can rely on more than just intuition.
- Data-Driven Insights: Predictive analytics transforms raw data into actionable insights. This approach allows businesses to anticipate customer behavior, market trends, and overall business performance with greater accuracy.
- Risk Mitigation: By predicting potential challenges and pitfalls, companies can mitigate risks more effectively. For instance, financial institutions can use predictive models to assess credit risk and improve loan approval processes.
- Improved Customer Engagement: Organizations are better equipped to personalize customer interactions. Understanding the likelihood of customer churn or preferences can enable tailored engagement strategies, ultimately resulting in increased loyalty and retention.
"Making decisions based on data and analysis rather than guesswork creates a competitive edge."
Operational Efficiency
Operational efficiency is another crucial area where predictive analytics can provide substantial benefits. Firms often strive for streamlined processes that minimize waste and maximize productivity. Predictive capabilities play a pivotal role in achieving these goals.
- Optimized Resource Allocation: By predicting future demand, businesses can allocate resources more effectively. For example, in the supply chain, predictive analytics can forecast inventory needs, reducing excess stock and cutting holding costs.
- Automation of Processes: Many organizations are increasingly interested in automating routine decisions based on predictive insights. This not only speeds up operations but also lessens the burden on human resources, allowing staff to focus on higher-value tasks.
- Performance Monitoring and Continuous Improvement: Predictive analytics enables ongoing performance assessments. By continuously monitoring processes and outcomes, businesses can identify areas for improvement, driving efficiency over the long term.
In summary, the integration of predictive analytics, particularly through SAP Analytics Cloud, vastly improves the decision-making process and enhances operational efficiency. Organizations can harness their data not only to predict future scenarios but also to execute strategies that sharpen their competitive edge.
Challenges in Implementing Predictive Analytics
In the context of SAP Analytics Cloud, implementing predictive analytics presents notable challenges that organizations must navigate. These challenges not only influence the effectiveness of predictive models but also impact the overall decision-making process. Understanding these elements is vital for ensuring that organizations can reap the full benefits from their investments in predictive analytics. Addressing these challenges can lead to enhanced data-driven decision-making and more efficient operations.
Data Quality and Availability
Data quality is pivotal in predictive analytics. Poor quality data can result in inaccurate predictions, undermining the trust in analytical outcomes. Businesses often struggle with a mix of data sources, leading to inconsistencies in data formats and structures. Some common issues include missing values, duplicates, and outdated information. Organizations must ensure that they have robust data governance frameworks in place. This framework should focus on data cleansing, normalization, and enrichment processes to maintain systematic data integrity.
Additionally, data availability plays a crucial role. If relevant data is not available or is difficult to access, creating predictive models faced by data scientists becomes problematic. Organizations should prioritize strategies that enhance data accessibility. This can include deploying advanced data integration tools and connecting various data silos. One of the goals here is to create a consolidated view of data from disparate sources within the business. This is important for building accurate predictive models that can inform key decisions about future actions.
Skill Gaps within Organizations
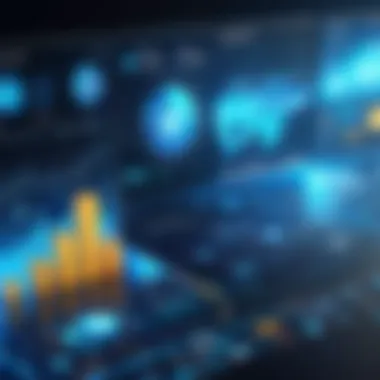
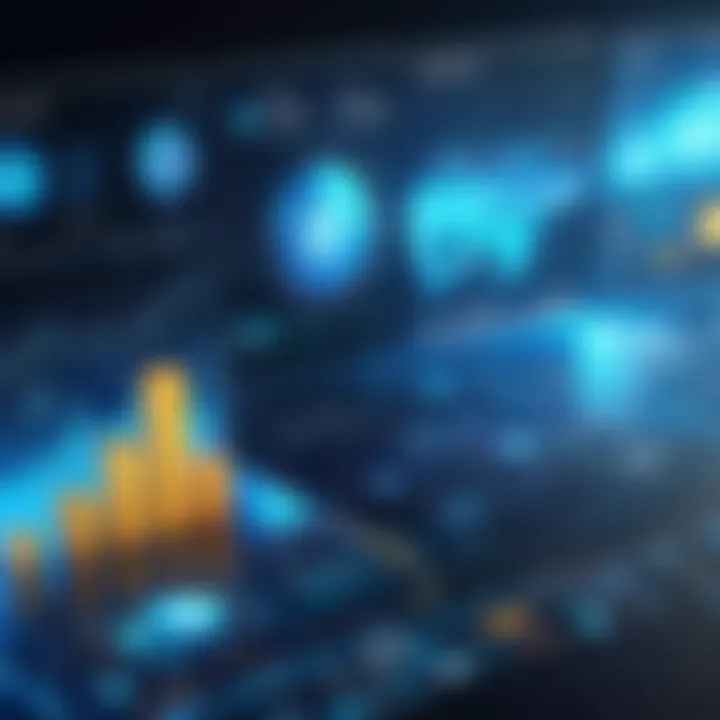
The workforce's skill sets can significantly influence the successful adoption of predictive analytics. Often, businesses face talent shortages when it comes to data science and predictive analytics. Many employees may not have the necessary skills to effectively interpret data findings or create predictive models. This skill gap presents barriers not only in the creation of analytics but also in effectively communicating results to stakeholders.
Organizations must invest in training programs to upskill their current workforce. This includes education around data literacy, statistical methods, and the usage of software tools such as SAP Analytics Cloud. Alternatively, hiring skilled data professionals or partnering with external consultants can help bridge this gap, ensuring that the organization has the expertise required to implement predictive analytics effectively.
"Addressing skill gaps is essential for maximizing the impact of predictive analytics within an organization."
In summary, navigating challenges such as data quality and availability, as well as skill gaps, is essential for organizations looking to leverage predictive analytics. By addressing these issues head-on, businesses can enhance their analytical capabilities and foster a culture that values data-driven decision-making.
Best Practices for Effective Implementation
The successful implementation of SAP Analytics Cloud's predictive analytics capabilities is not merely about deploying technology; it requires a thoughtful approach to ensure its effectiveness in business decision-making. Adopting best practices enhances usability and ensures that organizations can derive maximum value from predictive analytics. These practices can significantly reduce risks associated with implementation and improve the quality and efficiency of data insights.
Defining Clear Objectives
Before delving into predictive analytics, organizations must outline clear and measurable objectives. This step is essential to guide the entire implementation process. Clear objectives help to focus on what the organization aims to achieve, whether it is improving sales forecasts, enhancing customer satisfaction, or optimizing supply chain operations.
When setting these objectives, consider the following points:
- Specificity: Objectives should be well-defined. Rather than stating a goal like "improve sales", a measurable target could be "increase quarterly sales by 15% within the next fiscal year."
- Relevance: Ensure that the objectives align with the overall business strategy. This alignment guarantees that predictive analytics contributes to critical organizational goals.
- Measurable Outcomes: Establish metrics to evaluate success. These may include KPIs (Key Performance Indicators) relevant to predictive analytics outputs.
Defining these objectives creates a roadmap for the implementation process. It enables stakeholders to understand what success looks like and maintains focus throughout the project.
Continuous Monitoring and Improvement
After implementation, continuous monitoring of predictive analytics performance is vital. Organizations should analyze the outputs generated to identify trends, measure accuracy, and refine their models. This ongoing assessment ensures that the predictions remain reliable and relevant as business conditions change.
To facilitate effective monitoring, companies can consider the following actions:
- Regular Performance Reviews: Schedule periodic reviews to evaluate the performance of predictive analytics models. This includes assessing their accuracy and relevance over time.
- Feedback Loops: Establish feedback mechanisms from end-users. Users can provide invaluable insights into the practical effectiveness of the analytics and suggest areas for improvement.
- Model Updating: Predictive models should not be static. Organizations should revisit and adjust models based on new data and changing business priorities. This iterative approach helps in adapting to market volatility.
"Monitoring and improvement in predictive analytics is not a one-time event; it must be a continuous process to ensure optimal performance."
Implementing best practices such as defining clear objectives and committing to continuous monitoring vastly improves an organization’s ability to utilize SAP Analytics Cloud's predictive analytics effectively. By embracing these methodologies, businesses gain a strategic advantage, fostering data-driven decision-making to stay ahead in the competitive landscape.
Future Trends in Predictive Analytics
The landscape of predictive analytics is rapidly changing. Understanding future trends is essential for decision-makers and organizations looking to leverage SAP Analytics Cloud effectively. Businesses must not only grasp current capabilities, but also anticipate where predictive analytics is headed. This anticipation shapes strategies, enhances competitive advantage, and maximizes the impact of data-driven decision-making.
The Rise of Automated Analytics
Automated analytics is transforming the way organizations approach data. As the volume of data grows, manual analysis becomes impractical. Automated analytics simplifies this process by leveraging algorithms to analyze data rapidly, uncover insights, and produce reports with minimal human intervention. This shift allows teams to focus on interpreting results rather than just gathering and processing information.
Organizations utilizing SAP Analytics Cloud can harness automated analytics to enhance agility and responsiveness. For example, a retail business might use automated analytics to track sales data in real-time, allowing for timely adjustments to inventory. By minimizing the manual workload, businesses also reduce the risk of human error, further enhancing data reliability.
- Key Benefits of Automated Analytics:
- Improves speed and efficiency of data analysis
- Reduces human error
- Allows focus on strategic decision-making
- Enhances ability to identify trends early
"Automation in analytics not only streamlines processes but also empowers teams to sink their attention into more complex challenges."
Integration of Artificial Intelligence
The integration of artificial intelligence (AI) with predictive analytics is significant. AI enhances predictive modeling by incorporating machine learning techniques, enabling systems to learn from historical data and improve predictions over time. SAP Analytics Cloud, with its AI capabilities, allows users to build more sophisticated models that reflect complex relationships in the data.
This integration facilitates advanced scenario planning and forecasts, aiding organizations in navigating uncertainties. AI can also analyze large datasets beyond human capability, generating deeper insights and uncovering patterns that would typically remain hidden.
Consider a financial institution employing AI-driven predictive analytics to assess risk. It can evaluate a multitude of factors, enabling more accurate assessments of loan applications. The result is improved decision-making and reduced financial losses.
- Considerations for AI Integration:
- Ensure quality data for training models
- Continuously update models for accuracy
- Assess ethical implications of AI decisions
As predictive analytics continues to evolve, organizations must adapt. Keeping an eye on these trends will enable users to optimize their strategies and remain ahead in their respective markets.
Culmination
In the context of SAP Analytics Cloud, the conclusion encapsulates the critical insights gained throughout the article regarding predictive analytics. This section serves as a synthesis of the various elements discussed, emphasizing the undeniable significance of predictive insights in the modern business landscape.
The importance of adopting predictive analytics becomes evident when considering how it empowers organizations to make informed decisions. By leveraging data-driven strategies, businesses can forecast potential outcomes and trends, allowing for proactive adjustments in operations and strategy.
Key Benefits
Some of the notable benefits of employing predictive analytics include:
- Enhanced Decision-Making: Decision-makers can access foresight that guides their choices. The ability to predict market changes can lead to more strategic planning.
- Improved Efficiency: Organizations experience streamlined operations by anticipating customer behavior and optimizing resource allocation.
- Competitive Edge: Utilizing advanced analytics techniques, businesses can stay ahead of their competitors by identifying emerging trends before they become mainstream.
While the advantages are substantial, the conclusion also reinforces the need for cautious consideration regarding the challenges discussed. Factors such as data quality, technological integration, and skill gaps require systematic approaches to avoid hindering the implementation.
Moreover, adopting best practices in predictive analytics will further enhance effectiveness. Clear objectives and continuous monitoring foster an adaptive mindset, essential for success in a rapidly evolving market.
Effective predictive analytics is not merely a tool, but a strategic enabler for businesses aiming to thrive in complexity.